-
Non-Intrusive Monitoring of Vital Signs in the Lower Limbs Using Optical Sensors
-
Structural Fatigue Monitoring with Piezoelectric Sensors: Fundamentals, Advances, and Future Directions
-
Monitoring Peatlands Using IoT
-
Flexible Electromagnetic Sensor with Inkjet-Printed Silver Nanoparticles on PET Substrate for Chemical and Biomedical Applications
Journal Description
Sensors
Sensors
is an international, peer-reviewed, open access journal on the science and technology of sensors. Sensors is published semimonthly online by MDPI. The Polish Society of Applied Electromagnetics (PTZE), Japan Society of Photogrammetry and Remote Sensing (JSPRS), Spanish Society of Biomedical Engineering (SEIB), International Society for the Measurement of Physical Behaviour (ISMPB) and Chinese Society of Micro-Nano Technology (CSMNT) are affiliated with Sensors and their members receive a discount on the article processing charges.
- Open Access — free for readers, with article processing charges (APC) paid by authors or their institutions.
- High Visibility: indexed within Scopus, SCIE (Web of Science), PubMed, MEDLINE, PMC, Ei Compendex, Inspec, Astrophysics Data System, and other databases.
- Journal Rank: JCR - Q2 (Chemistry, Analytical) / CiteScore - Q1 (Instrumentation)
- Rapid Publication: manuscripts are peer-reviewed and a first decision is provided to authors approximately 18.6 days after submission; acceptance to publication is undertaken in 2.4 days (median values for papers published in this journal in the second half of 2024).
- Recognition of Reviewers: reviewers who provide timely, thorough peer-review reports receive vouchers entitling them to a discount on the APC of their next publication in any MDPI journal, in appreciation of the work done.
- Testimonials: See what our editors and authors say about Sensors.
- Companion journals for Sensors include: Chips, JCP and Targets.
Impact Factor:
3.4 (2023);
5-Year Impact Factor:
3.7 (2023)
Latest Articles
A Review of Multi-Robot Systems and Soft Robotics: Challenges and Opportunities
Sensors 2025, 25(5), 1353; https://doi.org/10.3390/s25051353 (registering DOI) - 22 Feb 2025
Abstract
This review investigates the latest advancements in Multi-Robot Systems (MRSs) and soft robotics, with a particular focus on their integration and emerging opportunities. An MRS extends principles from distributed artificial intelligence and coordination frameworks, enabling efficient collaboration in robotic applications such as object
[...] Read more.
This review investigates the latest advancements in Multi-Robot Systems (MRSs) and soft robotics, with a particular focus on their integration and emerging opportunities. An MRS extends principles from distributed artificial intelligence and coordination frameworks, enabling efficient collaboration in robotic applications such as object manipulation, navigation, and transportation. Soft robotics employs flexible materials and biomimetic designs to improve adaptability in unstructured environments, with applications in manufacturing, sensing, actuation, and modeling. Unlike previous reviews, which often address these fields independently, this work emphasizes their integration, identifying key challenges such as nonlinear dynamics, hyper-redundant configurations, and adaptive control. This review discusses recent advancements in locomotion, coordination, and simulation, offering insights into the development of adaptive and collaborative robotic systems across diverse applications.
Full article
(This article belongs to the Special Issue Sensing for Automatic Control and Measurement System)
Open AccessArticle
A Compact Orthosis Compliance Monitoring Device Using Pressure Sensors and Accelerometers: Design and Proof-of-Concept Testing
by
Devi Baruni Devanand, Matthew D. Gardiner and Angela E. Kedgley
Sensors 2025, 25(5), 1352; https://doi.org/10.3390/s25051352 (registering DOI) - 22 Feb 2025
Abstract
Monitoring orthosis compliance using patient diaries is subjective, as patients can overestimate their levels of device use. An objective way to monitor compliance is required because if an orthotic prescription is not followed, the orthosis will not work as intended. This study aimed
[...] Read more.
Monitoring orthosis compliance using patient diaries is subjective, as patients can overestimate their levels of device use. An objective way to monitor compliance is required because if an orthotic prescription is not followed, the orthosis will not work as intended. This study aimed to develop and validate a device that monitors orthosis compliance objectively using pressure and acceleration. Fifteen participants were recruited to test the device’s ability to estimate wear time during the performance of several grip patterns and whilst completing selected activities of daily living. Sensor threshold values were used to discern whether users were wearing their orthosis or not. No differences between pressure sensor and accelerometer-based wear time estimations were found. The device’s pressure-based wear time estimations were found to have a specificity of 92.7 ± 16.4% and sensitivity of 74.0 ± 41.3%, whilst accelerometer-based wear time estimates had a specificity of 66.1 ± 34.7% and sensitivity of 86.2 ± 8.0%. This study successfully demonstrated the feasibility of monitoring hand orthosis compliance using pressure or acceleration. This device has the potential to provide insight into the effectiveness of both existing and novel orthotics, benefitting both clinical practice and research.
Full article
(This article belongs to the Special Issue Innovative Applications of Wearable Sensors in Musculoskeletal Biomechanics and Rehabilitation)
►▼
Show Figures
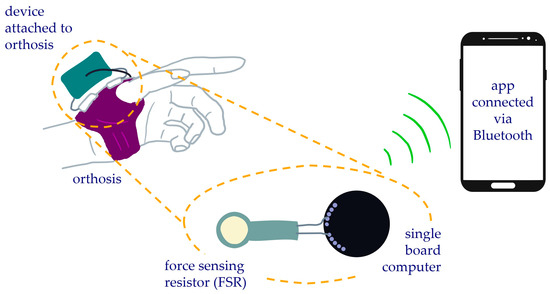
Figure 1
Figure 1
<p>Orthosis compliance monitoring device setup comprising of a force sensing resistor attached to a miniature single board computer with an in-built 3-axis accelerometer, connected to a mobile phone application via Bluetooth.</p> Full article ">Figure 2
<p>The force sensing resistor’s accuracy, calculated as the percentage agreement between actual and estimated wear times obtained while the orthosis was donned and doffed ten times, for various positions within a Push<sup>®</sup> orthosis.</p> Full article ">Figure 3
<p>The orthosis compliance monitoring device after electronics were compacted.</p> Full article ">Figure 4
<p>The final prototype of the orthosis compliance monitoring device fitted in an off-the-shelf enclosure. (<b>a</b>) Electronic components in enclosure. (<b>b</b>) Device enclosed. (<b>c</b>) Device attached to the straps of the orthosis. (<b>d</b>) Orthosis instrumented with compliance monitoring device worn by user.</p> Full article ">Figure 5
<p>The first part of the Southampton Hand Assessment Procedure (SHAP). Participants were required to lift six abstract objects from one slot to another using a different grip pattern for each. This was completed using both light and heavy abstract objects.</p> Full article ">Figure 6
<p>The second part of the Southampton Hand Assessment Procedure (SHAP). Participants were asked to perform 14 different activities of daily living (ADLs) whilst wearing the orthosis instrumented with the compliance monitoring device.</p> Full article ">Figure 7
<p>The equations used to calculate device accuracy as sensitivity, specificity, positive predictive value, and negative predictive value.</p> Full article ">Figure 8
<p>Average accuracy of wear time estimations, by the force sensing resistor (FSR) and accelerometer, whilst participants completed activities of daily living (ADLs) as part of the Southampton Hand Assessment Procedure (SHAP).</p> Full article ">Figure 9
<p>User feedback obtained regarding the issues they faced and thus, areas of improvement for the device. Feedback segment size is proportional to the frequency of said feedback received.</p> Full article ">
<p>Orthosis compliance monitoring device setup comprising of a force sensing resistor attached to a miniature single board computer with an in-built 3-axis accelerometer, connected to a mobile phone application via Bluetooth.</p> Full article ">Figure 2
<p>The force sensing resistor’s accuracy, calculated as the percentage agreement between actual and estimated wear times obtained while the orthosis was donned and doffed ten times, for various positions within a Push<sup>®</sup> orthosis.</p> Full article ">Figure 3
<p>The orthosis compliance monitoring device after electronics were compacted.</p> Full article ">Figure 4
<p>The final prototype of the orthosis compliance monitoring device fitted in an off-the-shelf enclosure. (<b>a</b>) Electronic components in enclosure. (<b>b</b>) Device enclosed. (<b>c</b>) Device attached to the straps of the orthosis. (<b>d</b>) Orthosis instrumented with compliance monitoring device worn by user.</p> Full article ">Figure 5
<p>The first part of the Southampton Hand Assessment Procedure (SHAP). Participants were required to lift six abstract objects from one slot to another using a different grip pattern for each. This was completed using both light and heavy abstract objects.</p> Full article ">Figure 6
<p>The second part of the Southampton Hand Assessment Procedure (SHAP). Participants were asked to perform 14 different activities of daily living (ADLs) whilst wearing the orthosis instrumented with the compliance monitoring device.</p> Full article ">Figure 7
<p>The equations used to calculate device accuracy as sensitivity, specificity, positive predictive value, and negative predictive value.</p> Full article ">Figure 8
<p>Average accuracy of wear time estimations, by the force sensing resistor (FSR) and accelerometer, whilst participants completed activities of daily living (ADLs) as part of the Southampton Hand Assessment Procedure (SHAP).</p> Full article ">Figure 9
<p>User feedback obtained regarding the issues they faced and thus, areas of improvement for the device. Feedback segment size is proportional to the frequency of said feedback received.</p> Full article ">
Open AccessArticle
Posture Control of Hydraulic Flexible Second-Order Manipulators Based on Adaptive Integral Terminal Variable-Structure Predictive Method
by
Jianliang Xu, Zhen Sui and Feng Xu
Sensors 2025, 25(5), 1351; https://doi.org/10.3390/s25051351 (registering DOI) - 22 Feb 2025
Abstract
As operational scenarios become more complex and task demands intensify, the requirements for the intelligence and automation of manipulators in industry are increasing. This work investigates the challenge of posture tracking control for hydraulic flexible manipulators by proposing a discrete-time integral terminal sliding
[...] Read more.
As operational scenarios become more complex and task demands intensify, the requirements for the intelligence and automation of manipulators in industry are increasing. This work investigates the challenge of posture tracking control for hydraulic flexible manipulators by proposing a discrete-time integral terminal sliding mode predictive control (DITSMPC) method. First, the proposed method develops a second-order dynamic model of the manipulator using the Lagrangian dynamic strategy. Second, a discrete-time sliding mode control (SMC) law based on an adaptive switching term is designed to achieve high-precision tracking control of the system. Finally, to weaken the influence of SMC buffeting on the manipulator system, the predictive time domain function is integrated into the proposed SMC law, and the delay estimation of the unknown term in the manipulator system is carried out. The DITSMPC scheme is derived and its convergence is proven. Simulation experiments comparing the DITSMPC scheme with the classical discrete-time SMC method demonstrate that the proposed scheme results in smooth torque changes in each joint of the manipulator, with the integral of torque variations being . The trajectory tracking errors for each joint remain within ±0.0025 rad, all of which are smaller than those of the classical scheme.
Full article
(This article belongs to the Special Issue Dynamics and Control System Design for Robot Manipulation)
Open AccessArticle
Content-Based Histopathological Image Retrieval
by
Camilo Nuñez-Fernández , Humberto Farias and Mauricio Solar
Sensors 2025, 25(5), 1350; https://doi.org/10.3390/s25051350 (registering DOI) - 22 Feb 2025
Abstract
Feature descriptors in histopathological images are an important challenge for the implementation of Content-Based Image Retrieval (CBIR) systems, an essential tool to support pathologists. Deep learning models like Convolutional Neural Networks and Vision Transformers improve the extraction of these feature descriptors. These models
[...] Read more.
Feature descriptors in histopathological images are an important challenge for the implementation of Content-Based Image Retrieval (CBIR) systems, an essential tool to support pathologists. Deep learning models like Convolutional Neural Networks and Vision Transformers improve the extraction of these feature descriptors. These models typically generate embeddings by leveraging deeper single-scale linear layers or advanced pooling layers. However, these embeddings, by focusing on local spatial details at a single scale, miss out on the richer spatial context from earlier layers. This gap suggests the development of methods that incorporate multi-scale information to enhance the depth and utility of feature descriptors in histopathological image analysis. In this work, we propose the Local–Global Feature Fusion Embedding Model. This proposal is composed of three elements: (1) a pre-trained backbone for feature extraction from multi-scales, (2) a neck branch for local–global feature fusion, and (3) a Generalized Mean (GeM)-based pooling head for feature descriptors. Based on our experiments, the model’s neck and head were trained on ImageNet-1k and PanNuke datasets employing the Sub-center ArcFace loss and compared with the state-of-the-art Kimia Path24C dataset for histopathological image retrieval, achieving a Recall@1 of 99.40% for test patches.
Full article
(This article belongs to the Special Issue Deep Learning-Based Image and Signal Sensing and Processing: 2nd Edition)
Open AccessArticle
An Independent UAV-Based Mobile Base Station
by
Sung-Chan Choi and Sung-Yeon Kim
Sensors 2025, 25(5), 1349; https://doi.org/10.3390/s25051349 (registering DOI) - 22 Feb 2025
Abstract
In disaster scenarios, e.g., earthquakes, tsunamis, and wildfires, communication infrastructure often becomes severely damaged. To rapidly restore damaged communication systems, we propose a UAV-based mobile base station equipped with Public Safety LTE (PS-LTE) technology to provide standalone communication capabilities. The proposed system includes
[...] Read more.
In disaster scenarios, e.g., earthquakes, tsunamis, and wildfires, communication infrastructure often becomes severely damaged. To rapidly restore damaged communication systems, we propose a UAV-based mobile base station equipped with Public Safety LTE (PS-LTE) technology to provide standalone communication capabilities. The proposed system includes PS-LTE functionalities, mission-critical push-to-talk, proximity-based services, and isolated E-UTRAN operation to ensure the reliable and secure communication for emergency services. We provide a simulation result to achieve the radio coverage of mobile base station. By using this radio coverage, we find an appropriate location of the end device for performing the outdoor experiments. We develop a prototype of a proposed mobile base station and test its operation in an outdoor environment. The experimental results provide a sufficient data rate to make an independent mobile base station to restore communication infrastructure in areas that experienced environmental disasters. This prototype and experimental results offer a significant step forward in creating agile and efficient communication solutions for emergency scenarios.
Full article
(This article belongs to the Topic Unmanned Vehicles Technology and Embodied Intelligence Systems for Intelligent Transportation)
Open AccessArticle
Preparation of Ion Composite Photosensitive Resin and Its Application in 3D-Printing Highly Sensitive Pressure Sensor
by
Tong Guan, Huayang Li, Jinyun Liu, Wuxu Zhang, Siying Wang, Wentao Ye, Baoru Bian, Xiaohui Yi, Yuanzhao Wu, Yiwei Liu, Juan Du, Jie Shang and Run-Wei Li
Sensors 2025, 25(5), 1348; https://doi.org/10.3390/s25051348 (registering DOI) - 22 Feb 2025
Abstract
Flexible pressure sensors play an extremely important role in the fields of intelligent medical treatment, humanoid robots, and so on. However, the low sensitivity and the small initial capacitance still limit its application and development. At present, the method of constructing the microstructure
[...] Read more.
Flexible pressure sensors play an extremely important role in the fields of intelligent medical treatment, humanoid robots, and so on. However, the low sensitivity and the small initial capacitance still limit its application and development. At present, the method of constructing the microstructure of the dielectric layer is commonly used to improve the sensitivity of the sensor, but there are some problems, such as the complex process and inaccurate control of the microstructure. In this work, an ion composite photosensitive resin based on polyurethane acrylate and ionic liquids (ILs) was prepared. The high compatibility of the photosensitive resin and ILs was achieved by adding a chitooligosaccharide (COS) chain extender. The microstructure of the dielectric layer was optimized by digital light processing (DLP) 3D-printing. Due to the introduction of ILs to construct an electric double layer (EDL), the flexible pressure sensor exhibits a high sensitivity of 32.62 kPa−1, which is 12.2 times higher than that without ILs. It also has a wide range of 100 kPa and a fast response time of 51 ms. It has a good pressure response under different pressures and can realize the demonstration application of human health.
Full article
(This article belongs to the Special Issue Wearable Sensors for Continuous Health Monitoring and Analysis)
►▼
Show Figures
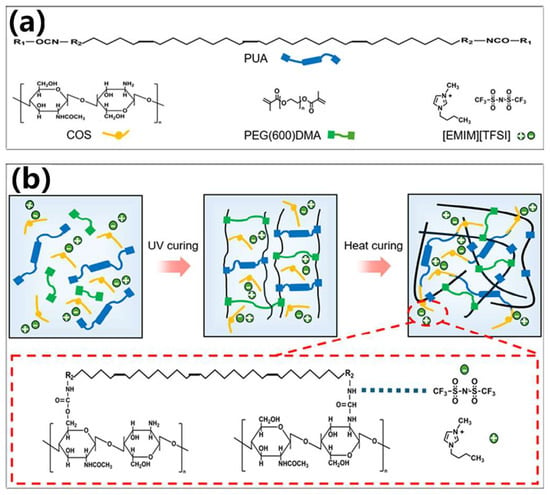
Figure 1
Figure 1
<p>Curing principle of ion composite photosensitive resin: (<b>a</b>) chemical structure of PUA, COS, PEG(600)DMA, and [EMIM][TFSI]; and (<b>b</b>) the schematic diagram of UV curing and heat curing.</p> Full article ">Figure 2
<p>(<b>a</b>) Preparation process of dielectric layer; (<b>b</b>,<b>c</b>) SEM and EDX on the surface of ion composite photosensitive resin without COS; (<b>d</b>,<b>e</b>) the cross-section SEM and EDX of ion composite photosensitive resin without COS; (<b>f</b>,<b>g</b>) SEM and EDX on the surface of ion composite photosensitive resin with COS; (<b>h</b>,<b>i</b>) the cross section SEM and EDX of ion composite photosensitive resin with COS; and (<b>j</b>) image of different structures by DLP 3D printing.</p> Full article ">Figure 3
<p>Mechanical and electrical properties of ion composite photosensitive resin: (<b>a</b>–<b>c</b>) the mechanical properties changed with the content of COS, PEG(600)DMA, and ILs; (<b>d</b>) the comparison of the tensile loading–unloading curves of the ion composite photosensitive resin containing 40 wt.% ILs (PUA@ILs) and other commercial flexible photosensitive resins; and (<b>e</b>,<b>f</b>) distribution of sample conductivity before and after compression.</p> Full article ">Figure 4
<p>3D printing of ion composite photosensitive resin: (<b>a</b>) relationship between viscosity of photosensitive resin and content of [EMIM][TFSI]; (<b>b</b>) the relationship between curing depth and exposure energy; (<b>c</b>) horizontal resolution of ion composite photosensitive resin; and (<b>d</b>) image of TYPE-C structure printed by ion composite photosensitive resin.</p> Full article ">Figure 5
<p>Sensing performance of the 3D-printed capacitive sensor based on ion composite photosensitive resin: (<b>a</b>) sensor structure diagram; (<b>b</b>) the ΔC/C<sub>0</sub>–P relation of sensors with dielectric layers of different lattice structures; (<b>c</b>) the ΔC/C<sub>0</sub>–P relation of sensors with TYPE-C dielectric layers of different density ratios; (<b>d</b>) the ΔC/C<sub>0</sub>–P relation of TYPE-C structure with 25% density ratio; (<b>e</b>) the sensitivity of sensors with different dielectric layer materials; and (<b>f</b>) comparison of sensitivity and range (sensitivity > 0.1 kPa<sup>−1</sup>) of different capacitive sensors based on photosensitive resin [<a href="#B32-sensors-25-01348" class="html-bibr">32</a>,<a href="#B37-sensors-25-01348" class="html-bibr">37</a>,<a href="#B38-sensors-25-01348" class="html-bibr">38</a>,<a href="#B39-sensors-25-01348" class="html-bibr">39</a>,<a href="#B47-sensors-25-01348" class="html-bibr">47</a>,<a href="#B48-sensors-25-01348" class="html-bibr">48</a>].</p> Full article ">Figure 6
<p>Other sensing performance and application demonstrations of the 3D-printed capacitive sensor based on ion composite photosensitive resin: (<b>a</b>) response time of the sensor at approximately 3 kPa; (<b>b</b>) response of the sensor to a gradually increasing force of 20 kPa; (<b>c</b>) the cyclic response of the sensor under different pressures (1 kPa, 10 kPa, 20 kPa, 50 kPa, and 100 kPa); (<b>d</b>) response of the sensor under 1000 cycles; (<b>e</b>) real-time monitoring of human pulse; and (<b>f</b>) real-time monitoring of deep breath, swallow, and cough in the human throat.</p> Full article ">
<p>Curing principle of ion composite photosensitive resin: (<b>a</b>) chemical structure of PUA, COS, PEG(600)DMA, and [EMIM][TFSI]; and (<b>b</b>) the schematic diagram of UV curing and heat curing.</p> Full article ">Figure 2
<p>(<b>a</b>) Preparation process of dielectric layer; (<b>b</b>,<b>c</b>) SEM and EDX on the surface of ion composite photosensitive resin without COS; (<b>d</b>,<b>e</b>) the cross-section SEM and EDX of ion composite photosensitive resin without COS; (<b>f</b>,<b>g</b>) SEM and EDX on the surface of ion composite photosensitive resin with COS; (<b>h</b>,<b>i</b>) the cross section SEM and EDX of ion composite photosensitive resin with COS; and (<b>j</b>) image of different structures by DLP 3D printing.</p> Full article ">Figure 3
<p>Mechanical and electrical properties of ion composite photosensitive resin: (<b>a</b>–<b>c</b>) the mechanical properties changed with the content of COS, PEG(600)DMA, and ILs; (<b>d</b>) the comparison of the tensile loading–unloading curves of the ion composite photosensitive resin containing 40 wt.% ILs (PUA@ILs) and other commercial flexible photosensitive resins; and (<b>e</b>,<b>f</b>) distribution of sample conductivity before and after compression.</p> Full article ">Figure 4
<p>3D printing of ion composite photosensitive resin: (<b>a</b>) relationship between viscosity of photosensitive resin and content of [EMIM][TFSI]; (<b>b</b>) the relationship between curing depth and exposure energy; (<b>c</b>) horizontal resolution of ion composite photosensitive resin; and (<b>d</b>) image of TYPE-C structure printed by ion composite photosensitive resin.</p> Full article ">Figure 5
<p>Sensing performance of the 3D-printed capacitive sensor based on ion composite photosensitive resin: (<b>a</b>) sensor structure diagram; (<b>b</b>) the ΔC/C<sub>0</sub>–P relation of sensors with dielectric layers of different lattice structures; (<b>c</b>) the ΔC/C<sub>0</sub>–P relation of sensors with TYPE-C dielectric layers of different density ratios; (<b>d</b>) the ΔC/C<sub>0</sub>–P relation of TYPE-C structure with 25% density ratio; (<b>e</b>) the sensitivity of sensors with different dielectric layer materials; and (<b>f</b>) comparison of sensitivity and range (sensitivity > 0.1 kPa<sup>−1</sup>) of different capacitive sensors based on photosensitive resin [<a href="#B32-sensors-25-01348" class="html-bibr">32</a>,<a href="#B37-sensors-25-01348" class="html-bibr">37</a>,<a href="#B38-sensors-25-01348" class="html-bibr">38</a>,<a href="#B39-sensors-25-01348" class="html-bibr">39</a>,<a href="#B47-sensors-25-01348" class="html-bibr">47</a>,<a href="#B48-sensors-25-01348" class="html-bibr">48</a>].</p> Full article ">Figure 6
<p>Other sensing performance and application demonstrations of the 3D-printed capacitive sensor based on ion composite photosensitive resin: (<b>a</b>) response time of the sensor at approximately 3 kPa; (<b>b</b>) response of the sensor to a gradually increasing force of 20 kPa; (<b>c</b>) the cyclic response of the sensor under different pressures (1 kPa, 10 kPa, 20 kPa, 50 kPa, and 100 kPa); (<b>d</b>) response of the sensor under 1000 cycles; (<b>e</b>) real-time monitoring of human pulse; and (<b>f</b>) real-time monitoring of deep breath, swallow, and cough in the human throat.</p> Full article ">
Open AccessArticle
AIPI: Network Status Identification on Multi-Protocol Wireless Sensor Networks
by
Peng Jiang, Xinglin Feng, Renhai Feng and Junpeng Cui
Sensors 2025, 25(5), 1347; https://doi.org/10.3390/s25051347 (registering DOI) - 22 Feb 2025
Abstract
Topology control is important for extending networks lifetime and reducing interference. The accuracy of topology identification plays a crucial role in topology control. Traditional passive interception can only identify the connectivity among cooperative sensor networks with known protocol. This paper proposes a novel
[...] Read more.
Topology control is important for extending networks lifetime and reducing interference. The accuracy of topology identification plays a crucial role in topology control. Traditional passive interception can only identify the connectivity among cooperative sensor networks with known protocol. This paper proposes a novel method called Active Interfere and Passive Interception (AIPI) to identify the topology of non-cooperative sensor networks by using both active and passive interceptions. Active interception uses full duplex sensors to disrupt communication until frequency hopped to acquire distance information, and thus, infer their connectivity and calculate the location after modifying error in a non-cooperative sensor network. Passive interception uses Granger causality to infer the connectivity between two communication nodes after getting the time frame structure in physical layer. Passive interception is applied to conserve power consumption after obtaining physical information via active interception. Simulation results indicate that AIPI can identify the topology of non-cooperative sensor networks with a higher accuracy than traditional method.
Full article
(This article belongs to the Special Issue Security Issues and Solutions in Sensing Systems and Networks)
Open AccessArticle
Securing IoT Networks Against DDoS Attacks: A Hybrid Deep Learning Approach
by
Noor Ul Ain, Muhammad Sardaraz, Muhammad Tahir, Mohamed W. Abo Elsoud and Abdullah Alourani
Sensors 2025, 25(5), 1346; https://doi.org/10.3390/s25051346 (registering DOI) - 22 Feb 2025
Abstract
The Internet of Things (IoT) has revolutionized many domains. Due to the growing interconnectivity of IoT networks, several security challenges persist that need to be addressed. This research presents the application of deep learning techniques for Distributed Denial-of-Service (DDoS) attack detection in IoT
[...] Read more.
The Internet of Things (IoT) has revolutionized many domains. Due to the growing interconnectivity of IoT networks, several security challenges persist that need to be addressed. This research presents the application of deep learning techniques for Distributed Denial-of-Service (DDoS) attack detection in IoT networks. This study assesses the performance of various deep learning models, including Latent Autoencoders, LSTM Autoencoders, and convolutional neural networks (CNNs), for DDoS attack detection in IoT environments. Furthermore, a novel hybrid model is proposed, integrating CNNs for feature extraction, Long Short-Term Memory (LSTM) networks for temporal pattern recognition, and Autoencoders for dimensionality reduction. Experimental results on the CICIOT2023 dataset show the enhanced performance of the proposed hybrid model, achieving training and testing accuracy of 96.78% integrated with 96.60% validation accuracy. This presents its efficiency in addressing complex attack patterns within IoT networks. Results’ analysis shows that the proposed hybrid model outperforms the others. However, this research has limitations in detecting rare attack types and emphasizes the importance of addressing data imbalance challenges for further enhancement of DDoS attack detection capabilities in future.
Full article
(This article belongs to the Special Issue Machine Learning and Big Data Analytics for the Internet of Things and Wireless Sensor Networks)
►▼
Show Figures
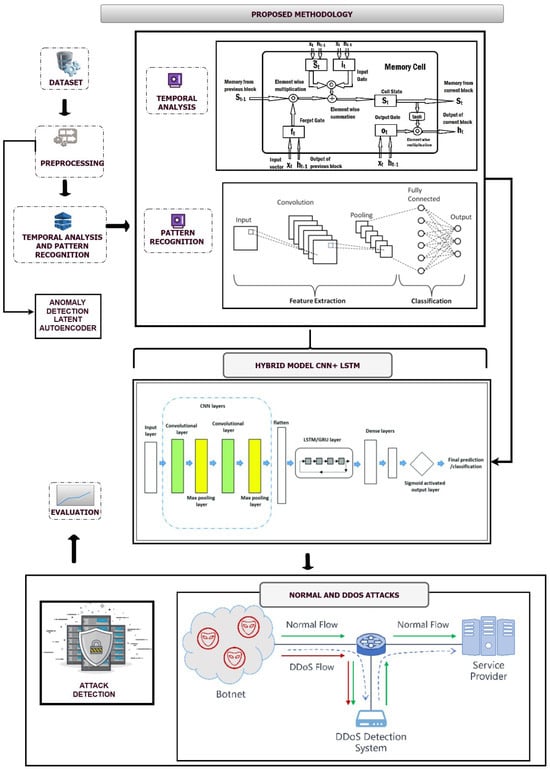
Figure 1
Figure 1
<p>Workflow of the proposed model.</p> Full article ">Figure 2
<p>Validation and training loss of Autoencoder.</p> Full article ">Figure 3
<p>Distribution of reconstruction errors.</p> Full article ">Figure 4
<p>A histogram illustrating the distribution of reconstruction errors.</p> Full article ">Figure 5
<p>Training and validation loss of the LSTM model.</p> Full article ">Figure 6
<p>Anomaly detection in test data for LSTM-based Autoencoder.</p> Full article ">Figure 7
<p>Distribution of reconstruction error on test data.</p> Full article ">Figure 8
<p>Performance of the CNN model during training and validation phases.</p> Full article ">Figure 9
<p>Confusion matrix of the CNN.</p> Full article ">Figure 10
<p>Performance of the hybrid model during training and validation phases.</p> Full article ">Figure 11
<p>Confusion matrix of the hybrid model.</p> Full article ">
<p>Workflow of the proposed model.</p> Full article ">Figure 2
<p>Validation and training loss of Autoencoder.</p> Full article ">Figure 3
<p>Distribution of reconstruction errors.</p> Full article ">Figure 4
<p>A histogram illustrating the distribution of reconstruction errors.</p> Full article ">Figure 5
<p>Training and validation loss of the LSTM model.</p> Full article ">Figure 6
<p>Anomaly detection in test data for LSTM-based Autoencoder.</p> Full article ">Figure 7
<p>Distribution of reconstruction error on test data.</p> Full article ">Figure 8
<p>Performance of the CNN model during training and validation phases.</p> Full article ">Figure 9
<p>Confusion matrix of the CNN.</p> Full article ">Figure 10
<p>Performance of the hybrid model during training and validation phases.</p> Full article ">Figure 11
<p>Confusion matrix of the hybrid model.</p> Full article ">
Open AccessArticle
A Method Combining Discrete Cosine Transform with Attention for Multi-Temporal Remote Sensing Image Matching
by
Qinyan Zeng, Bin Hui, Zhaoji Liu, Zheng Xu and Miao He
Sensors 2025, 25(5), 1345; https://doi.org/10.3390/s25051345 (registering DOI) - 22 Feb 2025
Abstract
Multi-temporal remote sensing image matching is crucial for tasks such as drone positioning under satellite-denial conditions, natural disaster monitoring, and land-cover-change detection. However, the significant differences between multi-temporal images often lead to the reduced accuracy or even failure of most image matching methods
[...] Read more.
Multi-temporal remote sensing image matching is crucial for tasks such as drone positioning under satellite-denial conditions, natural disaster monitoring, and land-cover-change detection. However, the significant differences between multi-temporal images often lead to the reduced accuracy or even failure of most image matching methods in these scenarios. To address this challenge, this paper introduces a Discrete Cosine Transform (DCT) for frequency analysis tailored to the characteristics of remote sensing images, and proposes a network that combines the DCT with attention mechanisms for multi-scale feature matching. First, DCT-enhanced channel attention is embedded in the multi-scale feature extraction module to capture richer ground object information. Second, in coarse-scale feature matching, DCT-guided sparse attention is proposed for feature enhancement, which suppresses the impact of temporal differences on matching while making the amount of computation controllable. The coarse-scale matching results are further refined in the fine-scale feature map to obtain the final matches. Our method achieved correct keypoint percentages of 81.92% and 88.48%, with average corner errors of 4.27 and 2.98 pixels on the DSIFN dataset and LEVIR-CD dataset, respectively, while maintaining a high inference speed. The experimental results demonstrate that our method outperformed the state-of-art methods in terms of both robustness and efficiency in the multi-temporal scenarios.
Full article
(This article belongs to the Section Remote Sensors)
►▼
Show Figures
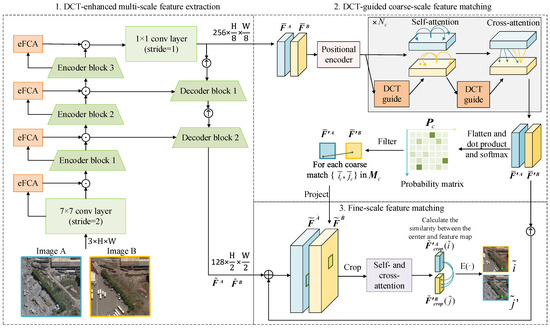
Figure 1
Figure 1
<p>Overall framework of the proposed method.</p> Full article ">Figure 2
<p>The details in the feature extraction module. (<b>a</b>) The structure of the blocks. BN is short for Batch Normalization. (<b>b</b>) The structure of the enhanced frequency channel attention (eFCA).</p> Full article ">Figure 3
<p>The flowchart of DCT-guided sparse attention. For self-attention, <math display="inline"><semantics> <mi mathvariant="bold-italic">Q</mi> </semantics></math>, <math display="inline"><semantics> <mi mathvariant="bold-italic">K</mi> </semantics></math>, and <math display="inline"><semantics> <mi mathvariant="bold-italic">V</mi> </semantics></math> all come from the feature map to be updated. For cross-attention, <math display="inline"><semantics> <mi mathvariant="bold-italic">Q</mi> </semantics></math> comes from the feature map to be updated, while <math display="inline"><semantics> <mi mathvariant="bold-italic">K</mi> </semantics></math> and <math display="inline"><semantics> <mi mathvariant="bold-italic">V</mi> </semantics></math> come from the other one.</p> Full article ">Figure 4
<p>Examples of the datasets [<a href="#B51-sensors-25-01345" class="html-bibr">51</a>,<a href="#B52-sensors-25-01345" class="html-bibr">52</a>,<a href="#B53-sensors-25-01345" class="html-bibr">53</a>].</p> Full article ">Figure 5
<p>Box plots of ablation experiment results. The dots represent outliers of the results, and the orange lines indicate the median. (<b>a</b>) Box plot of PCK results. (<b>b</b>) Box plot of ACE results.</p> Full article ">Figure 6
<p>The line charts show the proportion of results that met different PCK thresholds. The x-axis represents the set PCK thresholds, and the y-axis represents the proportion of images in the dataset that satisfied each threshold. (<b>a</b>) Results on the DSIFN dataset. (<b>b</b>) Results on the LEVIR-CD dataset.</p> Full article ">Figure 7
<p>Visualization of some results from the DSIFN dataset and the LEVIR-CD dataset. The two groups on the left were from the DSIFN dataset, while the two groups on the right were from the LEVIR-CD dataset. The green dots indicate correctly matched keypoints, and the red dots indicate incorrectly matched keypoints.</p> Full article ">Figure 8
<p>This is a visualization of qualitative comparison, with correct matching keypoints represented in green and incorrect matching keypoints represented in red.</p> Full article ">
<p>Overall framework of the proposed method.</p> Full article ">Figure 2
<p>The details in the feature extraction module. (<b>a</b>) The structure of the blocks. BN is short for Batch Normalization. (<b>b</b>) The structure of the enhanced frequency channel attention (eFCA).</p> Full article ">Figure 3
<p>The flowchart of DCT-guided sparse attention. For self-attention, <math display="inline"><semantics> <mi mathvariant="bold-italic">Q</mi> </semantics></math>, <math display="inline"><semantics> <mi mathvariant="bold-italic">K</mi> </semantics></math>, and <math display="inline"><semantics> <mi mathvariant="bold-italic">V</mi> </semantics></math> all come from the feature map to be updated. For cross-attention, <math display="inline"><semantics> <mi mathvariant="bold-italic">Q</mi> </semantics></math> comes from the feature map to be updated, while <math display="inline"><semantics> <mi mathvariant="bold-italic">K</mi> </semantics></math> and <math display="inline"><semantics> <mi mathvariant="bold-italic">V</mi> </semantics></math> come from the other one.</p> Full article ">Figure 4
<p>Examples of the datasets [<a href="#B51-sensors-25-01345" class="html-bibr">51</a>,<a href="#B52-sensors-25-01345" class="html-bibr">52</a>,<a href="#B53-sensors-25-01345" class="html-bibr">53</a>].</p> Full article ">Figure 5
<p>Box plots of ablation experiment results. The dots represent outliers of the results, and the orange lines indicate the median. (<b>a</b>) Box plot of PCK results. (<b>b</b>) Box plot of ACE results.</p> Full article ">Figure 6
<p>The line charts show the proportion of results that met different PCK thresholds. The x-axis represents the set PCK thresholds, and the y-axis represents the proportion of images in the dataset that satisfied each threshold. (<b>a</b>) Results on the DSIFN dataset. (<b>b</b>) Results on the LEVIR-CD dataset.</p> Full article ">Figure 7
<p>Visualization of some results from the DSIFN dataset and the LEVIR-CD dataset. The two groups on the left were from the DSIFN dataset, while the two groups on the right were from the LEVIR-CD dataset. The green dots indicate correctly matched keypoints, and the red dots indicate incorrectly matched keypoints.</p> Full article ">Figure 8
<p>This is a visualization of qualitative comparison, with correct matching keypoints represented in green and incorrect matching keypoints represented in red.</p> Full article ">
Open AccessArticle
Assessment of the Mechanical Properties of Soft Tissue Phantoms Using Impact Analysis
by
Arthur Bouffandeau, Anne-Sophie Poudrel, Chloé Brossier, Giuseppe Rosi, Vu-Hieu Nguyen, Charles-Henri Flouzat-Lachaniette, Jean-Paul Meningaud and Guillaume Haïat
Sensors 2025, 25(5), 1344; https://doi.org/10.3390/s25051344 (registering DOI) - 22 Feb 2025
Abstract
Skin physiopathological conditions have a strong influence on its biomechanical properties. However, it remains difficult to accurately assess the surface stiffness of soft tissues. The aim of this study was to evaluate the performances of an impact-based analysis method (IBAM) and to compare
[...] Read more.
Skin physiopathological conditions have a strong influence on its biomechanical properties. However, it remains difficult to accurately assess the surface stiffness of soft tissues. The aim of this study was to evaluate the performances of an impact-based analysis method (IBAM) and to compare them with those of an existing digital palpation device, MyotonPro®. The IBAM is based on the impact of an instrumented hammer equipped with a force sensor on a cylindrical punch in contact with agar-based phantoms mimicking soft tissues. The indicator Δt is estimated by analyzing the force signal obtained from the instrumented hammer. Various phantom geometries, stiffnesses and structures (homogeneous and bilayer) were used to estimate the performances of both methods. Measurements show that the IBAM is sensitive to a volume of interest equivalent to a sphere approximately twice the punch diameter. The sensitivity of the IBAM to changes in Young’s modulus is similar to that of dynamic mechanical analysis (DMA) and significantly better compared to MyotonPro. The axial (respectively, lateral) resolution is two (respectively, five) times lower with the IBAM than with MyotonPro. The present study paves the way for the development of a simple, quantitative and non-invasive method to measure skin biomechanical properties.
Full article
(This article belongs to the Special Issue (Bio)sensors for Physiological Monitoring)
Open AccessArticle
The Design of a Low-Power Pipelined ADC for IoT Applications
by
Junkai Zhang, Tao Sun, Zunkai Huang, Wei Tao, Ning Wang, Li Tian, Yongxin Zhu and Hui Wang
Sensors 2025, 25(5), 1343; https://doi.org/10.3390/s25051343 (registering DOI) - 22 Feb 2025
Abstract
This paper proposes a low-power 10-bit 20 MS/s pipelined analog-to-digital converter (ADC) designed for the burgeoning needs of low-data-rate communication systems, particularly within the Internet of Things (IoT) domain. To reduce power usage, multiple power-saving techniques are combined, such as sample-and-hold amplifier-less (SHA-less)
[...] Read more.
This paper proposes a low-power 10-bit 20 MS/s pipelined analog-to-digital converter (ADC) designed for the burgeoning needs of low-data-rate communication systems, particularly within the Internet of Things (IoT) domain. To reduce power usage, multiple power-saving techniques are combined, such as sample-and-hold amplifier-less (SHA-less) architecture, capacitor scaling, and dynamic comparators. In addition, this paper presents a novel operational amplifier (op-amp) with gain boosting, featuring a dual-input differential pair that enables internal pipeline stage switching, effectively alleviating the crosstalk and memory effects inherent in conventional shared op-amp configurations, thereby further reducing power consumption. A prototype ADC was fabricated in a 180 nm CMOS process and the core size was 0.333 mm2. The ADC implemented operated at a 20 MHz sampling rate under a 1.8 V supply voltage. It achieved a spurious-free dynamic range (SFDR) of 61.83 dB and a signal-to-noise-and-distortion ratio (SNDR) of 54.15 dB while demonstrating a maximum differential non-linearity (DNL) of 0.36 least significant bit (LSB) and a maximum integral non-linearity (INL) of 0.67 LSB. Notably, the ADC consumed less than 5 mW of power at the mentioned sampling frequency, showcasing excellent power efficiency.
Full article
(This article belongs to the Section Electronic Sensors)
►▼
Show Figures
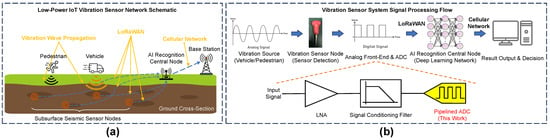
Figure 1
Figure 1
<p>An overview of a low-power IoT vibration sensor network. (<b>a</b>) Subsurface seismic sensor deployment for vehicle/pedestrian vibration detection in a smart city, with LoRaWAN communication to an AI node. (<b>b</b>) Signal processing flow in a sensor node, highlighting the analog front-end and proposed low-power pipelined ADC for energy-efficient data conversion.</p> Full article ">Figure 2
<p>Architecture of 10-bit pipelined ADC.</p> Full article ">Figure 3
<p>Structure and timing of first stage and second stage.</p> Full article ">Figure 4
<p>Structure of gate-boosted switch.</p> Full article ">Figure 5
<p>Dual-input, differential-pair, gain-boosted, switched operational amplifier circuit.</p> Full article ">Figure 6
<p>Switched-capacitor common-mode feedback circuit.</p> Full article ">Figure 7
<p>(<b>a</b>) The dynamic comparator with a pre-amplifier and (<b>b</b>) differential-pair dynamic comparator.</p> Full article ">Figure 8
<p>Die photo micrograph.</p> Full article ">Figure 9
<p>Measured ADC output FFT spectra under (<b>a</b>) <math display="inline"><semantics> <msub> <mi>F</mi> <mi>S</mi> </msub> </semantics></math> = 20 MHz and <math display="inline"><semantics> <msub> <mi>F</mi> <mrow> <mi>I</mi> <mi>N</mi> </mrow> </msub> </semantics></math> = 1MHz; (<b>b</b>) <math display="inline"><semantics> <msub> <mi>F</mi> <mi>S</mi> </msub> </semantics></math> = 20 MHz and <math display="inline"><semantics> <msub> <mi>F</mi> <mrow> <mi>I</mi> <mi>N</mi> </mrow> </msub> </semantics></math> = 2.4 MHz; and (<b>c</b>) <math display="inline"><semantics> <msub> <mi>F</mi> <mi>S</mi> </msub> </semantics></math> = 20 MHz and <math display="inline"><semantics> <msub> <mi>F</mi> <mrow> <mi>I</mi> <mi>N</mi> </mrow> </msub> </semantics></math> = 3.6 MHz.</p> Full article ">Figure 10
<p>Measured SNR, SNDR, and SFDR versus input frequency at 20 MS/s.</p> Full article ">Figure 11
<p>(<b>a</b>) The measured DNL of the proposed ADC and (<b>b</b>) measured INL of the proposed ADC.</p> Full article ">
<p>An overview of a low-power IoT vibration sensor network. (<b>a</b>) Subsurface seismic sensor deployment for vehicle/pedestrian vibration detection in a smart city, with LoRaWAN communication to an AI node. (<b>b</b>) Signal processing flow in a sensor node, highlighting the analog front-end and proposed low-power pipelined ADC for energy-efficient data conversion.</p> Full article ">Figure 2
<p>Architecture of 10-bit pipelined ADC.</p> Full article ">Figure 3
<p>Structure and timing of first stage and second stage.</p> Full article ">Figure 4
<p>Structure of gate-boosted switch.</p> Full article ">Figure 5
<p>Dual-input, differential-pair, gain-boosted, switched operational amplifier circuit.</p> Full article ">Figure 6
<p>Switched-capacitor common-mode feedback circuit.</p> Full article ">Figure 7
<p>(<b>a</b>) The dynamic comparator with a pre-amplifier and (<b>b</b>) differential-pair dynamic comparator.</p> Full article ">Figure 8
<p>Die photo micrograph.</p> Full article ">Figure 9
<p>Measured ADC output FFT spectra under (<b>a</b>) <math display="inline"><semantics> <msub> <mi>F</mi> <mi>S</mi> </msub> </semantics></math> = 20 MHz and <math display="inline"><semantics> <msub> <mi>F</mi> <mrow> <mi>I</mi> <mi>N</mi> </mrow> </msub> </semantics></math> = 1MHz; (<b>b</b>) <math display="inline"><semantics> <msub> <mi>F</mi> <mi>S</mi> </msub> </semantics></math> = 20 MHz and <math display="inline"><semantics> <msub> <mi>F</mi> <mrow> <mi>I</mi> <mi>N</mi> </mrow> </msub> </semantics></math> = 2.4 MHz; and (<b>c</b>) <math display="inline"><semantics> <msub> <mi>F</mi> <mi>S</mi> </msub> </semantics></math> = 20 MHz and <math display="inline"><semantics> <msub> <mi>F</mi> <mrow> <mi>I</mi> <mi>N</mi> </mrow> </msub> </semantics></math> = 3.6 MHz.</p> Full article ">Figure 10
<p>Measured SNR, SNDR, and SFDR versus input frequency at 20 MS/s.</p> Full article ">Figure 11
<p>(<b>a</b>) The measured DNL of the proposed ADC and (<b>b</b>) measured INL of the proposed ADC.</p> Full article ">
Open AccessSystematic Review
Non-Invasive BCI-VR Applied Protocols as Intervention Paradigms on School-Aged Subjects with ASD: A Systematic Review
by
Archondoula Alexopoulou, Pantelis Pergantis, Constantinos Koutsojannis, Vassilios Triantafillou and Athanasios Drigas
Sensors 2025, 25(5), 1342; https://doi.org/10.3390/s25051342 (registering DOI) - 22 Feb 2025
Abstract
This paper aims to highlight non-invasive BCI-VR applied protocols as intervention paradigms on school-aged subjects with ASD. Computer-based interventions are considered appropriate for users with ASD as concentration on a screen reduces other stimuli from the environment that are likely to be distracting
[...] Read more.
This paper aims to highlight non-invasive BCI-VR applied protocols as intervention paradigms on school-aged subjects with ASD. Computer-based interventions are considered appropriate for users with ASD as concentration on a screen reduces other stimuli from the environment that are likely to be distracting or disruptive. Since there are no social conditions for engagement in such processes and the responses of computing systems do not hold surprises for users, as the outputs are fully controlled, they are ideal for ASD subjects. Children and adolescents with ASD, when supported by BCI interventions through virtual reality applications, especially appear to show significant improvements in core symptoms, such as cognitive and social deficits, regardless of their age or IQ. We examined nine protocols applied from 2016 to 2023, focusing on the BCI paradigms, the procedure, and the outcomes. Our study is non-exhaustive but representative of the state of the art in the field. As concluded by the research, BCI-VR applied protocols have no side effects and are rather easy to handle and maintain, and despite the fact that there are research limitations, they hold promise as a tool for improving social and cognitive skills in school-aged individuals with ASD.
Full article
(This article belongs to the Special Issue Combining Brain-Computer Interfaces and Assistive Biosensing Technologies)
►▼
Show Figures
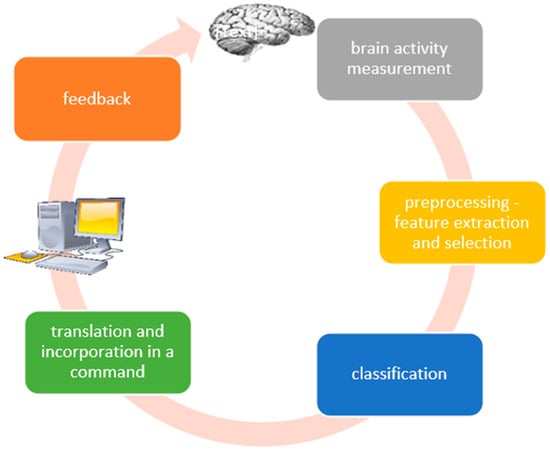
Figure 1
Figure 1
<p>A typical BCI system.</p> Full article ">Figure 2
<p>BCI types.</p> Full article ">Figure 3
<p>PRISMA Flow Diagram.</p> Full article ">Figure 4
<p>Gender representation.</p> Full article ">Figure 5
<p>Study distribution by country.</p> Full article ">Figure 6
<p>Categorised research types.</p> Full article ">
<p>A typical BCI system.</p> Full article ">Figure 2
<p>BCI types.</p> Full article ">Figure 3
<p>PRISMA Flow Diagram.</p> Full article ">Figure 4
<p>Gender representation.</p> Full article ">Figure 5
<p>Study distribution by country.</p> Full article ">Figure 6
<p>Categorised research types.</p> Full article ">
Open AccessArticle
Improved MTF Measurement of Medical Flat-Panel Detectors Based on a Slit Model
by
Haiyang Zhang and Zhiyong Ji
Sensors 2025, 25(5), 1341; https://doi.org/10.3390/s25051341 (registering DOI) - 22 Feb 2025
Abstract
In the development, evaluation, and application of medical flat-panel detectors, the modulation transfer function (MTF) is crucial, as it reflects the device’s ability to restore detailed information. Medical flat-panel detectors encompass both image data acquisition and digitization processes, and detectors with varying pixel
[...] Read more.
In the development, evaluation, and application of medical flat-panel detectors, the modulation transfer function (MTF) is crucial, as it reflects the device’s ability to restore detailed information. Medical flat-panel detectors encompass both image data acquisition and digitization processes, and detectors with varying pixel sizes exhibit differing capabilities for observing details. Accurately quantifying MTF is a critical challenge. The complexity of MTF calculation, combined with unclear principles and details, may result in erroneous outcomes, thereby misleading research and decision-making processes. This paper presents an improved MTF oversampling method based on the slit model. MTF testing is conducted under various sample conditions and using different focal spot diameters of the X-ray tube to analyze the impact of focal spot size. High-precision tungsten plates and fixtures are designed and fabricated, and MTF results with varying line spread function (LSF) sampling intervals are compared. The results demonstrate that the improved slit model offers distinct advantages, with MTF measurements achieving 92.4% of the ideal value. Compared to traditional tungsten edge and point (aperture) model testing methods, the accuracy of the proposed method is improved by 5–13%. The optimal sampling interval is approximately 1/29 of the pixel pitch, offering a more accurate method for evaluating detector performance.
Full article
(This article belongs to the Section Biomedical Sensors)
►▼
Show Figures
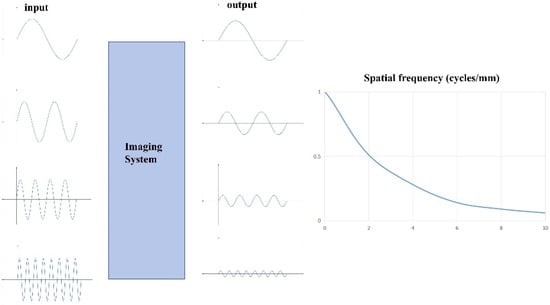
Figure 1
Figure 1
<p>Schematic diagram of the MTF calculation process.</p> Full article ">Figure 2
<p>Comparison of signal input and output with MTF calculation results.</p> Full article ">Figure 3
<p>Comparison of three diffusion functions.</p> Full article ">Figure 4
<p>The schematic diagram of LSF oversampling with slit without tilt angle (<b>A</b>) and with tilt angle (<b>B</b>).</p> Full article ">Figure 5
<p>Comparison of MTF calculation results, before and after improvement.</p> Full article ">Figure 6
<p>Local enlargements of MTF calculation results, before and after improvement.</p> Full article ">Figure 7
<p>Comparison of MTF calculation results for different sampling intervals in the slit model.</p> Full article ">Figure 8
<p>Local magnification of MTF calculation results for different sampling intervals of the slit model.</p> Full article ">Figure 9
<p>The noise level is calculated by MTF under different sampling intervals.</p> Full article ">
<p>Schematic diagram of the MTF calculation process.</p> Full article ">Figure 2
<p>Comparison of signal input and output with MTF calculation results.</p> Full article ">Figure 3
<p>Comparison of three diffusion functions.</p> Full article ">Figure 4
<p>The schematic diagram of LSF oversampling with slit without tilt angle (<b>A</b>) and with tilt angle (<b>B</b>).</p> Full article ">Figure 5
<p>Comparison of MTF calculation results, before and after improvement.</p> Full article ">Figure 6
<p>Local enlargements of MTF calculation results, before and after improvement.</p> Full article ">Figure 7
<p>Comparison of MTF calculation results for different sampling intervals in the slit model.</p> Full article ">Figure 8
<p>Local magnification of MTF calculation results for different sampling intervals of the slit model.</p> Full article ">Figure 9
<p>The noise level is calculated by MTF under different sampling intervals.</p> Full article ">
Open AccessArticle
L-GABS: Parametric Modeling of a Generic Active Lumbar Exoskeleton for Ergonomic Impact Assessment
by
Manuel Pérez-Soto, Javier Marín and José J. Marín
Sensors 2025, 25(5), 1340; https://doi.org/10.3390/s25051340 (registering DOI) - 22 Feb 2025
Abstract
Companies increasingly implement exoskeletons in their production lines to reduce musculoskeletal disorders. Studies have been conducted on the general ergonomic effects of exoskeletons in production environments; however, it remains challenging to predict the biomechanical effects these devices may have in specific jobs. This
[...] Read more.
Companies increasingly implement exoskeletons in their production lines to reduce musculoskeletal disorders. Studies have been conducted on the general ergonomic effects of exoskeletons in production environments; however, it remains challenging to predict the biomechanical effects these devices may have in specific jobs. This article proposes the parametric modeling of an active lumbar exoskeleton using the Forces ergonomic method, which calculates the ergonomic risk using motion capture in the workplace, considering the internal joint forces. The exoskeleton was studied to model it in the Forces method using a four-phase approach based on experimental observations (Phase 1) and objective data collection via motion capture with inertial sensors and load cells for lifting load movements. From the experimentation the angles of each body segment, the effort perceived by the user, and the activation conditions were obtained (Phase 2). After modeling development (Phase 3), the experimental results regarding the force and risk were evaluated obtaining differences between model and experimental data of 0.971 ± 0.171 kg in chest force and 1.983 ± 0.678% in lumbar risk (Phase 4). This approach provides a tool to evaluate the biomechanical effects of this device in a work task, offering a parametric and direct approximation of the effects prior to implementation.
Full article
(This article belongs to the Special Issue Wearable Robotics and Assistive Devices)
►▼
Show Figures
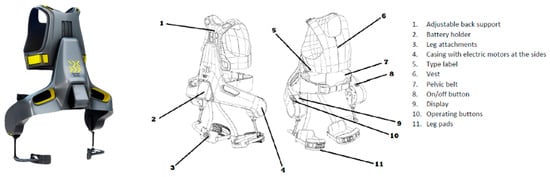
Figure 1
Figure 1
<p>Apogee active exoskeleton diagram (images from Apogee User Manual).</p> Full article ">Figure 2
<p>Apogee exoskeleton configuration examples and legend.</p> Full article ">Figure 3
<p>Model diagram forces (right: R, left: L) from Delgado et al. [<a href="#B15-sensors-25-01340" class="html-bibr">15</a>] modified for Apogee Exoskeleton.</p> Full article ">Figure 4
<p>Load cell assembly for force measurement. Experimentation with motion capture and synchronized force measurement. CAD drawing of load cell assembly (1: Load cell, 2: Ad hoc 3D printed part with a threaded insert, 3: Adjustable back support, 4: Casing with electric motors at the side).</p> Full article ">Figure 5
<p>Experimental diagram.</p> Full article ">Figure 6
<p>(<b>a</b>) Averaged chest force curves experimentally obtained concerning lumbar flexion. (<b>b</b>) Modeled passive torque curves on each side.</p> Full article ">Figure 7
<p>(<b>a</b>) Averaged chest force curves obtained experimentally concerning lumbar angular velocity. (<b>b</b>) Modeled active torque curves on each side.</p> Full article ">Figure 8
<p>Force measured on the chest compared to the force simulated by the model. (<b>a</b>) Passive assistance comparison. (<b>b</b>) Active assistance comparison. (<b>c</b>) Active Mod. assistance comparison.</p> Full article ">Figure 8 Cont.
<p>Force measured on the chest compared to the force simulated by the model. (<b>a</b>) Passive assistance comparison. (<b>b</b>) Active assistance comparison. (<b>c</b>) Active Mod. assistance comparison.</p> Full article ">Figure 9
<p>Comparison of the hybrid assistance. Force measured on the chest compared to force simulated by the model.</p> Full article ">
<p>Apogee active exoskeleton diagram (images from Apogee User Manual).</p> Full article ">Figure 2
<p>Apogee exoskeleton configuration examples and legend.</p> Full article ">Figure 3
<p>Model diagram forces (right: R, left: L) from Delgado et al. [<a href="#B15-sensors-25-01340" class="html-bibr">15</a>] modified for Apogee Exoskeleton.</p> Full article ">Figure 4
<p>Load cell assembly for force measurement. Experimentation with motion capture and synchronized force measurement. CAD drawing of load cell assembly (1: Load cell, 2: Ad hoc 3D printed part with a threaded insert, 3: Adjustable back support, 4: Casing with electric motors at the side).</p> Full article ">Figure 5
<p>Experimental diagram.</p> Full article ">Figure 6
<p>(<b>a</b>) Averaged chest force curves experimentally obtained concerning lumbar flexion. (<b>b</b>) Modeled passive torque curves on each side.</p> Full article ">Figure 7
<p>(<b>a</b>) Averaged chest force curves obtained experimentally concerning lumbar angular velocity. (<b>b</b>) Modeled active torque curves on each side.</p> Full article ">Figure 8
<p>Force measured on the chest compared to the force simulated by the model. (<b>a</b>) Passive assistance comparison. (<b>b</b>) Active assistance comparison. (<b>c</b>) Active Mod. assistance comparison.</p> Full article ">Figure 8 Cont.
<p>Force measured on the chest compared to the force simulated by the model. (<b>a</b>) Passive assistance comparison. (<b>b</b>) Active assistance comparison. (<b>c</b>) Active Mod. assistance comparison.</p> Full article ">Figure 9
<p>Comparison of the hybrid assistance. Force measured on the chest compared to force simulated by the model.</p> Full article ">
Open AccessArticle
The Multi-Station Fusion-Based Radiation Source Localization Method Based on Spectrum Energy
by
Guojin He, Yulong Hao and Yaocong Xie
Sensors 2025, 25(5), 1339; https://doi.org/10.3390/s25051339 (registering DOI) - 22 Feb 2025
Abstract
Today’s highly complex and rapidly changing electromagnetic environment places higher demands on the precise localization of illegal radiation sources. In response to this, this paper innovatively proposes a multi-station fusion-based radiation source localization method, which leverages the frequency, field strength, bandwidth, and other
[...] Read more.
Today’s highly complex and rapidly changing electromagnetic environment places higher demands on the precise localization of illegal radiation sources. In response to this, this paper innovatively proposes a multi-station fusion-based radiation source localization method, which leverages the frequency, field strength, bandwidth, and other characteristic information embedded in frequency band scanning data. The method thoroughly explores the energy characteristics of the detected radiation sources while closely integrating the objective laws of propagation attenuation in electromagnetic space. By employing an advanced, normalized power calculation technique, it successfully achieves high-precision localization of the radiation source. Through rigorous and thorough experimental validation, this method reduces localization errors by 25% and cuts the equivalent radiation power error by 30% compared to traditional localization methods. This achievement provides more reliable and accurate technical support for applications in the electromagnetic field, offering promising prospects for advancing and refining electromagnetic environment monitoring and management technologies.
Full article
(This article belongs to the Section Navigation and Positioning)
►▼
Show Figures
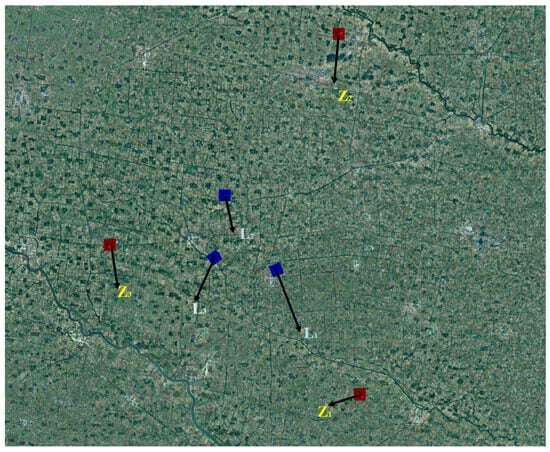
Figure 1
Figure 1
<p>The locations of the monitoring stations and radiation source deployment.</p> Full article ">Figure 2
<p>The connection diagram of the experimental monitoring system.</p> Full article ">Figure 3
<p>Spectrum monitoring plot for station <span class="html-italic">Z</span><sub>1</sub>.</p> Full article ">Figure 4
<p>Spectrum monitoring plot for station <span class="html-italic">Z</span><sub>2</sub>.</p> Full article ">Figure 5
<p>Spectrum monitoring plot for station <span class="html-italic">Z</span><sub>3</sub>.</p> Full article ">Figure 6
<p>Comparison between calculated results and actual information.</p> Full article ">
<p>The locations of the monitoring stations and radiation source deployment.</p> Full article ">Figure 2
<p>The connection diagram of the experimental monitoring system.</p> Full article ">Figure 3
<p>Spectrum monitoring plot for station <span class="html-italic">Z</span><sub>1</sub>.</p> Full article ">Figure 4
<p>Spectrum monitoring plot for station <span class="html-italic">Z</span><sub>2</sub>.</p> Full article ">Figure 5
<p>Spectrum monitoring plot for station <span class="html-italic">Z</span><sub>3</sub>.</p> Full article ">Figure 6
<p>Comparison between calculated results and actual information.</p> Full article ">
Open AccessArticle
A High-Sensitivity Inkjet-Printed Flexible Resonator for Monitoring Dielectric Changes in Meat
by
Jamal Abounasr, Mariam El Gharbi, Raúl Fernández García and Ignacio Gil
Sensors 2025, 25(5), 1338; https://doi.org/10.3390/s25051338 (registering DOI) - 22 Feb 2025
Abstract
This paper introduces a flexible loop antenna-based sensor optimized for real-time monitoring of meat quality by detecting changes in dielectric properties over a six-day storage period. Operating within the 2.4 GHz ISM band, the sensor is designed using CST Microwave Studio 2024 to
[...] Read more.
This paper introduces a flexible loop antenna-based sensor optimized for real-time monitoring of meat quality by detecting changes in dielectric properties over a six-day storage period. Operating within the 2.4 GHz ISM band, the sensor is designed using CST Microwave Studio 2024 to deliver high sensitivity and accuracy. The sensing mechanism leverages resonance frequency shifts caused by variations in permittivity as the meat degrades. Experimental validation across five samples showed a consistent frequency shift from 2.14 GHz (Day 0) to 1.29 GHz (Day 5), with an average sensitivity of . A strong correlation was observed between measured and simulated results, as evidenced by linear regression ( and for measured and simulated data, respectively). The sensor demonstrated high precision and repeatability, validated by low standard deviations and minimal frequency deviations. Compact, printable, and cost-effective, the proposed sensor offers a scalable solution for food quality monitoring. Its robust performance highlights its potential for integration into IoT platforms and extension to other perishable food products, advancing real-time, non-invasive, RF-based food safety technologies.
Full article
(This article belongs to the Special Issue Applications of Antenna Technology in Sensors II)
►▼
Show Figures
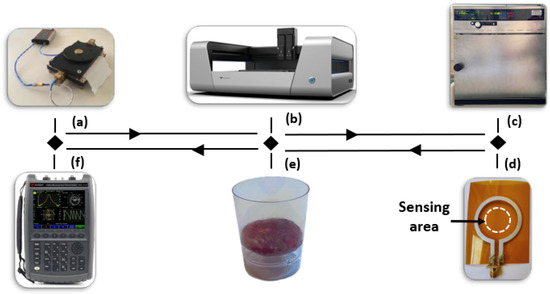
Figure 1
Figure 1
<p>Overview of the experimental setup and fabrication process: (<b>a</b>) Q-meter used for extracting electrical properties of the substrate; (<b>b</b>) Voltera NOVA inkjet and extrusion printer used for antenna fabrication; (<b>c</b>) Memmert oven for drying and curing the printed ink to ensure proper adhesion and distribution; (<b>d</b>) final fabricated loop antenna; (<b>e</b>) 10 g of meat sample; (<b>f</b>) vector network analyzer (VNA) for antenna performance validation.</p> Full article ">Figure 2
<p>The geometrical parameters of the proposed antenna.</p> Full article ">Figure 3
<p>Simulated electric field highlighting the interactions between the loop antenna and the meat sample: (<b>a</b>) side view and (<b>b</b>) 3D view of the E field.</p> Full article ">Figure 4
<p>Three-dimensional model representation of the experimental protocol for assessing meat quality using microwave sensing. The model depicts fresh samples (Day 0) and aged samples (Day 5) placed in containers, connected to a microwave sensing setup and a vector network analyzer (VNA).</p> Full article ">Figure 5
<p>Comprehensive experimental setup for analyzing dielectric property variations in meat samples from Day 0 to Day 5.</p> Full article ">Figure 6
<p>Comparison of measured and simulated <math display="inline"><semantics> <msub> <mi>S</mi> <mn>11</mn> </msub> </semantics></math> in free space.</p> Full article ">Figure 7
<p>Simulated <math display="inline"><semantics> <msub> <mi>S</mi> <mn>11</mn> </msub> </semantics></math> response over the frequency range for various permittivity values corresponding to different days.</p> Full article ">Figure 8
<p>Dynamic relationship between simulated permittivity and frequency across days.</p> Full article ">Figure 9
<p>Average (<math display="inline"><semantics> <msub> <mi>S</mi> <mn>11</mn> </msub> </semantics></math>) measurements over six days.</p> Full article ">Figure 10
<p>Trend of measured resonance frequencies across individual samples over six days.</p> Full article ">Figure 11
<p>Measured minimum <math display="inline"><semantics> <msub> <mi>S</mi> <mn>11</mn> </msub> </semantics></math> amplitudes with average and error bars over days.</p> Full article ">Figure 12
<p>Linear regression analysis of measured and simulated resonant frequencies over time.</p> Full article ">
<p>Overview of the experimental setup and fabrication process: (<b>a</b>) Q-meter used for extracting electrical properties of the substrate; (<b>b</b>) Voltera NOVA inkjet and extrusion printer used for antenna fabrication; (<b>c</b>) Memmert oven for drying and curing the printed ink to ensure proper adhesion and distribution; (<b>d</b>) final fabricated loop antenna; (<b>e</b>) 10 g of meat sample; (<b>f</b>) vector network analyzer (VNA) for antenna performance validation.</p> Full article ">Figure 2
<p>The geometrical parameters of the proposed antenna.</p> Full article ">Figure 3
<p>Simulated electric field highlighting the interactions between the loop antenna and the meat sample: (<b>a</b>) side view and (<b>b</b>) 3D view of the E field.</p> Full article ">Figure 4
<p>Three-dimensional model representation of the experimental protocol for assessing meat quality using microwave sensing. The model depicts fresh samples (Day 0) and aged samples (Day 5) placed in containers, connected to a microwave sensing setup and a vector network analyzer (VNA).</p> Full article ">Figure 5
<p>Comprehensive experimental setup for analyzing dielectric property variations in meat samples from Day 0 to Day 5.</p> Full article ">Figure 6
<p>Comparison of measured and simulated <math display="inline"><semantics> <msub> <mi>S</mi> <mn>11</mn> </msub> </semantics></math> in free space.</p> Full article ">Figure 7
<p>Simulated <math display="inline"><semantics> <msub> <mi>S</mi> <mn>11</mn> </msub> </semantics></math> response over the frequency range for various permittivity values corresponding to different days.</p> Full article ">Figure 8
<p>Dynamic relationship between simulated permittivity and frequency across days.</p> Full article ">Figure 9
<p>Average (<math display="inline"><semantics> <msub> <mi>S</mi> <mn>11</mn> </msub> </semantics></math>) measurements over six days.</p> Full article ">Figure 10
<p>Trend of measured resonance frequencies across individual samples over six days.</p> Full article ">Figure 11
<p>Measured minimum <math display="inline"><semantics> <msub> <mi>S</mi> <mn>11</mn> </msub> </semantics></math> amplitudes with average and error bars over days.</p> Full article ">Figure 12
<p>Linear regression analysis of measured and simulated resonant frequencies over time.</p> Full article ">
Open AccessArticle
Anti-Interference Spectral Confocal Sensors Based on Line Spot
by
Bo Wang, Jiafu Li, Mingzhe Luo, Fengshuang Liang and Jiacheng Hu
Sensors 2025, 25(5), 1337; https://doi.org/10.3390/s25051337 (registering DOI) - 22 Feb 2025
Abstract
Spectral confocal displacement sensors are non-contact optoelectronic sensors widely utilized for their high accuracy, speed, and ability to measure diverse surfaces. However, challenges including vibration, angular deflection, and surface quality variations can reduce sensor stability and accuracy when performing measurements such as lithium
[...] Read more.
Spectral confocal displacement sensors are non-contact optoelectronic sensors widely utilized for their high accuracy, speed, and ability to measure diverse surfaces. However, challenges including vibration, angular deflection, and surface quality variations can reduce sensor stability and accuracy when performing measurements such as lithium battery wafer thickness, wafer warpage, and optical component surface topography. This study proposes a line-spot-based measurement method using a binary diffractive lens and cylindrical lens with a 20× objective, and then the overall structure is simulated and optimized by using ZEMAX, which realizes a confocal measurement system with a measurement range of 800 μm, line spot length of 3.8 mm, and width of 0.2 mm. The system, calibrated with a nanometer displacement stage, achieved 30 nm resolution and significantly improved dynamic stability (standard deviation (SD) of 0.013 μm) compared to a point spectral confocal sensor (SD of 0.064 μm). The results indicate the proposed sensor exhibits improved stability during scanning measurements.
Full article
(This article belongs to the Section Physical Sensors)
►▼
Show Figures
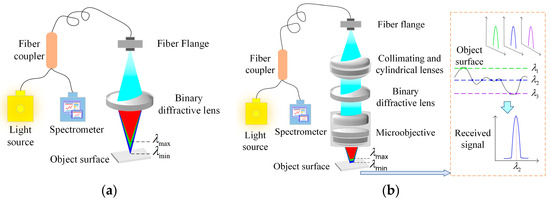
Figure 1
Figure 1
<p>Spectral confocal sensor measurement principle: (<b>a</b>) Conventional diffractive lens point confocal sensor measurement system; (<b>b</b>) The line spot confocal sensor measurement system proposed in this paper.</p> Full article ">Figure 2
<p>Structure of the proposed line spot spectral confocal sensor.</p> Full article ">Figure 3
<p>Simulation results of optimized binary diffraction lens at 400–700 nm: (<b>a</b>) Axial chromatic aberration curve; (<b>b</b>) Chromatic focal shift curve for binary diffractive lens.</p> Full article ">Figure 4
<p>Image quality analysis of the microscope objective at four specific wavelengths: (<b>a</b>) 480 nm (blue) spot diagram and MTF curve; (<b>b</b>) 550 nm (green) spot diagram and MTF curve; (<b>c</b>) 600 nm (red) spot diagram and MTF curve; (<b>d</b>) 650 nm (yellow) spot diagram and MTF curve.</p> Full article ">Figure 4 Cont.
<p>Image quality analysis of the microscope objective at four specific wavelengths: (<b>a</b>) 480 nm (blue) spot diagram and MTF curve; (<b>b</b>) 550 nm (green) spot diagram and MTF curve; (<b>c</b>) 600 nm (red) spot diagram and MTF curve; (<b>d</b>) 650 nm (yellow) spot diagram and MTF curve.</p> Full article ">Figure 5
<p>Simulation analysis of the overall structure after ZEMAX optimization: (<b>a</b>) Chromatic focus shift curve of line spot spectral confocal sensor between 480 and 650 nm; (<b>b</b>) 480nm (blue), 550 nm (green), 650 nm (red) defocusing between −400~400 μm.</p> Full article ">Figure 6
<p>PSF analysis of the spot generated by a line spectral confocal sensor and the spot received by a spectrometer: (<b>a</b>) Image plane Huygens PSF simulation, (<b>b</b>) Return signal PSF transverse cross-section distribution, (<b>c</b>) Return signal PSF longitudinal cross-section distribution.</p> Full article ">Figure 7
<p>Experimental platform for calibration. A, B indicates two base adjustment brackets, and C represents a localized magnified view of the lens and the subject.</p> Full article ">Figure 8
<p>Line spot sensor calibration results and measurement errors: (<b>a</b>) Line spot 800 μm calibration curve and some interval fitting error; (<b>b</b>) Line spot full-scale measurement error curve.</p> Full article ">Figure 9
<p>Point spot sensor calibration results and measurement errors: (<b>a</b>) Point spot 800 μm calibration curve and some interval fitting error; (<b>b</b>) Point spot full-scale measurement error curve.</p> Full article ">Figure 10
<p>Experimental results of axial resolution for different steps: (<b>a</b>) 20 nm; (<b>b</b>) 30 nm; (<b>c</b>) 40 nm; (<b>d</b>) 50 nm.</p> Full article ">Figure 11
<p>Static stability analysis: (<b>a</b>) Point-line spot static stability differences; (<b>b</b>) Point spot static stability frequency histogram and Gaussian fitting; (<b>c</b>) Line spot static stability frequency histogram and Gaussian fitting.</p> Full article ">Figure 11 Cont.
<p>Static stability analysis: (<b>a</b>) Point-line spot static stability differences; (<b>b</b>) Point spot static stability frequency histogram and Gaussian fitting; (<b>c</b>) Line spot static stability frequency histogram and Gaussian fitting.</p> Full article ">Figure 12
<p>Dynamic stability analysis: (<b>a</b>) Point-Line spot dynamic stability differences; (<b>b</b>) Point spot dynamic stability frequency histogram and Gaussian fitting; (<b>c</b>) Line spot dynamic stability frequency histogram and Gaussian fitting.</p> Full article ">Figure 13
<p>Relative position of the scanning spot to the step and one complete scanning process.</p> Full article ">Figure 14
<p>Measurement error curves for each step.</p> Full article ">
<p>Spectral confocal sensor measurement principle: (<b>a</b>) Conventional diffractive lens point confocal sensor measurement system; (<b>b</b>) The line spot confocal sensor measurement system proposed in this paper.</p> Full article ">Figure 2
<p>Structure of the proposed line spot spectral confocal sensor.</p> Full article ">Figure 3
<p>Simulation results of optimized binary diffraction lens at 400–700 nm: (<b>a</b>) Axial chromatic aberration curve; (<b>b</b>) Chromatic focal shift curve for binary diffractive lens.</p> Full article ">Figure 4
<p>Image quality analysis of the microscope objective at four specific wavelengths: (<b>a</b>) 480 nm (blue) spot diagram and MTF curve; (<b>b</b>) 550 nm (green) spot diagram and MTF curve; (<b>c</b>) 600 nm (red) spot diagram and MTF curve; (<b>d</b>) 650 nm (yellow) spot diagram and MTF curve.</p> Full article ">Figure 4 Cont.
<p>Image quality analysis of the microscope objective at four specific wavelengths: (<b>a</b>) 480 nm (blue) spot diagram and MTF curve; (<b>b</b>) 550 nm (green) spot diagram and MTF curve; (<b>c</b>) 600 nm (red) spot diagram and MTF curve; (<b>d</b>) 650 nm (yellow) spot diagram and MTF curve.</p> Full article ">Figure 5
<p>Simulation analysis of the overall structure after ZEMAX optimization: (<b>a</b>) Chromatic focus shift curve of line spot spectral confocal sensor between 480 and 650 nm; (<b>b</b>) 480nm (blue), 550 nm (green), 650 nm (red) defocusing between −400~400 μm.</p> Full article ">Figure 6
<p>PSF analysis of the spot generated by a line spectral confocal sensor and the spot received by a spectrometer: (<b>a</b>) Image plane Huygens PSF simulation, (<b>b</b>) Return signal PSF transverse cross-section distribution, (<b>c</b>) Return signal PSF longitudinal cross-section distribution.</p> Full article ">Figure 7
<p>Experimental platform for calibration. A, B indicates two base adjustment brackets, and C represents a localized magnified view of the lens and the subject.</p> Full article ">Figure 8
<p>Line spot sensor calibration results and measurement errors: (<b>a</b>) Line spot 800 μm calibration curve and some interval fitting error; (<b>b</b>) Line spot full-scale measurement error curve.</p> Full article ">Figure 9
<p>Point spot sensor calibration results and measurement errors: (<b>a</b>) Point spot 800 μm calibration curve and some interval fitting error; (<b>b</b>) Point spot full-scale measurement error curve.</p> Full article ">Figure 10
<p>Experimental results of axial resolution for different steps: (<b>a</b>) 20 nm; (<b>b</b>) 30 nm; (<b>c</b>) 40 nm; (<b>d</b>) 50 nm.</p> Full article ">Figure 11
<p>Static stability analysis: (<b>a</b>) Point-line spot static stability differences; (<b>b</b>) Point spot static stability frequency histogram and Gaussian fitting; (<b>c</b>) Line spot static stability frequency histogram and Gaussian fitting.</p> Full article ">Figure 11 Cont.
<p>Static stability analysis: (<b>a</b>) Point-line spot static stability differences; (<b>b</b>) Point spot static stability frequency histogram and Gaussian fitting; (<b>c</b>) Line spot static stability frequency histogram and Gaussian fitting.</p> Full article ">Figure 12
<p>Dynamic stability analysis: (<b>a</b>) Point-Line spot dynamic stability differences; (<b>b</b>) Point spot dynamic stability frequency histogram and Gaussian fitting; (<b>c</b>) Line spot dynamic stability frequency histogram and Gaussian fitting.</p> Full article ">Figure 13
<p>Relative position of the scanning spot to the step and one complete scanning process.</p> Full article ">Figure 14
<p>Measurement error curves for each step.</p> Full article ">
Open AccessArticle
High-Performance ZIF-7@PANI Electrochemical Sensor for Simultaneous Trace Cadmium and Lead Detection in Water Samples: A Box–Behnken Design Optimization Approach
by
Sondes Guesmi, Nashwan H. Ali, Nadhem Missaoui, Zouhaier Aloui, Chama Mabrouk, Carlos Castilla Martinez, Fraj Echouchene, Houcine Barhoumi, Nicole Jaffrezic-Renault and Hamza Kahri
Sensors 2025, 25(5), 1336; https://doi.org/10.3390/s25051336 (registering DOI) - 22 Feb 2025
Abstract
This study presents the development of an innovative electrochemical sensor for the simultaneous detection of cadmium (Cd2+) and lead (Pb2+) ions in environmental samples. The sensor is developed based on a composite material of zeolite imidazolate framework ZIF-7 and
[...] Read more.
This study presents the development of an innovative electrochemical sensor for the simultaneous detection of cadmium (Cd2+) and lead (Pb2+) ions in environmental samples. The sensor is developed based on a composite material of zeolite imidazolate framework ZIF-7 and polyaniline (PANI), referred to as ZIF-7@PANI, where ZIF-7 is rapidly synthesized at room temperature and polyaniline used to enhance the conductivity of the composite. Characterization via X-ray diffraction (DRX), scanning electron microscopy (SEM), and Fourier transform infrared spectroscopy (FTIR) confirmed successful synthesis. The composite was applied to a glassy carbon electrode (GCE) using drop-casting for heavy metal ion detection. Experimental parameters—including pH, incubation time, deposited quantity, and drying time—were optimized using the Box–Behnken design. Under optimal conditions, the ZIF-7@PANI/GCE sensor demonstrated a broad dynamic concentration range (0.002–1 µM for Pb2+ and 0.02–30 µM for Cd2+), with low detection limits (2.96 nM for Pb2+ and 10.6 nM for Cd2+). It also exhibited strong anti-interference properties and high recovery rates (85–110%), highlighting its potential for real practical applications.
Full article
(This article belongs to the Section Chemical Sensors)
►▼
Show Figures
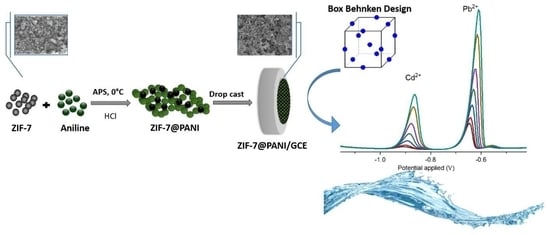
Graphical abstract
Graphical abstract
Full article ">Figure 1
<p>FTIR spectra of PANI, synthesized ZIF-7 and ZIF-7@PANI composite.</p> Full article ">Figure 2
<p>The powder X-ray diffraction patterns of the ZIF-7 and ZIF-7@PANI.</p> Full article ">Figure 3
<p>SEM images of PANI/SPCE (<b>a</b>,<b>b</b>), ZIF-7/SPCE (<b>c</b>,<b>d</b>), and ZIF-7@PANI/SPCE (<b>e</b>,<b>f</b>). Images (<b>a</b>,<b>c</b>,<b>e</b>) are taken at 25,000× magnification with a scale bar of 400 nm, while images (<b>b</b>,<b>d</b>,<b>f</b>) are taken at 100,000× magnification with a scale bar of 100 nm.</p> Full article ">Figure 4
<p>(<b>a</b>) CV and (<b>b</b>) EIS curves of bare GCE, ZIF-7/GCE, PANI/GCE, and ZIF-7@PANI/GCE in 0.1 M KCl solution containing 5 mM Fe(CN)<sub>6</sub><sup>−3/−4</sup>.</p> Full article ">Figure 5
<p>DPV curves of a 10 µM mixture of Cd<sup>2+</sup> and Pb<sup>2+</sup> ions in 0.1 m acetate buffer (pH 5) using different sensors.</p> Full article ">Figure 6
<p>Statistical analysis fit of the RSM model (<b>a</b>) for Cd<sup>2+</sup> current response and (<b>b</b>) for Pb<sup>2+</sup> current response.</p> Full article ">Figure 7
<p>Optimization plot using desirability function.</p> Full article ">Figure 8
<p>DPV curves of the ZIF-7@PANI/GCE sensor for the individual detection of (<b>a</b>) Pb<sup>2+</sup> and (<b>c</b>) Cd<sup>2+</sup> ions at different concentration ranges from 0.02 µM to 30 µM. Calibration curves of (<b>b</b>) Pb<sup>2+</sup> and (<b>d</b>) Cd<sup>2+</sup>.</p> Full article ">Figure 9
<p>(<b>a</b>) DPV curves of ZIF-7@PANI/GCE sensor for the simultaneous analysis of Pb<sup>2+</sup> and Cd<sup>2+</sup> across concentrations from 0.002 µM to 30 µM. The calibration curves of (<b>b</b>) Pb<sup>2+</sup> and (<b>c</b>) Cd<sup>2+</sup>.</p> Full article ">Figure 10
<p>The specificity of the ZIF-7@PANI/GCE sensor toward 5 μM Cd<sup>2+</sup> and Pb<sup>2+</sup> against 50 µM Ca<sup>2+</sup>, Co<sup>2+</sup>, Mg<sup>2+</sup>, Mn<sup>2+</sup>, Zn<sup>2+</sup>, and Cu<sup>2+</sup>.</p> Full article ">Scheme 1
<p>Schematic illustration of ZIF-7@PANI composite synthesis.</p> Full article ">
Full article ">Figure 1
<p>FTIR spectra of PANI, synthesized ZIF-7 and ZIF-7@PANI composite.</p> Full article ">Figure 2
<p>The powder X-ray diffraction patterns of the ZIF-7 and ZIF-7@PANI.</p> Full article ">Figure 3
<p>SEM images of PANI/SPCE (<b>a</b>,<b>b</b>), ZIF-7/SPCE (<b>c</b>,<b>d</b>), and ZIF-7@PANI/SPCE (<b>e</b>,<b>f</b>). Images (<b>a</b>,<b>c</b>,<b>e</b>) are taken at 25,000× magnification with a scale bar of 400 nm, while images (<b>b</b>,<b>d</b>,<b>f</b>) are taken at 100,000× magnification with a scale bar of 100 nm.</p> Full article ">Figure 4
<p>(<b>a</b>) CV and (<b>b</b>) EIS curves of bare GCE, ZIF-7/GCE, PANI/GCE, and ZIF-7@PANI/GCE in 0.1 M KCl solution containing 5 mM Fe(CN)<sub>6</sub><sup>−3/−4</sup>.</p> Full article ">Figure 5
<p>DPV curves of a 10 µM mixture of Cd<sup>2+</sup> and Pb<sup>2+</sup> ions in 0.1 m acetate buffer (pH 5) using different sensors.</p> Full article ">Figure 6
<p>Statistical analysis fit of the RSM model (<b>a</b>) for Cd<sup>2+</sup> current response and (<b>b</b>) for Pb<sup>2+</sup> current response.</p> Full article ">Figure 7
<p>Optimization plot using desirability function.</p> Full article ">Figure 8
<p>DPV curves of the ZIF-7@PANI/GCE sensor for the individual detection of (<b>a</b>) Pb<sup>2+</sup> and (<b>c</b>) Cd<sup>2+</sup> ions at different concentration ranges from 0.02 µM to 30 µM. Calibration curves of (<b>b</b>) Pb<sup>2+</sup> and (<b>d</b>) Cd<sup>2+</sup>.</p> Full article ">Figure 9
<p>(<b>a</b>) DPV curves of ZIF-7@PANI/GCE sensor for the simultaneous analysis of Pb<sup>2+</sup> and Cd<sup>2+</sup> across concentrations from 0.002 µM to 30 µM. The calibration curves of (<b>b</b>) Pb<sup>2+</sup> and (<b>c</b>) Cd<sup>2+</sup>.</p> Full article ">Figure 10
<p>The specificity of the ZIF-7@PANI/GCE sensor toward 5 μM Cd<sup>2+</sup> and Pb<sup>2+</sup> against 50 µM Ca<sup>2+</sup>, Co<sup>2+</sup>, Mg<sup>2+</sup>, Mn<sup>2+</sup>, Zn<sup>2+</sup>, and Cu<sup>2+</sup>.</p> Full article ">Scheme 1
<p>Schematic illustration of ZIF-7@PANI composite synthesis.</p> Full article ">
Open AccessArticle
Investigation of Trajectory Tracking Control in Hip Joints of Lower-Limb Exoskeletons Using SSA-Fuzzy PID Optimization
by
Wei Li, Xiaojie Wei, Dawen Sun, Siyu Zong and Zhengwei Yue
Sensors 2025, 25(5), 1335; https://doi.org/10.3390/s25051335 (registering DOI) - 22 Feb 2025
Abstract
The application of lower-limb exoskeleton robots in rehabilitation is becoming more prevalent, where the precision of control and the speed of response are essential for effective movement tracking. This study tackles the challenge of optimizing both control accuracy and response speed in trajectory
[...] Read more.
The application of lower-limb exoskeleton robots in rehabilitation is becoming more prevalent, where the precision of control and the speed of response are essential for effective movement tracking. This study tackles the challenge of optimizing both control accuracy and response speed in trajectory tracking for lower-limb exoskeleton hip robots. We introduce an optimization strategy that integrates the Sparrow Search Algorithm (SSA) with fuzzy Proportional-Integral-Derivative (PID) control. This approach addresses the inefficiencies and time-consuming process of manual parameter tuning, thereby improving trajectory tracking performance. First, recognizing the complexity of hip joint motion, which involves multiple degrees of freedom and intricate dynamics, we employed the Lagrangian method. This method is particularly effective for handling nonlinear systems and simplifying the modeling process, allowing for the development of a dynamic model for the hip joint. The SSA is subsequently utilized for the online self-tuning optimization of both the proportional and quantization factors within the fuzzy PID controller. Simulation experiments confirm the efficacy of this strategy in tracking hip joint trajectories during flat walking and standing hip flexion rehabilitation exercises. Experimental results from diverse test populations demonstrate that SSA-fuzzy PID control improves response times by 27.8% (for flat walking) and 30% (for standing hip flexion) when compared to traditional PID control, and by 6% and 2%, respectively, relative to fuzzy PID control. Regarding tracking accuracy, the SSA-fuzzy PID approach increases accuracy by 81.4% (for flat walking) and 80% (for standing hip flexion) when compared to PID control, and by 57.5% and 56.8% relative to fuzzy PID control. The proposed strategy significantly improves both control accuracy and response speed, offering substantial theoretical support for rehabilitation training in individuals with lower-limb impairments. Moreover, in comparison to existing methods, this approach uniquely tackles the challenges of parameter tuning and optimization, presenting a more efficient solution for trajectory tracking in exoskeleton systems.
Full article
(This article belongs to the Section Biomedical Sensors)
►▼
Show Figures
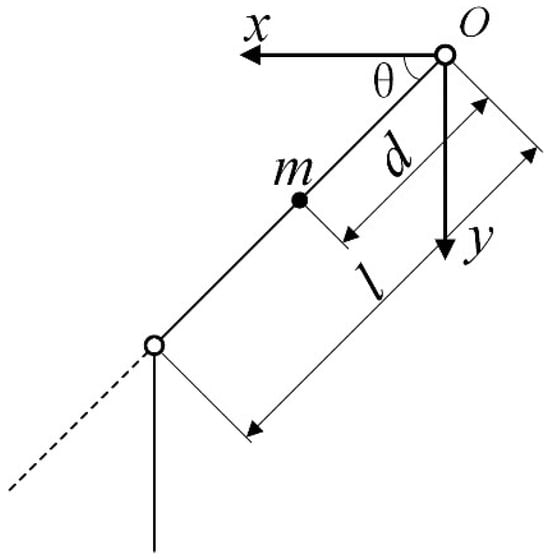
Figure 1
Figure 1
<p>Dynamic model of the hip joint.</p> Full article ">Figure 2
<p>Structure of the PID control system.</p> Full article ">Figure 3
<p>Principle of the fuzzy PID controller structure.</p> Full article ">Figure 4
<p>Membership function of input and output variables.</p> Full article ">Figure 5
<p>Fuzzy surfaces of Δk<sub>p</sub>, Δk<sub>i</sub>, and Δk<sub>d</sub>.</p> Full article ">Figure 6
<p>Flowchart of SSA-fuzzy PID parameter optimization.</p> Full article ">Figure 7
<p>Iteration plot of SSA algorithm adaptation degree.</p> Full article ">Figure 8
<p>Parameter variations in the optimized SSA algorithm system. (<b>a</b>) Change in Ke and Kec parameters; (<b>b</b>) change in Ckp, Cki and Ckd parameters.</p> Full article ">Figure 9
<p>Simulation models. (<b>a</b>) Proportional-Integral-Derivative (PID) controller; (<b>b</b>) fuzzy PID controller.</p> Full article ">Figure 10
<p>Simulation model of the Sparrow Search Algorithm (SSA)-based fuzzy PID controller.</p> Full article ">Figure 11
<p>Comparison of hip joint motion trajectory tracking curves during human-level walking using three control methods.</p> Full article ">Figure 12
<p>Comparison of tracking errors in hip joint motion trajectory during human-level walking using three control methods.</p> Full article ">Figure 13
<p>Comparison of trajectory tracking curves for standing hip flexion motion using three control methods.</p> Full article ">Figure 14
<p>Comparison of tracking errors in standing hip flexion trajectory using three control methods.</p> Full article ">Figure 15
<p>Experimental platform for the lower-limb exoskeleton hip robot. (<b>a</b>) Experimental platform; (<b>b</b>) lower-limb exoskeleton hip robot.</p> Full article ">Figure 16
<p>Motion data acquisition process for Testers A, B, and C wearing the lower-limb exoskeleton device. (<b>a</b>) Acquisition of motion data for Testers A, B, and C while walking on level ground; (<b>b</b>) collection of standing hip flexion movement data for Testers A, B, and C.</p> Full article ">Figure 17
<p>Comparison of hip joint trajectories and tracking errors during level walking for Testers A, B, and C under the three control methods. (<b>a</b>) Comparison of hip joint trajectory tracking curves during level walking for Tester A; (<b>b</b>) comparison of hip joint trajectory tracking errors during level walking for Tester A; (<b>c</b>) comparison of hip joint trajectory tracking curves during level walking for Tester B; (<b>d</b>) comparison of hip joint trajectory tracking errors during level walking for Tester B; (<b>e</b>) comparison of hip joint trajectory tracking curves during level walking for Tester C; (<b>f</b>) comparison of hip joint trajectory tracking errors during level walking for Tester C.</p> Full article ">Figure 18
<p>Comparison of the trajectories and tracking errors of standing hip flexion movements for Testers A, B, and C under the three control methods. (<b>a</b>) Comparison of trajectory tracking curves for Tester A’s standing hip flexion movement; (<b>b</b>) comparison of tracking errors for Tester A’s standing hip flexion trajectory; (<b>c</b>) comparison of trajectory tracking curves for Tester B’s standing hip flexion movement; (<b>d</b>) comparison of tracking errors for Tester B’s standing hip flexion trajectory; (<b>e</b>) comparison of trajectory tracking curves for Tester C’s standing hip flexion movement; (<b>f</b>) comparison of tracking errors for Tester C’s standing hip flexion trajectory.</p> Full article ">Figure 18 Cont.
<p>Comparison of the trajectories and tracking errors of standing hip flexion movements for Testers A, B, and C under the three control methods. (<b>a</b>) Comparison of trajectory tracking curves for Tester A’s standing hip flexion movement; (<b>b</b>) comparison of tracking errors for Tester A’s standing hip flexion trajectory; (<b>c</b>) comparison of trajectory tracking curves for Tester B’s standing hip flexion movement; (<b>d</b>) comparison of tracking errors for Tester B’s standing hip flexion trajectory; (<b>e</b>) comparison of trajectory tracking curves for Tester C’s standing hip flexion movement; (<b>f</b>) comparison of tracking errors for Tester C’s standing hip flexion trajectory.</p> Full article ">
<p>Dynamic model of the hip joint.</p> Full article ">Figure 2
<p>Structure of the PID control system.</p> Full article ">Figure 3
<p>Principle of the fuzzy PID controller structure.</p> Full article ">Figure 4
<p>Membership function of input and output variables.</p> Full article ">Figure 5
<p>Fuzzy surfaces of Δk<sub>p</sub>, Δk<sub>i</sub>, and Δk<sub>d</sub>.</p> Full article ">Figure 6
<p>Flowchart of SSA-fuzzy PID parameter optimization.</p> Full article ">Figure 7
<p>Iteration plot of SSA algorithm adaptation degree.</p> Full article ">Figure 8
<p>Parameter variations in the optimized SSA algorithm system. (<b>a</b>) Change in Ke and Kec parameters; (<b>b</b>) change in Ckp, Cki and Ckd parameters.</p> Full article ">Figure 9
<p>Simulation models. (<b>a</b>) Proportional-Integral-Derivative (PID) controller; (<b>b</b>) fuzzy PID controller.</p> Full article ">Figure 10
<p>Simulation model of the Sparrow Search Algorithm (SSA)-based fuzzy PID controller.</p> Full article ">Figure 11
<p>Comparison of hip joint motion trajectory tracking curves during human-level walking using three control methods.</p> Full article ">Figure 12
<p>Comparison of tracking errors in hip joint motion trajectory during human-level walking using three control methods.</p> Full article ">Figure 13
<p>Comparison of trajectory tracking curves for standing hip flexion motion using three control methods.</p> Full article ">Figure 14
<p>Comparison of tracking errors in standing hip flexion trajectory using three control methods.</p> Full article ">Figure 15
<p>Experimental platform for the lower-limb exoskeleton hip robot. (<b>a</b>) Experimental platform; (<b>b</b>) lower-limb exoskeleton hip robot.</p> Full article ">Figure 16
<p>Motion data acquisition process for Testers A, B, and C wearing the lower-limb exoskeleton device. (<b>a</b>) Acquisition of motion data for Testers A, B, and C while walking on level ground; (<b>b</b>) collection of standing hip flexion movement data for Testers A, B, and C.</p> Full article ">Figure 17
<p>Comparison of hip joint trajectories and tracking errors during level walking for Testers A, B, and C under the three control methods. (<b>a</b>) Comparison of hip joint trajectory tracking curves during level walking for Tester A; (<b>b</b>) comparison of hip joint trajectory tracking errors during level walking for Tester A; (<b>c</b>) comparison of hip joint trajectory tracking curves during level walking for Tester B; (<b>d</b>) comparison of hip joint trajectory tracking errors during level walking for Tester B; (<b>e</b>) comparison of hip joint trajectory tracking curves during level walking for Tester C; (<b>f</b>) comparison of hip joint trajectory tracking errors during level walking for Tester C.</p> Full article ">Figure 18
<p>Comparison of the trajectories and tracking errors of standing hip flexion movements for Testers A, B, and C under the three control methods. (<b>a</b>) Comparison of trajectory tracking curves for Tester A’s standing hip flexion movement; (<b>b</b>) comparison of tracking errors for Tester A’s standing hip flexion trajectory; (<b>c</b>) comparison of trajectory tracking curves for Tester B’s standing hip flexion movement; (<b>d</b>) comparison of tracking errors for Tester B’s standing hip flexion trajectory; (<b>e</b>) comparison of trajectory tracking curves for Tester C’s standing hip flexion movement; (<b>f</b>) comparison of tracking errors for Tester C’s standing hip flexion trajectory.</p> Full article ">Figure 18 Cont.
<p>Comparison of the trajectories and tracking errors of standing hip flexion movements for Testers A, B, and C under the three control methods. (<b>a</b>) Comparison of trajectory tracking curves for Tester A’s standing hip flexion movement; (<b>b</b>) comparison of tracking errors for Tester A’s standing hip flexion trajectory; (<b>c</b>) comparison of trajectory tracking curves for Tester B’s standing hip flexion movement; (<b>d</b>) comparison of tracking errors for Tester B’s standing hip flexion trajectory; (<b>e</b>) comparison of trajectory tracking curves for Tester C’s standing hip flexion movement; (<b>f</b>) comparison of tracking errors for Tester C’s standing hip flexion trajectory.</p> Full article ">
Open AccessArticle
Multiple CR Spatiotemporal Compressive Imaging System
by
Xiaowen Hao, Dingaoyu Zhao and Jun Ke
Sensors 2025, 25(5), 1334; https://doi.org/10.3390/s25051334 - 21 Feb 2025
Abstract
Higher spatial and temporal resolutions are two important performance parameters in an imaging system. However, due to hardware limitations, the two resolutions are usually mutually restricted. To meet this challenge, we propose a spatiotemporal compressive imaging (STCI) system to reconstruct high-spatiotemporal-resolution images from
[...] Read more.
Higher spatial and temporal resolutions are two important performance parameters in an imaging system. However, due to hardware limitations, the two resolutions are usually mutually restricted. To meet this challenge, we propose a spatiotemporal compressive imaging (STCI) system to reconstruct high-spatiotemporal-resolution images from low-resolution measurements. For STCI, we also designed a novel reconstruction network for multiple compression ratio (CR). To verify the effectiveness of our method, we implemented simulation and optical experiments, respectively. The experiment results show that our method can effectively reconstruct high-spatiotemporal-resolution target scenes for nine different CRs. With the maximum spatiotemporal CR of 128:1, our method can achieve a reconstruction accuracy of dB.
Full article
(This article belongs to the Special Issue New Trends in Optical Imaging and Sensing Technologies)

Journal Menu
► ▼ Journal Menu-
- Sensors Home
- Aims & Scope
- Editorial Board
- Reviewer Board
- Topical Advisory Panel
- Instructions for Authors
- Special Issues
- Topics
- Sections & Collections
- Article Processing Charge
- Indexing & Archiving
- Editor’s Choice Articles
- Most Cited & Viewed
- Journal Statistics
- Journal History
- Journal Awards
- Society Collaborations
- Conferences
- Editorial Office
Journal Browser
► ▼ Journal Browser-
arrow_forward_ios
Forthcoming issue
arrow_forward_ios Current issue - Vol. 25 (2025)
- Vol. 24 (2024)
- Vol. 23 (2023)
- Vol. 22 (2022)
- Vol. 21 (2021)
- Vol. 20 (2020)
- Vol. 19 (2019)
- Vol. 18 (2018)
- Vol. 17 (2017)
- Vol. 16 (2016)
- Vol. 15 (2015)
- Vol. 14 (2014)
- Vol. 13 (2013)
- Vol. 12 (2012)
- Vol. 11 (2011)
- Vol. 10 (2010)
- Vol. 9 (2009)
- Vol. 8 (2008)
- Vol. 7 (2007)
- Vol. 6 (2006)
- Vol. 5 (2005)
- Vol. 4 (2004)
- Vol. 3 (2003)
- Vol. 2 (2002)
- Vol. 1 (2001)
Highly Accessed Articles
Latest Books
E-Mail Alert
News
Topics
Topic in
Energies, Hydrology, JMSE, Remote Sensing, Water, Sensors
Application of Smart Technologies in Water Resources Management
Topic Editors: Elias Dimitriou, Joaquim SousaDeadline: 28 February 2025
Topic in
Data, Energies, Sensors, Sustainability, Water
Water and Energy Monitoring and Their Nexus
Topic Editors: Lucas Pereira, Hugo Morais, Wolf-Gerrit FrühDeadline: 31 March 2025
Topic in
Applied Sciences, Electronics, Energies, Sci, Sensors, Systems
Technologies and Applications of Data-Driven Anomaly Detection in Energy Systems
Topic Editors: Marco Savino Piscitelli, Alfonso Capozzoli, Antonio Rosato, Cheng FanDeadline: 30 April 2025
Topic in
Applied Sciences, Electronics, Future Internet, Sensors, Smart Cities
Cloud and Edge Computing for Smart Devices
Topic Editors: Mehdi Sookhak, Francesco MoscatoDeadline: 20 May 2025

Conferences
Special Issues
Special Issue in
Sensors
Advances in Fiber Optic Sensors for Energy Applications
Guest Editors: Michael P. Buric, Nageswara R. LalamDeadline: 25 February 2025
Special Issue in
Sensors
Pattern Recognition Applications in Medical Image Analysis
Guest Editor: Ines DominguesDeadline: 25 February 2025
Special Issue in
Sensors
Vehicle-to-Everything (V2X) Communication Networks 2024–2025
Guest Editors: Qiong Wu, Qiang FanDeadline: 25 February 2025
Special Issue in
Sensors
Editorial Board Members’ Collection Series: Application of InSAR Technology in Geodesy, Earthquake, Landslides and Other Disaster Warning
Guest Editors: Guangcai Feng, Yong ZhengDeadline: 25 February 2025
Topical Collections
Topical Collection in
Sensors
Deep Learning in Biomedical Informatics and Healthcare
Collection Editors: Gianluca Borghini, Gianluca Di Flumeri, Nicolina Sciaraffa, Mobyen Uddin Ahmed, Manousos Klados
Topical Collection in
Sensors
Measurements, Instrumentation, Sensing and Simulation Techniques for the Detection of Radiation
Collection Editors: Andrea Malizia, Tzany Kokalova Wheldon
Topical Collection in
Sensors
Topical Advisory Panel Members’ Collection Series: Sensors Technologies for Intelligent Transportation Systems
Collection Editors: Xiao Wang, Jiangchen Li, Karolina Krzykowska-Piotrowska
Topical Collection in
Sensors
Sensors for Gait, Posture, and Health Monitoring
Collection Editor: Thurmon Lockhart