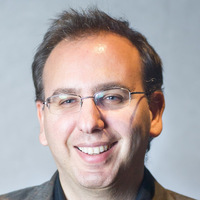
Nik Vasiloglou
Related Authors
Noel B. Salazar
KU Leuven
Geoffrey Skoll
SUNY: Buffalo State College
Monica Marquina
CONICET
Denilson Lopes
Universidade Federal do Rio de Janeiro (UFRJ)
Armando Marques-Guedes
UNL - New University of Lisbon
Tom Van Hout
University of Antwerp
Dr. Surendra Pathak
Dr. Hari Singh Gaur University, Sagar(M.P.)
Svend Hollensen
University of Southern Denmark
Emanuele Coccia
EHESS-Ecole des hautes études en sciences sociales
Dinesh Kumar
Amity University
Uploads
Papers by Nik Vasiloglou