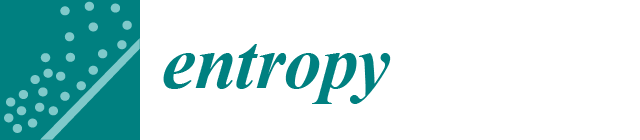
Journal Menu
► ▼ Journal Menu-
- Entropy Home
- Aims & Scope
- Editorial Board
- Reviewer Board
- Topical Advisory Panel
- Photography Exhibition
- Instructions for Authors
- Special Issues
- Topics
- Sections & Collections
- Article Processing Charge
- Indexing & Archiving
- Editor’s Choice Articles
- Most Cited & Viewed
- Journal Statistics
- Journal History
- Journal Awards
- Society Collaborations
- Conferences
- Editorial Office
Journal Browser
► ▼ Journal Browser-
arrow_forward_ios
Forthcoming issue
arrow_forward_ios Current issue - Vol. 26 (2024)
- Vol. 25 (2023)
- Vol. 24 (2022)
- Vol. 23 (2021)
- Vol. 22 (2020)
- Vol. 21 (2019)
- Vol. 20 (2018)
- Vol. 19 (2017)
- Vol. 18 (2016)
- Vol. 17 (2015)
- Vol. 16 (2014)
- Vol. 15 (2013)
- Vol. 14 (2012)
- Vol. 13 (2011)
- Vol. 12 (2010)
- Vol. 11 (2009)
- Vol. 10 (2008)
- Vol. 9 (2007)
- Vol. 8 (2006)
- Vol. 7 (2005)
- Vol. 6 (2004)
- Vol. 5 (2003)
- Vol. 4 (2002)
- Vol. 3 (2001)
- Vol. 2 (2000)
- Vol. 1 (1999)
Entropy, Volume 26, Issue 11 (November 2024) – 94 articles
- Issues are regarded as officially published after their release is announced to the table of contents alert mailing list.
- You may sign up for e-mail alerts to receive table of contents of newly released issues.
- PDF is the official format for papers published in both, html and pdf forms. To view the papers in pdf format, click on the "PDF Full-text" link, and use the free Adobe Reader to open them.
Previous Issue
Issue View Metrics
Multiple requests from the same IP address are counted as one view.