Journal Description
ISPRS International Journal of Geo-Information
ISPRS International Journal of Geo-Information
is an international, peer-reviewed, open access journal on geo-information. The journal is owned by the International Society for Photogrammetry and Remote Sensing (ISPRS) and is published monthly online by MDPI.
- Open Access— free for readers, with article processing charges (APC) paid by authors or their institutions.
- High Visibility: indexed within Scopus, SCIE (Web of Science), GeoRef, PubAg, dblp, Astrophysics Data System, Inspec, and other databases.
- Journal Rank: JCR - Q2 (Remote Sensing) / CiteScore - Q1 (Geography, Planning and Development)
- Rapid Publication: manuscripts are peer-reviewed and a first decision is provided to authors approximately 36.2 days after submission; acceptance to publication is undertaken in 2.7 days (median values for papers published in this journal in the first half of 2024).
- Recognition of Reviewers: reviewers who provide timely, thorough peer-review reports receive vouchers entitling them to a discount on the APC of their next publication in any MDPI journal, in appreciation of the work done.
Impact Factor:
2.8 (2023);
5-Year Impact Factor:
3.0 (2023)
Latest Articles
Efficient and Verifiable Range Query Scheme for Encrypted Geographical Information in Untrusted Cloud Environments
ISPRS Int. J. Geo-Inf. 2024, 13(8), 281; https://doi.org/10.3390/ijgi13080281 (registering DOI) - 11 Aug 2024
Abstract
With the rapid development of geo-positioning technologies, location-based services have become increasingly widespread. In the field of location-based services, range queries on geographical data have emerged as an important research topic, attracting significant attention from academia and industry. In many applications, data owners
[...] Read more.
With the rapid development of geo-positioning technologies, location-based services have become increasingly widespread. In the field of location-based services, range queries on geographical data have emerged as an important research topic, attracting significant attention from academia and industry. In many applications, data owners choose to outsource their geographical data and range query tasks to cloud servers to alleviate the burden of local data storage and computation. However, this outsourcing presents many security challenges. These challenges include adversaries analyzing outsourced geographical data and query requests to obtain privacy information, untrusted cloud servers selectively querying a portion of the outsourced data to conserve computational resources, returning incorrect search results to data users, and even illegally modifying the outsourced geographical data, etc. To address these security concerns and provide reliable services to data owners and data users, this paper proposes an efficient and verifiable range query scheme (EVRQ) for encrypted geographical information in untrusted cloud environments. EVRQ is constructed based on a map region tree, 0–1encoding, hash function, Bloom filter, and cryptographic multiset accumulator. Extensive experimental evaluations demonstrate the efficiency of EVRQ, and a comprehensive analysis confirms the security of EVRQ.
Full article
(This article belongs to the Topic Recent Advances in Security, Privacy, and Trust)
Open AccessArticle
Spatio-Temporal Big Data Collaborative Storage Mechanism Based on Incremental Aggregation Subvector Commitment in On-Chain and Off-Chain Systems
by
Mingjia Han, Xinyi Yang, Huachang Su, Yekang Zhao, Ding Huang and Yongjun Ren
ISPRS Int. J. Geo-Inf. 2024, 13(8), 280; https://doi.org/10.3390/ijgi13080280 (registering DOI) - 10 Aug 2024
Abstract
As mobile internet and Internet of Things technologies rapidly advance, the amount of spatio-temporal big data have surged, and efficient and secure management solutions are urgently needed. Although cloud storage provides convenience, it also brings significant data security challenges. Blockchain technology is an
[...] Read more.
As mobile internet and Internet of Things technologies rapidly advance, the amount of spatio-temporal big data have surged, and efficient and secure management solutions are urgently needed. Although cloud storage provides convenience, it also brings significant data security challenges. Blockchain technology is an ideal choice for processing large-scale spatio-temporal big data due to its unique security features, but its storage scalability is limited because the data need to be replicated throughout the network. To solve this problem, a common approach is to combine blockchain with off-chain storage to form a hybrid storage blockchain. However, these solutions cannot guarantee the authenticity, integrity, and consistency of on-chain and off-chain data storage, and preprocessing is required in the setup phase to generate public parameters proportional to the data length, which increases the computational burden and reduces transmission efficiency. Therefore, this paper proposes a collaborative storage mechanism for spatio-temporal big data based on incremental aggregation sub-vector commitments, which uses vector commitment binding technology to ensure the secure storage of on-chain and off-chain data. By generating public parameters of fixed length, the computational complexity is reduced and the communication efficiency is improved while improving the security of the system. In addition, we design an aggregation proof protocol that integrates aggregation algorithms and smart contracts to improve the efficiency of data query and verification and ensure the consistency and integrity of spatio-temporal big data storage. Finally, simulation experiments verify the correctness and security of the proposed protocol, providing a solid foundation for the blockchain-based spatio-temporal big data storage system.
Full article
(This article belongs to the Topic Recent Advances in Security, Privacy, and Trust)
►▼
Show Figures
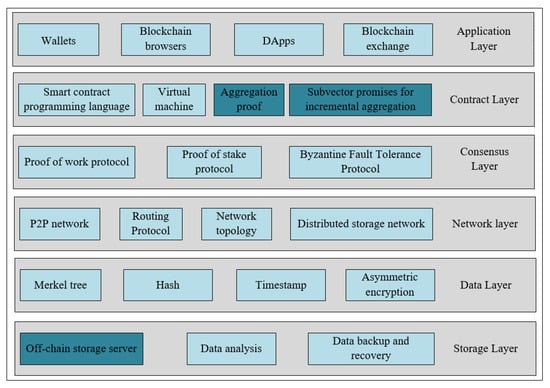
Figure 1
Figure 1
<p>Storage system architecture in on-chain and off-chain system.</p> Full article ">Figure 2
<p>Spatio-temporal big data collaborative storage scheme.</p> Full article ">Figure 3
<p>Comparison result of communication overhead of different schemes.</p> Full article ">Figure 4
<p>Comparison chart of verification and query experimental results.</p> Full article ">Figure 5
<p>Comparison chart of updated experimental results.</p> Full article ">
<p>Storage system architecture in on-chain and off-chain system.</p> Full article ">Figure 2
<p>Spatio-temporal big data collaborative storage scheme.</p> Full article ">Figure 3
<p>Comparison result of communication overhead of different schemes.</p> Full article ">Figure 4
<p>Comparison chart of verification and query experimental results.</p> Full article ">Figure 5
<p>Comparison chart of updated experimental results.</p> Full article ">
Open AccessArticle
Ecological Network Construction Based on Red, Green and Blue Space: A Case Study of Dali City, China
by
Rong Chen, Shunmin Zhang, Xiaoyuan Huang, Xiang Li and Jiansong Peng
ISPRS Int. J. Geo-Inf. 2024, 13(8), 279; https://doi.org/10.3390/ijgi13080279 - 7 Aug 2024
Abstract
Rapid urbanization leads to fragmentation and reduced connectivity of urban landscapes, endangering regional biodiversity conservation and sustainable development. Constructing a red, green, and blue spatial ecological network is an effective way to alleviate ecological pressure and promote economic development. Using circuit theory, hydrological
[...] Read more.
Rapid urbanization leads to fragmentation and reduced connectivity of urban landscapes, endangering regional biodiversity conservation and sustainable development. Constructing a red, green, and blue spatial ecological network is an effective way to alleviate ecological pressure and promote economic development. Using circuit theory, hydrological analysis, and suitability analysis, this study constructs a composite ecological network under urban–rural integration. The results show the following: (1) A total of 22 ecological corridors with a length of 349.20 km, 22 ecological pinch points, and 22 ecological barrier points are identified in the municipal area, mainly distributed in Haidong Town. There are 504 stormwater corridors, which are more evenly distributed, 502 riverfront landscape corridors, and 130 slow-moving landscape corridors. (2) A total of 20 ecological corridors, with a length of 99.23 km, 19 ecological pinch points, and 25 barrier points were identified in the main urban area, and most of them are located in the ecological corridors. There are 71 stormwater corridors, mainly located in the northwestern forest area, 71 riverfront recreation corridors, and 50 slow-moving recreation corridors. (3) Two scales of superimposed ecological source area of 3.65 km2, and eleven ecological corridors, are primarily distributed between Erhai Lake and Xiaguan Town. There are two superimposed stormwater corridors and fourteen recreational corridors. The eco-nodes are mostly distributed in the east and south of Dali City; wetland nodes are mainly situated in the eighteen streams of Cangshan Mountain; and landscape nodes are more balanced in spatial distribution. The study results can provide a reference for composite ecological network construction.
Full article
(This article belongs to the Special Issue Geographic Information Systems and Cartography for a Sustainable World)
►▼
Show Figures
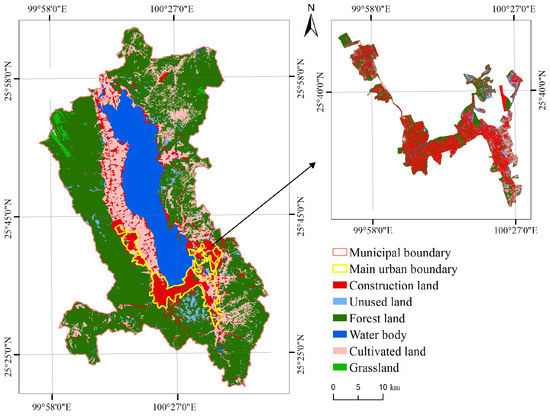
Figure 1
Figure 1
<p>Overview of the study area.</p> Full article ">Figure 2
<p>Research framework. Source–sink theory suggests that landscapes can be categorized as “sources” or “sinks”, with “source” landscapes being those that facilitate processes and “sink” landscapes being those that prevent or retard processes. “Sinks” landscapes are those that prevent or retard the development of processes. Accessibility analysis is an important indicator of the effectiveness of an urban transport system, referring to the ability of residents to reach their destinations within a certain time and cost.</p> Full article ">Figure 3
<p>Spatial distribution of MSPA landscape type and ecological resistance surface. (<b>a</b>) Municipal MSPA landscape type. (<b>b</b>) Municipal ecological resistance surface. (<b>c</b>) Main urban MSPA landscape type. (<b>d</b>) Main urban ecological resistance surface.</p> Full article ">Figure 4
<p>Bubble chart of interaction between ecological source areas based on a gravity model. In the figure, 1–12 represent the ecological source code, and the range of values represents the strength of interaction forces between different ecological sources; the larger the bubble, the stronger the interaction force.</p> Full article ">Figure 5
<p>Eco-node analysis based on Pinchpoint Mapper. (<b>a</b>) Municipal “pairwise” model. (<b>b</b>) Municipal “all to one” model. (<b>c</b>) Main urban “pairwise” model. (<b>d</b>) Main urban “all to one” model.</p> Full article ">Figure 6
<p>Eco-node analysis based on Barrier Mapper. (<b>a</b>) Municipal unselected improvement scores relative to LCD percentage. (<b>b</b>) Municipal improvement scores versus LCD percentage. (<b>c</b>) Main urban unselected improvement scores relative to LCD percentage. (<b>d</b>) Main urban improvement score versus LCD percentage.</p> Full article ">Figure 7
<p>Spatial distribution of ecological corridors and nodes in (<b>a</b>) municipal and (<b>b</b>) main urban areas.</p> Full article ">Figure 8
<p>Spatial distribution of water green network in (<b>a</b>) municipal and (<b>b</b>) main urban areas.</p> Full article ">Figure 9
<p>Suitability assessment and spatial distribution of landscape recreation networks. (<b>a</b>) Municipal suitability assessment. (<b>b</b>) Municipal landscape network. (<b>c</b>) Main urban suitability assessment. (<b>d</b>) Main urban recreation network.</p> Full article ">Figure 10
<p>Percentage of area in land use types with different corridor widths. (<b>a</b>) Width of biological migration corridor in municipal area. (<b>b</b>) Width of biological migration corridor in main urban area. (<b>c</b>) Width of municipal stormwater corridor. (<b>d</b>) Width of main urban stormwater corridor.</p> Full article ">Figure 11
<p>Spatial distribution of red–green–blue ecological networks with nested analysis. (<b>a</b>) Nesting source and ecological corridors. (<b>b</b>) Nesting of pinch points and barrier points. (<b>c</b>) Nesting of source and stormwater corridors. (<b>d</b>) Nesting of wetland nodes. (<b>e</b>) Nesting of recreational nodes. (<b>f</b>) Nesting of landscape and recreational corridors. (<b>g</b>) Municipal complex ecological network. (<b>h</b>) Main urban complex ecological network.</p> Full article ">
<p>Overview of the study area.</p> Full article ">Figure 2
<p>Research framework. Source–sink theory suggests that landscapes can be categorized as “sources” or “sinks”, with “source” landscapes being those that facilitate processes and “sink” landscapes being those that prevent or retard processes. “Sinks” landscapes are those that prevent or retard the development of processes. Accessibility analysis is an important indicator of the effectiveness of an urban transport system, referring to the ability of residents to reach their destinations within a certain time and cost.</p> Full article ">Figure 3
<p>Spatial distribution of MSPA landscape type and ecological resistance surface. (<b>a</b>) Municipal MSPA landscape type. (<b>b</b>) Municipal ecological resistance surface. (<b>c</b>) Main urban MSPA landscape type. (<b>d</b>) Main urban ecological resistance surface.</p> Full article ">Figure 4
<p>Bubble chart of interaction between ecological source areas based on a gravity model. In the figure, 1–12 represent the ecological source code, and the range of values represents the strength of interaction forces between different ecological sources; the larger the bubble, the stronger the interaction force.</p> Full article ">Figure 5
<p>Eco-node analysis based on Pinchpoint Mapper. (<b>a</b>) Municipal “pairwise” model. (<b>b</b>) Municipal “all to one” model. (<b>c</b>) Main urban “pairwise” model. (<b>d</b>) Main urban “all to one” model.</p> Full article ">Figure 6
<p>Eco-node analysis based on Barrier Mapper. (<b>a</b>) Municipal unselected improvement scores relative to LCD percentage. (<b>b</b>) Municipal improvement scores versus LCD percentage. (<b>c</b>) Main urban unselected improvement scores relative to LCD percentage. (<b>d</b>) Main urban improvement score versus LCD percentage.</p> Full article ">Figure 7
<p>Spatial distribution of ecological corridors and nodes in (<b>a</b>) municipal and (<b>b</b>) main urban areas.</p> Full article ">Figure 8
<p>Spatial distribution of water green network in (<b>a</b>) municipal and (<b>b</b>) main urban areas.</p> Full article ">Figure 9
<p>Suitability assessment and spatial distribution of landscape recreation networks. (<b>a</b>) Municipal suitability assessment. (<b>b</b>) Municipal landscape network. (<b>c</b>) Main urban suitability assessment. (<b>d</b>) Main urban recreation network.</p> Full article ">Figure 10
<p>Percentage of area in land use types with different corridor widths. (<b>a</b>) Width of biological migration corridor in municipal area. (<b>b</b>) Width of biological migration corridor in main urban area. (<b>c</b>) Width of municipal stormwater corridor. (<b>d</b>) Width of main urban stormwater corridor.</p> Full article ">Figure 11
<p>Spatial distribution of red–green–blue ecological networks with nested analysis. (<b>a</b>) Nesting source and ecological corridors. (<b>b</b>) Nesting of pinch points and barrier points. (<b>c</b>) Nesting of source and stormwater corridors. (<b>d</b>) Nesting of wetland nodes. (<b>e</b>) Nesting of recreational nodes. (<b>f</b>) Nesting of landscape and recreational corridors. (<b>g</b>) Municipal complex ecological network. (<b>h</b>) Main urban complex ecological network.</p> Full article ">
Open AccessArticle
A Maximal Multimodal Accessibility Equality Model to Optimize the Equality of Healthcare Services
by
Zhuolin Tao, Qianyu Zhong and Yinuo Dang
ISPRS Int. J. Geo-Inf. 2024, 13(8), 278; https://doi.org/10.3390/ijgi13080278 - 7 Aug 2024
Abstract
►▼
Show Figures
The equality of healthcare services has been a focus among researchers and policymakers. The maximal accessibility equality (MAE) model is a widely used location-allocation model for the optimization of the accessibility equality of facilities. However, it might produce biased results due to the
[...] Read more.
The equality of healthcare services has been a focus among researchers and policymakers. The maximal accessibility equality (MAE) model is a widely used location-allocation model for the optimization of the accessibility equality of facilities. However, it might produce biased results due to the overlooking of multiple transport mode options for urban residents. This study develops a maximal multimodal accessibility equality (MMAE) model by incorporating the multimodal two-step floating catchment area (2SFCA) accessibility model. It reflects the multimodal context in cities and aims to maximize the equality of multimodal accessibility. A case study of healthcare facilities in Shenzhen demonstrates that the proposed MMAE model can significantly improve the equality of multimodal accessibility. However, the traditional single-modal MAE model generates unequal multimodal accessibility, which might yield biased planning recommendations in multimodal contexts. The findings highlight the superiority of the MMAE model against the traditional single-modal MAE model in terms of pursuing equal accessibility for all residents. The MMAE model can serve as a scientific tool to support the rational planning of healthcare facilities or other types of public facilities in multimodal contexts.
Full article
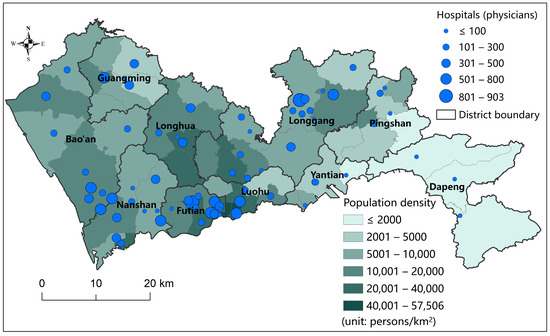
Figure 1
Figure 1
<p>The distribution of the subdistrict-level population density and healthcare facilities.</p> Full article ">Figure 2
<p>The distribution of the actual multimodal accessibility.</p> Full article ">Figure 3
<p>The distribution of optimal healthcare facility sizes and accessibility by MMAE. (<b>a</b>) Overall accessibility; (<b>b</b>) car-modal accessibility; (<b>c</b>) transit-modal accessibility.</p> Full article ">Figure 4
<p>The distribution of (<b>a</b>) the car-modal accessibility and (<b>b</b>) the multimodal accessibility based on optimal healthcare facility sizes by the single car-modal MAE.</p> Full article ">Figure 5
<p>The distribution of (<b>a</b>) the transit-modal accessibility and (<b>b</b>) the multimodal accessibility based on optimal healthcare facility sizes by the single transit-modal transit MAE.</p> Full article ">Figure 6
<p>The distribution of the optimal newly added healthcare resources and multimodal accessibility.</p> Full article ">
<p>The distribution of the subdistrict-level population density and healthcare facilities.</p> Full article ">Figure 2
<p>The distribution of the actual multimodal accessibility.</p> Full article ">Figure 3
<p>The distribution of optimal healthcare facility sizes and accessibility by MMAE. (<b>a</b>) Overall accessibility; (<b>b</b>) car-modal accessibility; (<b>c</b>) transit-modal accessibility.</p> Full article ">Figure 4
<p>The distribution of (<b>a</b>) the car-modal accessibility and (<b>b</b>) the multimodal accessibility based on optimal healthcare facility sizes by the single car-modal MAE.</p> Full article ">Figure 5
<p>The distribution of (<b>a</b>) the transit-modal accessibility and (<b>b</b>) the multimodal accessibility based on optimal healthcare facility sizes by the single transit-modal transit MAE.</p> Full article ">Figure 6
<p>The distribution of the optimal newly added healthcare resources and multimodal accessibility.</p> Full article ">
Open AccessArticle
Influencing Factors of Street Vitality in Historic Districts Based on Multisource Data: Evidence from China
by
Bing Yu, Jing Sun, Zhaoxing Wang and Sanfeng Jin
ISPRS Int. J. Geo-Inf. 2024, 13(8), 277; https://doi.org/10.3390/ijgi13080277 - 5 Aug 2024
Abstract
Amid urban expansion, historic districts face challenges such as declining vitality and deteriorating spatial quality. Using the streets of Xi’an’s historical and cultural district as examples, this research utilizes multisource data, including points of interest (POIs), street view images, and Baidu heatmaps, alongside
[...] Read more.
Amid urban expansion, historic districts face challenges such as declining vitality and deteriorating spatial quality. Using the streets of Xi’an’s historical and cultural district as examples, this research utilizes multisource data, including points of interest (POIs), street view images, and Baidu heatmaps, alongside analytical techniques such as machine learning. This study explores the determinants of street vitality from the dual perspectives of its external manifestation and spatial carriers. A quantitative framework for measuring street vitality in historic districts is established, thoroughly examining the driving factors behind street vitality. Additionally, the relationship between built environment indicators and street vitality is elucidated through statistical analysis methods. The findings reveal significant, time-varying influences of these spatial carriers on human vitality, with distinct spatial distribution patterns of human activity across different times, and the significance of the influence of external representations of human vitality and various types of spatial carriers varies over time. Based on these insights, this paper proposes strategies for enhancing the vitality of historic streets, aiming to rejuvenate and sustain the diverse and dynamic energy of these districts. It provides a foundation for revitalizing the vigor of cultural heritage zones and offers strategies applicable to similar urban contexts.
Full article
(This article belongs to the Special Issue Spatial Information for Improved Living Spaces)
►▼
Show Figures
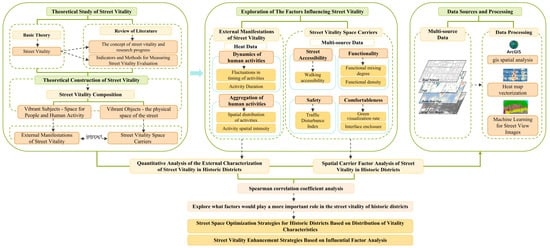
Figure 1
Figure 1
<p>Research framework.</p> Full article ">Figure 2
<p>Study area.</p> Full article ">Figure 3
<p>POIs’ spatial distribution.</p> Full article ">Figure 4
<p>Street vitality evaluation dimensions.</p> Full article ">Figure 5
<p>Spatial distribution characteristics of population aggregation.</p> Full article ">Figure 6
<p>Plot of relative vitality value changes on weekends and weekdays.</p> Full article ">Figure 7
<p>Walking accessibility.</p> Full article ">Figure 8
<p>Functional density.</p> Full article ">Figure 9
<p>The functional mixing degree.</p> Full article ">Figure 10
<p>Green visualization rate.</p> Full article ">Figure 11
<p>Interface enclosure.</p> Full article ">Figure 12
<p>Motorized traffic interference index.</p> Full article ">
<p>Research framework.</p> Full article ">Figure 2
<p>Study area.</p> Full article ">Figure 3
<p>POIs’ spatial distribution.</p> Full article ">Figure 4
<p>Street vitality evaluation dimensions.</p> Full article ">Figure 5
<p>Spatial distribution characteristics of population aggregation.</p> Full article ">Figure 6
<p>Plot of relative vitality value changes on weekends and weekdays.</p> Full article ">Figure 7
<p>Walking accessibility.</p> Full article ">Figure 8
<p>Functional density.</p> Full article ">Figure 9
<p>The functional mixing degree.</p> Full article ">Figure 10
<p>Green visualization rate.</p> Full article ">Figure 11
<p>Interface enclosure.</p> Full article ">Figure 12
<p>Motorized traffic interference index.</p> Full article ">
Open AccessArticle
DCPMS: A Large-Scale Raster Layer Serving Method for Custom Online Calculation and Rendering
by
Anbang Yang, Feng Zhang, Jie Feng, Luoqi Wang, Enjiang Yue, Xinhua Fan, Jingyi Zhang, Linshu Hu and Sensen Wu
ISPRS Int. J. Geo-Inf. 2024, 13(8), 276; https://doi.org/10.3390/ijgi13080276 - 1 Aug 2024
Abstract
Raster data represent one of the fundamental data formats utilized in GIS. As the technology used to observe the Earth continues to evolve, the spatial and temporal resolution of raster data is becoming increasingly refined, while the data scale is expanding. One of
[...] Read more.
Raster data represent one of the fundamental data formats utilized in GIS. As the technology used to observe the Earth continues to evolve, the spatial and temporal resolution of raster data is becoming increasingly refined, while the data scale is expanding. One of the key issues in the development of GIS technology is to determine how to make large-scale raster data better to provide computation, visualization, and analysis services in the Internet environment. This paper proposes a decentralized COG-pyramid-based map service method (DCPMS). In comparison to traditional raster data online service technology, such as GIS servers and static tiles, DCPMS employs virtual mapping to reduce data storage costs and combines tile technology with a cloud-native storage scheme to enhance the concurrency of supportable requests. Furthermore, the band calculation process is shifted to the client, thereby effectively resolving the issue of efficient customized band calculation and data rendering in the context of a large-scale raster data online service. The results indicate DCPMS delivers commendable performance. Its decentralized architecture significantly enhances performance in high concurrency scenarios. With a thousand concurrent requests, the response time of DCPMS is reduced by 74% compared to the GIS server. Moreover, this service exhibits considerable strengths in data preprocessing and storage, suggesting a novel pathway for future technical improvement of large-scale raster data map services.
Full article
(This article belongs to the Topic Remote Sensing and Visualization Methods: Monitoring, Modeling, Simulations and Interaction of Forest Resource)
►▼
Show Figures
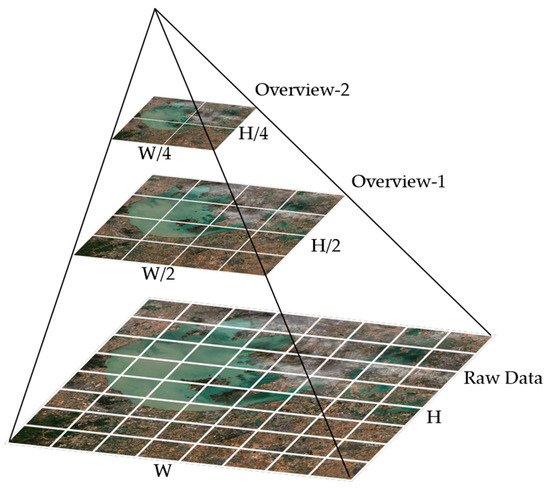
Figure 1
Figure 1
<p>Schematic diagram of COG organization.</p> Full article ">Figure 2
<p>Technology roadmap of DCPMS.</p> Full article ">Figure 3
<p>Schematic diagram of COG-pyramid model. <math display="inline"><semantics> <mrow> <msub> <mrow> <mi>n</mi> </mrow> <mrow> <mn>1</mn> </mrow> </msub> </mrow> </semantics></math> and <math display="inline"><semantics> <mrow> <msub> <mrow> <mi>n</mi> </mrow> <mrow> <mn>2</mn> </mrow> </msub> </mrow> </semantics></math> can be identical or different.</p> Full article ">Figure 4
<p>Data interaction diagram of DCPMS.</p> Full article ">Figure 5
<p>COG-pyramid data processing flow chart.</p> Full article ">Figure 6
<p>RGB rendering of Sentinel-2 image dataset.</p> Full article ">Figure 7
<p>NDVI band calculation and rendering of Sentinel-2 image dataset.</p> Full article ">Figure 8
<p>Comparison of average loading time of clients in different raster layer serving methods.</p> Full article ">Figure 9
<p>Comparison of response time under different concurrency.</p> Full article ">Figure 10
<p>Unique value rendering of WorldCover 2021 dataset.</p> Full article ">Figure 11
<p>Comparison of processing time and storage space of static tiles and DCPMS.</p> Full article ">
<p>Schematic diagram of COG organization.</p> Full article ">Figure 2
<p>Technology roadmap of DCPMS.</p> Full article ">Figure 3
<p>Schematic diagram of COG-pyramid model. <math display="inline"><semantics> <mrow> <msub> <mrow> <mi>n</mi> </mrow> <mrow> <mn>1</mn> </mrow> </msub> </mrow> </semantics></math> and <math display="inline"><semantics> <mrow> <msub> <mrow> <mi>n</mi> </mrow> <mrow> <mn>2</mn> </mrow> </msub> </mrow> </semantics></math> can be identical or different.</p> Full article ">Figure 4
<p>Data interaction diagram of DCPMS.</p> Full article ">Figure 5
<p>COG-pyramid data processing flow chart.</p> Full article ">Figure 6
<p>RGB rendering of Sentinel-2 image dataset.</p> Full article ">Figure 7
<p>NDVI band calculation and rendering of Sentinel-2 image dataset.</p> Full article ">Figure 8
<p>Comparison of average loading time of clients in different raster layer serving methods.</p> Full article ">Figure 9
<p>Comparison of response time under different concurrency.</p> Full article ">Figure 10
<p>Unique value rendering of WorldCover 2021 dataset.</p> Full article ">Figure 11
<p>Comparison of processing time and storage space of static tiles and DCPMS.</p> Full article ">
Open AccessArticle
Factors Influencing the Efficiency of Demand-Responsive Transport Services in Rural Areas: A GIS-Based Method for Optimising and Evaluating Potential Services
by
Carlos Tejero-Beteta, Amparo Moyano and Santos Sánchez-Cambronero
ISPRS Int. J. Geo-Inf. 2024, 13(8), 275; https://doi.org/10.3390/ijgi13080275 - 1 Aug 2024
Abstract
Demand-responsive transport (DRT) could be an alternative for extending the accessibility of high-speed rail (HSR) servicing cities in rural environments, where fixed public transport does not provide efficient services. This paper proposes a method to analyse the factors that influence the implementation of
[...] Read more.
Demand-responsive transport (DRT) could be an alternative for extending the accessibility of high-speed rail (HSR) servicing cities in rural environments, where fixed public transport does not provide efficient services. This paper proposes a method to analyse the factors that influence the implementation of DRT systems for inter-urban mobility, connecting and integrating towns in rural areas. Methodologically, a vehicle routing problem analysis in a GIS-based environment is applied to a theoretical case study to evaluate the factors that influence DRT efficiency in different scenarios, considering the specific singularities of this kind of inter-urban long-distance mobility. The results suggest the optimal DRT solutions in these rural contexts to be those that, after adjusting the fleet to specific demands, use low-capacity vehicles, which are much better adapted to the geography of sparsely populated areas. Moreover, in adapting DRT systems to HSR travellers’ needs, windows catering to these needs should incorporate the option of setting the pickup or arrival times. This paper demonstrates that DRT systems could reach significant levels of service in rural areas compared with fixed lines and even private vehicles, especially when evaluating key aspects of the system’s efficiency for its implementation.
Full article
(This article belongs to the Special Issue Application of Geographical Information System in Urban Design, Management or Evaluation)
►▼
Show Figures

Figure 1
Figure 1
<p>Steps of the method proposed.</p> Full article ">Figure 2
<p>Toy network.</p> Full article ">Figure 3
<p>Description of the steps of the proposed method.</p> Full article ">Figure 4
<p>Routes’ optimal solution for scenario E_02_2C4. (<b>a</b>) Route 1. (<b>b</b>) Route 2.</p> Full article ">Figure 5
<p>(<b>a</b>) Routes’ optimal solution for scenario E_02_2C4. (<b>b</b>) Satisfied demand trip directly. (<b>c</b>) Non-satisfied demand trip. (<b>d</b>) Satisfied demand trips, travelling together with other demand trip.</p> Full article ">Figure 6
<p>P score for scenarios of 12-trip matrices.</p> Full article ">Figure 7
<p>P score for scenarios of 25-trip matrices.</p> Full article ">Figure 8
<p>P score for scenarios of 50-trip matrices.</p> Full article ">
<p>Steps of the method proposed.</p> Full article ">Figure 2
<p>Toy network.</p> Full article ">Figure 3
<p>Description of the steps of the proposed method.</p> Full article ">Figure 4
<p>Routes’ optimal solution for scenario E_02_2C4. (<b>a</b>) Route 1. (<b>b</b>) Route 2.</p> Full article ">Figure 5
<p>(<b>a</b>) Routes’ optimal solution for scenario E_02_2C4. (<b>b</b>) Satisfied demand trip directly. (<b>c</b>) Non-satisfied demand trip. (<b>d</b>) Satisfied demand trips, travelling together with other demand trip.</p> Full article ">Figure 6
<p>P score for scenarios of 12-trip matrices.</p> Full article ">Figure 7
<p>P score for scenarios of 25-trip matrices.</p> Full article ">Figure 8
<p>P score for scenarios of 50-trip matrices.</p> Full article ">
Open AccessArticle
BS-GeoEduNet 1.0: Blockchain-Assisted Serverless Framework for Geospatial Educational Information Networks
by
Meenakshi Kandpal, Veena Goswami, Yash Pritwani, Rabindra K. Barik and Manob Jyoti Saikia
ISPRS Int. J. Geo-Inf. 2024, 13(8), 274; https://doi.org/10.3390/ijgi13080274 - 1 Aug 2024
Abstract
►▼
Show Figures
The integration of a blockchain-supported serverless computing framework enhances the performance of computational and analytical operations and the provision of services within internet-based data centers, rather than depending on independent desktop computers. Therefore, in the present research paper, a blockchain-assisted serverless framework for
[...] Read more.
The integration of a blockchain-supported serverless computing framework enhances the performance of computational and analytical operations and the provision of services within internet-based data centers, rather than depending on independent desktop computers. Therefore, in the present research paper, a blockchain-assisted serverless framework for geospatial data visualizations is implemented. The proposed BS-GeoEduNet 1.0 framework leverages the capabilities of AWS Lambda for serverless computing, providing a reliable and efficient solution for data storage, analysis, and distribution. The proposed framework incorporates AES encryption, decryption layers, and queue implementation to achieve a scalable approach for handling larger files. It implements a queueing mechanism during the heavier input/output processes of file processing by using Apache KAFKA, enabling the system to handle large volumes of data efficiently. It concludes with the visualization of all geospatial-enabled NIT/IIT details on the proposed framework, which utilizes the data fetched from MongoDB. The experimental findings validate the reliability and efficiency of the proposed system, demonstrating its efficacy in geospatial data storage and processing.
Full article
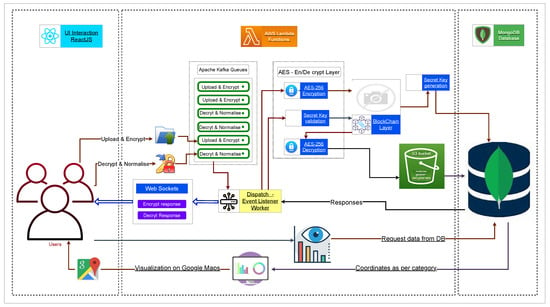
Figure 1
Figure 1
<p>Proposed framework: <span class="html-italic">BS-GeoEduNet 1.0</span>.</p> Full article ">Figure 2
<p>Layered structure of the blockchain architecture.</p> Full article ">Figure 3
<p>Queueing analytical approach for the proposed framework.</p> Full article ">Figure 4
<p>Utilization factor per processor vs. normalized waiting time.</p> Full article ">Figure 5
<p>Utilization factor per processor vs. fraction of processor busy time.</p> Full article ">Figure 6
<p>Utilization factor per processor vs. fraction of requests lost.</p> Full article ">Figure 7
<p><math display="inline"><semantics> <mi>φ</mi> </semantics></math> vs. processor vs. <math display="inline"><semantics> <msub> <mi>W</mi> <mi>s</mi> </msub> </semantics></math>.</p> Full article ">Figure 8
<p><math display="inline"><semantics> <mi>φ</mi> </semantics></math> vs. processor vs. <math display="inline"><semantics> <mrow> <mi>E</mi> <mo>(</mo> <mi>B</mi> <mo>)</mo> </mrow> </semantics></math>.</p> Full article ">Figure 9
<p>Sequence diagram for functionality 1: User uploads CSV and data processing. This diagram illustrates the steps involved in the user uploading a CSV file and the subsequent data processing steps within the system.</p> Full article ">Figure 10
<p>Sequence diagram for functionality 2: user decryption and normalization.</p> Full article ">Figure 11
<p>Sequence diagram for functionality 3: data visualization.</p> Full article ">Figure 12
<p>Sequence diagram for functionality of <span class="html-italic">BS-GeoEduNet 1.0</span>.</p> Full article ">Figure 13
<p>Geospatial data visualizations show the total number of IITs in India with the help of <span class="html-italic">BS-GeoEduNet 1.0</span>.</p> Full article ">
<p>Proposed framework: <span class="html-italic">BS-GeoEduNet 1.0</span>.</p> Full article ">Figure 2
<p>Layered structure of the blockchain architecture.</p> Full article ">Figure 3
<p>Queueing analytical approach for the proposed framework.</p> Full article ">Figure 4
<p>Utilization factor per processor vs. normalized waiting time.</p> Full article ">Figure 5
<p>Utilization factor per processor vs. fraction of processor busy time.</p> Full article ">Figure 6
<p>Utilization factor per processor vs. fraction of requests lost.</p> Full article ">Figure 7
<p><math display="inline"><semantics> <mi>φ</mi> </semantics></math> vs. processor vs. <math display="inline"><semantics> <msub> <mi>W</mi> <mi>s</mi> </msub> </semantics></math>.</p> Full article ">Figure 8
<p><math display="inline"><semantics> <mi>φ</mi> </semantics></math> vs. processor vs. <math display="inline"><semantics> <mrow> <mi>E</mi> <mo>(</mo> <mi>B</mi> <mo>)</mo> </mrow> </semantics></math>.</p> Full article ">Figure 9
<p>Sequence diagram for functionality 1: User uploads CSV and data processing. This diagram illustrates the steps involved in the user uploading a CSV file and the subsequent data processing steps within the system.</p> Full article ">Figure 10
<p>Sequence diagram for functionality 2: user decryption and normalization.</p> Full article ">Figure 11
<p>Sequence diagram for functionality 3: data visualization.</p> Full article ">Figure 12
<p>Sequence diagram for functionality of <span class="html-italic">BS-GeoEduNet 1.0</span>.</p> Full article ">Figure 13
<p>Geospatial data visualizations show the total number of IITs in India with the help of <span class="html-italic">BS-GeoEduNet 1.0</span>.</p> Full article ">
Open AccessArticle
The Influence of Proximity on the Evolution of Urban Innovation Networks in Nanjing Metropolitan Area, China: A Comparative Analysis of Knowledge and Technological Innovations
by
Yu Shi, Wei Zhai, Yiran Yan and Xingping Wang
ISPRS Int. J. Geo-Inf. 2024, 13(8), 273; https://doi.org/10.3390/ijgi13080273 - 1 Aug 2024
Abstract
►▼
Show Figures
This study investigates the dynamics of innovation element flows among metropolitan areas and examines the underlying proximity mechanisms that are crucial for elevating urban agglomerations’ innovation levels and spurring their development. Utilizing collaborative publication and patent data, this research constructs knowledge and technological
[...] Read more.
This study investigates the dynamics of innovation element flows among metropolitan areas and examines the underlying proximity mechanisms that are crucial for elevating urban agglomerations’ innovation levels and spurring their development. Utilizing collaborative publication and patent data, this research constructs knowledge and technological innovation networks within the Nanjing metropolitan area (NMA) from 2013 to 2020. It analyzes the evolution of network structures and applies the Multiple Regression Quadratic Assignment Procedure to discern the proximity mechanisms driving the urban innovation networks’ evolution in NMA. The main findings are as follows: (1) The knowledge collaborations within NMA cities remain largely confined to cities within Jiangsu province, whereas the technological collaborations are shifting from intra-province to cross-province cooperation. (2) Both knowledge and technological innovation networks display a “core-periphery” configuration, with Nanjing maintaining a dominant central position. The scale of the KIN surpasses that of the TIN, while the latter’s growth rate outpaces the former’s. Technological collaborations demonstrate more pronounced spillover effects than their knowledge counterparts. (3) At the metropolitan area level, organizational, social, cognitive, and technological proximities exert varying degrees of influence on innovation cooperation among different innovation entities across various years. Cognitive proximity exhibits the most substantial explanatory power. Based on these findings, the study proposes relevant policy recommendations for constructing an innovative NMA and promoting collaborative innovation development among cities within the NMA.
Full article
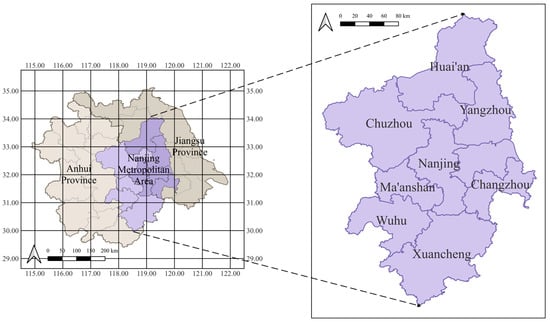
Figure 1
Figure 1
<p>Location of study area.</p> Full article ">Figure 2
<p>Analysis steps.</p> Full article ">Figure 3
<p>Selection of variables for proximity influence factors.</p> Full article ">Figure 4
<p>Number and growth rate of co-published papers in the NMA.</p> Full article ">Figure 5
<p>Composition of urban KIN in the NMA, 2013 (<b>a</b>) versus 2020 (<b>b</b>).</p> Full article ">Figure 6
<p>The structure of the urban knowledge collaboration network in the NMA, 2013 (<b>a</b>) compared to 2020 (<b>b</b>).</p> Full article ">Figure 7
<p>Composition of urban TIN in the NMA, 2013 (<b>a</b>) versus 2020 (<b>b</b>).</p> Full article ">Figure 8
<p>The structure of the urban TIN in the NMA, 2013 (<b>a</b>) compared to 2020 (<b>b</b>).</p> Full article ">Figure 9
<p>Innovation entities’ collaborative network topological diagram in the NMA, 2013–2020.</p> Full article ">
<p>Location of study area.</p> Full article ">Figure 2
<p>Analysis steps.</p> Full article ">Figure 3
<p>Selection of variables for proximity influence factors.</p> Full article ">Figure 4
<p>Number and growth rate of co-published papers in the NMA.</p> Full article ">Figure 5
<p>Composition of urban KIN in the NMA, 2013 (<b>a</b>) versus 2020 (<b>b</b>).</p> Full article ">Figure 6
<p>The structure of the urban knowledge collaboration network in the NMA, 2013 (<b>a</b>) compared to 2020 (<b>b</b>).</p> Full article ">Figure 7
<p>Composition of urban TIN in the NMA, 2013 (<b>a</b>) versus 2020 (<b>b</b>).</p> Full article ">Figure 8
<p>The structure of the urban TIN in the NMA, 2013 (<b>a</b>) compared to 2020 (<b>b</b>).</p> Full article ">Figure 9
<p>Innovation entities’ collaborative network topological diagram in the NMA, 2013–2020.</p> Full article ">
Open AccessArticle
Analysis of Road Safety Perception and Influencing Factors in a Complex Urban Environment—Taking Chaoyang District, Beijing, as an Example
by
Xinyu Hou and Peng Chen
ISPRS Int. J. Geo-Inf. 2024, 13(8), 272; https://doi.org/10.3390/ijgi13080272 - 31 Jul 2024
Abstract
Measuring human perception of environmental safety and quantifying the street view elements that affect human perception of environmental safety are of great significance for improving the urban environment and residents’ safety perception. However, domestic large-scale quantitative research on the safety perception of Chinese
[...] Read more.
Measuring human perception of environmental safety and quantifying the street view elements that affect human perception of environmental safety are of great significance for improving the urban environment and residents’ safety perception. However, domestic large-scale quantitative research on the safety perception of Chinese local cities needs to be deepened. Therefore, this paper chooses Chaoyang District in Beijing as the research area. Firstly, the network safety perception distribution of Chaoyang District is calculated and presented through the CNN model trained based on the perception dataset constructed by Chinese local cities. Then, the street view elements are extracted from the street view images using image semantic segmentation and target detection technology. Finally, the street view elements that affect the road safety perception are identified and analyzed based on LightGBM and SHAP interpretation framework. The results show the following: (1) the overall safety perception level of Chaoyang District in Beijing is high; (2) the number of motor vehicles and the proportion of the area of roads, skies, and sidewalks are the four factors that have the greatest impact on environmental safety perception; (3) there is an interaction between different street view elements on safety perception, and the proportion and number of street view elements have interaction on safety perception; (4) in the sections with the lowest, moderate, and highest levels of safety perception, the influence of street view elements on safety perception is inconsistent. Finally, this paper summarizes the results and points out the shortcomings of the research.
Full article
(This article belongs to the Special Issue Application of Geographical Information System in Urban Design, Management or Evaluation)
►▼
Show Figures
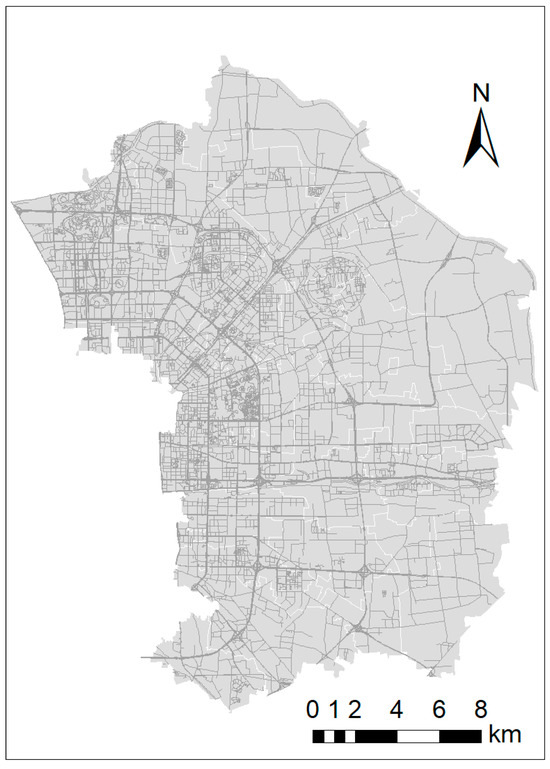
Figure 1
Figure 1
<p>Research area and road network structure. The white line is the boundary of each street, and the gray line is the road network.</p> Full article ">Figure 2
<p>Azimuth angles of four street view images of sample points.</p> Full article ">Figure 3
<p>The process of obtaining Google Street View images.</p> Full article ">Figure 4
<p>Process of safety perception scoring for street view images.</p> Full article ">Figure 5
<p>Extraction of street view elements. The red box indicates how to extract the number of street view elements through object detection.</p> Full article ">Figure 6
<p>Distribution of safety perception.</p> Full article ">Figure 7
<p>Impact of street view elements on environmental safety perception.</p> Full article ">Figure 8
<p>Impact of explanatory variables on safety perception.</p> Full article ">Figure 8 Cont.
<p>Impact of explanatory variables on safety perception.</p> Full article ">Figure 9
<p>The interactive effect of bivariate on SHAP values.</p> Full article ">Figure 10
<p>The interactive impact of street view elements on safety perception.</p> Full article ">Figure 10 Cont.
<p>The interactive impact of street view elements on safety perception.</p> Full article ">Figure 11
<p>Interactive influence of the number and proportion of street view elements.</p> Full article ">Figure 12
<p>The place with the lowest environmental safety perception. (<b>a</b>) Local SHAP value. (<b>b</b>) The example Street View Image.</p> Full article ">Figure 13
<p>The place with the moderate environmental safety perception. (<b>a</b>) Local SHAP value. (<b>b</b>) The example Street View Image.</p> Full article ">Figure 13 Cont.
<p>The place with the moderate environmental safety perception. (<b>a</b>) Local SHAP value. (<b>b</b>) The example Street View Image.</p> Full article ">Figure 14
<p>The place with the highest environmental safety perception. (<b>a</b>) Local SHAP value. (<b>b</b>) The example Street View Image.</p> Full article ">
<p>Research area and road network structure. The white line is the boundary of each street, and the gray line is the road network.</p> Full article ">Figure 2
<p>Azimuth angles of four street view images of sample points.</p> Full article ">Figure 3
<p>The process of obtaining Google Street View images.</p> Full article ">Figure 4
<p>Process of safety perception scoring for street view images.</p> Full article ">Figure 5
<p>Extraction of street view elements. The red box indicates how to extract the number of street view elements through object detection.</p> Full article ">Figure 6
<p>Distribution of safety perception.</p> Full article ">Figure 7
<p>Impact of street view elements on environmental safety perception.</p> Full article ">Figure 8
<p>Impact of explanatory variables on safety perception.</p> Full article ">Figure 8 Cont.
<p>Impact of explanatory variables on safety perception.</p> Full article ">Figure 9
<p>The interactive effect of bivariate on SHAP values.</p> Full article ">Figure 10
<p>The interactive impact of street view elements on safety perception.</p> Full article ">Figure 10 Cont.
<p>The interactive impact of street view elements on safety perception.</p> Full article ">Figure 11
<p>Interactive influence of the number and proportion of street view elements.</p> Full article ">Figure 12
<p>The place with the lowest environmental safety perception. (<b>a</b>) Local SHAP value. (<b>b</b>) The example Street View Image.</p> Full article ">Figure 13
<p>The place with the moderate environmental safety perception. (<b>a</b>) Local SHAP value. (<b>b</b>) The example Street View Image.</p> Full article ">Figure 13 Cont.
<p>The place with the moderate environmental safety perception. (<b>a</b>) Local SHAP value. (<b>b</b>) The example Street View Image.</p> Full article ">Figure 14
<p>The place with the highest environmental safety perception. (<b>a</b>) Local SHAP value. (<b>b</b>) The example Street View Image.</p> Full article ">
Open AccessArticle
A Spatial Case-Based Reasoning Method for Healthy City Assessment: A Case Study of Middle Layer Super Output Areas (MSOAs) in Birmingham, England
by
Shuguang Deng, Wei Liu, Ying Peng and Binglin Liu
ISPRS Int. J. Geo-Inf. 2024, 13(8), 271; https://doi.org/10.3390/ijgi13080271 - 31 Jul 2024
Abstract
►▼
Show Figures
Assessing healthy cities is a crucial strategy for realizing the concept of “health in all policies”. However, most current quantitative assessment methods for healthy cities are predominantly city-level and often overlook intra-urban evaluations. Building on the concept of geographic spatial case-based reasoning (CBR),
[...] Read more.
Assessing healthy cities is a crucial strategy for realizing the concept of “health in all policies”. However, most current quantitative assessment methods for healthy cities are predominantly city-level and often overlook intra-urban evaluations. Building on the concept of geographic spatial case-based reasoning (CBR), we present an innovative healthy city spatial case-based reasoning (HCSCBR) model. This model comprehensively integrates spatial relationships and attribute characteristics that impact urban health. We conducted experiments using a detailed multi-source dataset of health environment determinants for middle-layer super output areas (MSOAs) in Birmingham, England. The results demonstrate that our method surpasses traditional data mining techniques in classification performance, offering greater accuracy and efficiency than conventional CBR models. The flexibility of this method permits its application not only in intra-city health evaluations but also in extending to inter-city assessments. Our research concludes that the HCSCBR model significantly improves the precision and reliability of healthy city assessments by incorporating spatial relationships. Additionally, the model’s adaptability and efficiency render it a valuable tool for urban planners and public health researchers. Future research will focus on integrating the temporal dimension to further enhance and refine the healthy city evaluation model, thereby increasing its dynamism and predictive accuracy.
Full article
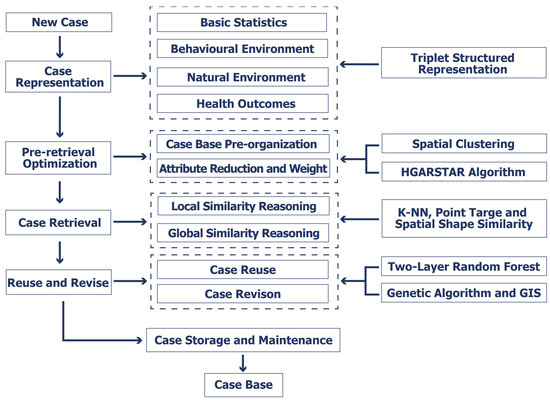
Figure 1
Figure 1
<p>Research Framework of HCSCBR.</p> Full article ">Figure 2
<p>Birmingham, England’s 135 MSOAs.</p> Full article ">Figure 3
<p>Pre-organization of the case: (<b>a</b>) 29 cities in England generate 3 clusters; (<b>b</b>) 135 MSOAs in Birmingham generate 5 clusters.</p> Full article ">Figure 4
<p>Error comparison of different CBR methods through 10-fold cross-validation.</p> Full article ">
<p>Research Framework of HCSCBR.</p> Full article ">Figure 2
<p>Birmingham, England’s 135 MSOAs.</p> Full article ">Figure 3
<p>Pre-organization of the case: (<b>a</b>) 29 cities in England generate 3 clusters; (<b>b</b>) 135 MSOAs in Birmingham generate 5 clusters.</p> Full article ">Figure 4
<p>Error comparison of different CBR methods through 10-fold cross-validation.</p> Full article ">
Open AccessArticle
Challenges to Viticulture in Montenegro under Climate Change
by
António Fernandes, Nataša Kovač, Hélder Fraga, André Fonseca, Sanja Šućur Radonjić, Marko Simeunović, Kruna Ratković, Christoph Menz, Sergi Costafreda-Aumedes and João A. Santos
ISPRS Int. J. Geo-Inf. 2024, 13(8), 270; https://doi.org/10.3390/ijgi13080270 - 30 Jul 2024
Abstract
The Montenegrin climate is characterised as very heterogeneous due to its complex topography. The viticultural heritage, dating back to before the Roman empire, is settled in a Mediterranean climate region, located south of the capital Podgorica, where climate conditions favour red wine production.
[...] Read more.
The Montenegrin climate is characterised as very heterogeneous due to its complex topography. The viticultural heritage, dating back to before the Roman empire, is settled in a Mediterranean climate region, located south of the capital Podgorica, where climate conditions favour red wine production. However, an overall increase in warmer and drier periods affects traditional viticulture. The present study aims to discuss climate change impacts on Montenegrin viticulture. Bioclimatic indices, ensembled from five climate models, were analysed for both historical (1981–2010) and future (2041–2070) periods upon three socio-economic pathways: SSP1-2.6, SSP3-7.0 and SSP5-8.5. CHELSA (≈1 km) was the selected dataset for this analysis. Obtained results for all scenarios have shown the suppression of baseline conditions for viticulture. The average summer temperature might reach around 29.5 °C, and the growing season average temperature could become higher than 23.5 °C, advancing phenological events. The Winkler index is estimated to range from 2900 °C up to 3100 °C, which is too hot for viticulture. Montenegrin viticulture requires the application of adaptation measures focused on reducing temperature-increase impacts. The implementation of adaptation measures shall start in the coming years, to assure the lasting productivity and sustainability of viticulture.
Full article
(This article belongs to the Topic Climate Change Impacts and Adaptation: Interdisciplinary Perspectives)
►▼
Show Figures
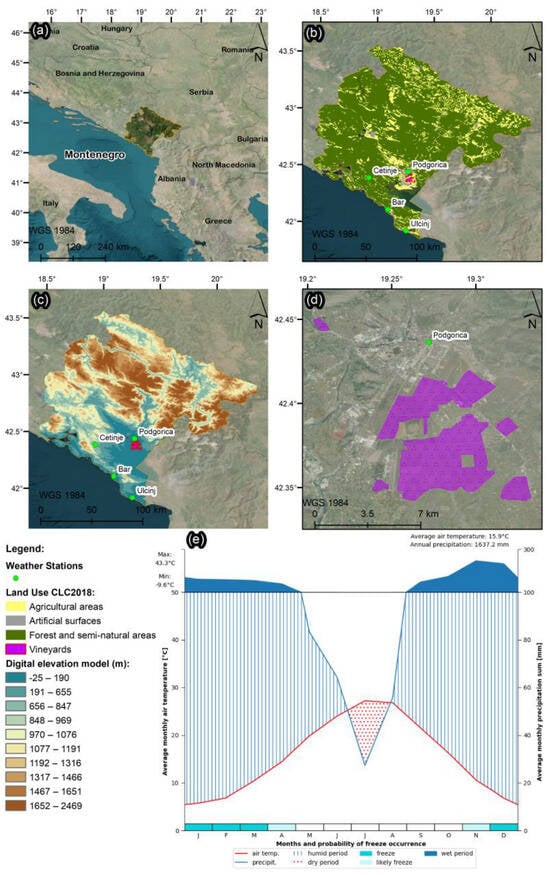
Figure 1
Figure 1
<p>Representation of the Study Area. (<b>a</b>) is the location of Montenegro and contiguous countries. (<b>b</b>) is the location of Montenegro vineyards and other land uses, according to CLC 2018, and the location of weather stations. (<b>c</b>) portrays the elevation of Montenegro, in 10 quantiles. In (<b>d</b>), the vineyards of Montenegro near Podgorica’s weather station are shown. In (<b>e</b>), an ombrothermic diagram of Podgorica weather station data (cf. legend for details) during the Historical period (1981–2010) is depicted.</p> Full article ">Figure 2
<p>Representation of the climate average of daily average temperature during the growing season (from April to October), for the Historical period (1981–2010), for CHELSA (<b>a</b>) with a resolution of 0.008°, E-OBS (<b>b</b>) with a resolution of 0.1°, and ERA5-Land (<b>c</b>) with a resolution of 0.1°.</p> Full article ">Figure 3
<p>Representation of the climate annual precipitation during the growing season (from April to October), for the Historical period (1981–2010), for CHELSA (<b>a</b>) with a resolution of 0.008°, E-OBS (<b>b</b>) with a resolution of 0.1°, and ERA5-Land (<b>c</b>) with a resolution of 0.1°.</p> Full article ">Figure 4
<p>Climate mean daily average temperature during the vineyard growing season from April to October, for Montenegro, according to the CHELSA dataset (<b>a</b>) over the historical period (1981–2010) and the future period (2041–2070) under (<b>b</b>) SSP1-2.6, (<b>c</b>) SSP3-7.0, and (<b>d</b>) SSP5-8.5. (<b>e</b>) Corresponding Kernel density plot of the spatial variability in (<b>a</b>–<b>d</b>). The median value in the vineyard area is shown as a vertical dashed line. Projections were ensembled from 5 climate models through the median.</p> Full article ">Figure 5
<p>Climate mean daily average temperature during the warmest quarter for Montenegro according to the CHELSA dataset (<b>a</b>) over the historical period (1981–2010) and the future period (2041–2070) under (<b>b</b>) SSP1-2.6, (<b>c</b>) SSP3-7.0, and (<b>d</b>) SSP5-8.5. (<b>e</b>) Corresponding Kernel density plot of the spatial variability in (<b>a</b>–<b>d</b>). The median value in the vineyard area is shown as a vertical dashed line. Projections were estimated by the ensemble median from 5 climate models.</p> Full article ">Figure 6
<p>Climate mean of temperature seasonality; the standard deviation of the monthly means for Montenegro according to the CHELSA dataset (<b>a</b>) over the historical period (1981–2010) and the future period (2041–2070) under (<b>b</b>) SSP1-2.6, (<b>c</b>) SSP3-7.0 and (<b>d</b>) SSP5-8.5. (<b>e</b>) Corresponding Kernel density plot of the spatial variability in (<b>a</b>–<b>d</b>). The median value in the vineyard area is shown as a vertical dashed line. Projections were estimated by the ensemble median from 5 climate models.</p> Full article ">Figure 7
<p>Climate mean growing degree days heat sum above 10 °C for Montenegro according to the CHELSA dataset (<b>a</b>) over the historical period (1981–2010) and the future period (2041–2070) under (<b>b</b>) SSP1-2.6, (<b>c</b>) SSP3-7.0 and (<b>d</b>) SSP5-8.5. (<b>e</b>) Corresponding Kernel density plot of the spatial variability in (<b>a</b>–<b>d</b>). The median value in the vineyard area is shown as a vertical dashed line. Projections were estimated by the ensemble median from 5 climate models.</p> Full article ">Figure 8
<p>Climate annual mean of Winkler index for Montenegro according to the CHELSA dataset (<b>a</b>) over the historical period (1981–2010) and the future period (2041–2070) under (<b>b</b>) SSP1-2.6, (<b>c</b>) SSP3-7.0 and (<b>d</b>) SSP5-8.5. (<b>e</b>) Corresponding Kernel density plot of the spatial variability in (<b>a</b>–<b>d</b>). The median value in the vineyard area is shown as a vertical dashed line. Projections were estimated by the ensemble median from 5 climate models.</p> Full article ">Figure 9
<p>Climate mean of growing degree days with an average daily temperature higher than 10 °C for Montenegro, according to the CHELSA dataset (<b>a</b>) over the historical period (1981–2010) and the future period (2041–2070), under (<b>b</b>) SSP1-2.6, (<b>c</b>) SSP3-7.0 and (<b>d</b>) SSP5-8.5. (<b>e</b>) Corresponding Kernel density plot of the spatial variability in (<b>a</b>–<b>d</b>). The median value in the vineyard area is shown as a vertical dashed line. Projections were estimated by the ensemble median from 5 climate models.</p> Full article ">Figure 10
<p>Chronograms of the annual averages of the outlined indices for Podgorica weather station during the historical period (1981–2010). (<b>a</b>) Daily mean temperature during the vineyard growing season, from April to October. (<b>b</b>) Daily mean temperature during the warmest quarter. (<b>c</b>) Daily average temperature. (<b>d</b>) Temperature seasonality, the standard deviation of the monthly means. (<b>e</b>) Growing degree days heat sum above 10 °C. (<b>f</b>) Growing degree days heat sum above 10 °C during the growing season. (<b>g</b>) Climate mean of growing degree days with an average daily temperature higher than 10 °C.</p> Full article ">Figure 11
<p>Notch boxplots of the outlined indices from the CHELSA dataset in the vineyard areas of Montenegro, for the historical period (1971–2010) and for the future period (2041–2070) models under SSP1-2.6, SSP3-7.0 and SSP5-8.5. (<b>a</b>) Climate means daily average temperature during the vineyard growing season, from April to October. (<b>b</b>) Climate means daily average temperature during the warmest quarter. (<b>c</b>) Climate annual means daily average temperature. (<b>d</b>) Climate means of temperature seasonality, the standard deviation of the monthly means. (<b>e</b>) Climate means of GDD10. (<b>f</b>) Climate means of WI. (<b>g</b>) Climate means of NGD10.</p> Full article ">
<p>Representation of the Study Area. (<b>a</b>) is the location of Montenegro and contiguous countries. (<b>b</b>) is the location of Montenegro vineyards and other land uses, according to CLC 2018, and the location of weather stations. (<b>c</b>) portrays the elevation of Montenegro, in 10 quantiles. In (<b>d</b>), the vineyards of Montenegro near Podgorica’s weather station are shown. In (<b>e</b>), an ombrothermic diagram of Podgorica weather station data (cf. legend for details) during the Historical period (1981–2010) is depicted.</p> Full article ">Figure 2
<p>Representation of the climate average of daily average temperature during the growing season (from April to October), for the Historical period (1981–2010), for CHELSA (<b>a</b>) with a resolution of 0.008°, E-OBS (<b>b</b>) with a resolution of 0.1°, and ERA5-Land (<b>c</b>) with a resolution of 0.1°.</p> Full article ">Figure 3
<p>Representation of the climate annual precipitation during the growing season (from April to October), for the Historical period (1981–2010), for CHELSA (<b>a</b>) with a resolution of 0.008°, E-OBS (<b>b</b>) with a resolution of 0.1°, and ERA5-Land (<b>c</b>) with a resolution of 0.1°.</p> Full article ">Figure 4
<p>Climate mean daily average temperature during the vineyard growing season from April to October, for Montenegro, according to the CHELSA dataset (<b>a</b>) over the historical period (1981–2010) and the future period (2041–2070) under (<b>b</b>) SSP1-2.6, (<b>c</b>) SSP3-7.0, and (<b>d</b>) SSP5-8.5. (<b>e</b>) Corresponding Kernel density plot of the spatial variability in (<b>a</b>–<b>d</b>). The median value in the vineyard area is shown as a vertical dashed line. Projections were ensembled from 5 climate models through the median.</p> Full article ">Figure 5
<p>Climate mean daily average temperature during the warmest quarter for Montenegro according to the CHELSA dataset (<b>a</b>) over the historical period (1981–2010) and the future period (2041–2070) under (<b>b</b>) SSP1-2.6, (<b>c</b>) SSP3-7.0, and (<b>d</b>) SSP5-8.5. (<b>e</b>) Corresponding Kernel density plot of the spatial variability in (<b>a</b>–<b>d</b>). The median value in the vineyard area is shown as a vertical dashed line. Projections were estimated by the ensemble median from 5 climate models.</p> Full article ">Figure 6
<p>Climate mean of temperature seasonality; the standard deviation of the monthly means for Montenegro according to the CHELSA dataset (<b>a</b>) over the historical period (1981–2010) and the future period (2041–2070) under (<b>b</b>) SSP1-2.6, (<b>c</b>) SSP3-7.0 and (<b>d</b>) SSP5-8.5. (<b>e</b>) Corresponding Kernel density plot of the spatial variability in (<b>a</b>–<b>d</b>). The median value in the vineyard area is shown as a vertical dashed line. Projections were estimated by the ensemble median from 5 climate models.</p> Full article ">Figure 7
<p>Climate mean growing degree days heat sum above 10 °C for Montenegro according to the CHELSA dataset (<b>a</b>) over the historical period (1981–2010) and the future period (2041–2070) under (<b>b</b>) SSP1-2.6, (<b>c</b>) SSP3-7.0 and (<b>d</b>) SSP5-8.5. (<b>e</b>) Corresponding Kernel density plot of the spatial variability in (<b>a</b>–<b>d</b>). The median value in the vineyard area is shown as a vertical dashed line. Projections were estimated by the ensemble median from 5 climate models.</p> Full article ">Figure 8
<p>Climate annual mean of Winkler index for Montenegro according to the CHELSA dataset (<b>a</b>) over the historical period (1981–2010) and the future period (2041–2070) under (<b>b</b>) SSP1-2.6, (<b>c</b>) SSP3-7.0 and (<b>d</b>) SSP5-8.5. (<b>e</b>) Corresponding Kernel density plot of the spatial variability in (<b>a</b>–<b>d</b>). The median value in the vineyard area is shown as a vertical dashed line. Projections were estimated by the ensemble median from 5 climate models.</p> Full article ">Figure 9
<p>Climate mean of growing degree days with an average daily temperature higher than 10 °C for Montenegro, according to the CHELSA dataset (<b>a</b>) over the historical period (1981–2010) and the future period (2041–2070), under (<b>b</b>) SSP1-2.6, (<b>c</b>) SSP3-7.0 and (<b>d</b>) SSP5-8.5. (<b>e</b>) Corresponding Kernel density plot of the spatial variability in (<b>a</b>–<b>d</b>). The median value in the vineyard area is shown as a vertical dashed line. Projections were estimated by the ensemble median from 5 climate models.</p> Full article ">Figure 10
<p>Chronograms of the annual averages of the outlined indices for Podgorica weather station during the historical period (1981–2010). (<b>a</b>) Daily mean temperature during the vineyard growing season, from April to October. (<b>b</b>) Daily mean temperature during the warmest quarter. (<b>c</b>) Daily average temperature. (<b>d</b>) Temperature seasonality, the standard deviation of the monthly means. (<b>e</b>) Growing degree days heat sum above 10 °C. (<b>f</b>) Growing degree days heat sum above 10 °C during the growing season. (<b>g</b>) Climate mean of growing degree days with an average daily temperature higher than 10 °C.</p> Full article ">Figure 11
<p>Notch boxplots of the outlined indices from the CHELSA dataset in the vineyard areas of Montenegro, for the historical period (1971–2010) and for the future period (2041–2070) models under SSP1-2.6, SSP3-7.0 and SSP5-8.5. (<b>a</b>) Climate means daily average temperature during the vineyard growing season, from April to October. (<b>b</b>) Climate means daily average temperature during the warmest quarter. (<b>c</b>) Climate annual means daily average temperature. (<b>d</b>) Climate means of temperature seasonality, the standard deviation of the monthly means. (<b>e</b>) Climate means of GDD10. (<b>f</b>) Climate means of WI. (<b>g</b>) Climate means of NGD10.</p> Full article ">
Open AccessArticle
Multi-Criteria Decision Analysis to Evaluate the Geographic Potential of Alternative Photovoltaic Types
by
Franziska Hübl, Franz Welscher and Johannes Scholz
ISPRS Int. J. Geo-Inf. 2024, 13(8), 269; https://doi.org/10.3390/ijgi13080269 - 30 Jul 2024
Abstract
►▼
Show Figures
This paper contributes to the expansion of green energy production, which is being pursued in order to mitigate climate change and carbon emissions from energy production. It addresses the delineation of areas that are suitable for the application of photovoltaics in the context
[...] Read more.
This paper contributes to the expansion of green energy production, which is being pursued in order to mitigate climate change and carbon emissions from energy production. It addresses the delineation of areas that are suitable for the application of photovoltaics in the context of agricultural areas, water bodies, and parking spaces. Three specific photovoltaic types are examined in order to find out which criteria influence their geographic potential and whether spatial multi-criteria decision analysis methods are suitable for identifying suitable areas. The proposed approach consists of four steps: (1) collecting factors through expert interviews and questionnaires; (2) mapping the criteria to the spatial datasets; (3) deriving weighted scores for individual criteria through expert interviews; (4) applying the multi-criteria decision analysis method to compute and aggregate the final scores. We test our methodology at selected sites in the state of Styria, Austria. The test sites represent the topographical characteristics of the state and are about 5% of the size of Styria, approximately 820 km2. The key contributions are a weighted set of criteria that are relevant for the geographic potential of alternative photovoltaic types and the developed methodology to determine this potential.
Full article
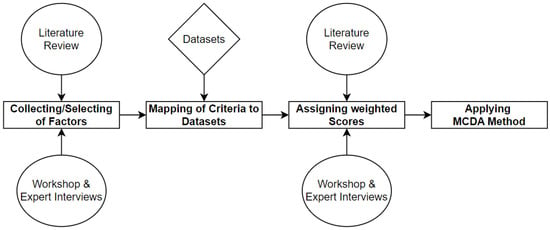
Figure 1
Figure 1
<p>Workflow of the applied methodology, consisting of four major steps with several inputs.</p> Full article ">Figure 2
<p>Example results for Agri-PV suitability showing an overlay of the 1 × 1 m raster containing the MCDA scores and their vectorized aggregation.</p> Full article ">Figure 3
<p>Example results for Floating-PV suitability showing an overlay of the 1 × 1 m raster containing the MCDA scores and their vectorized aggregation.</p> Full article ">Figure 4
<p>Example result for Parking-PV suitability showing an overlay of the 1 × 1 raster containing the MCDA scores and their vectorized aggregation.</p> Full article ">
<p>Workflow of the applied methodology, consisting of four major steps with several inputs.</p> Full article ">Figure 2
<p>Example results for Agri-PV suitability showing an overlay of the 1 × 1 m raster containing the MCDA scores and their vectorized aggregation.</p> Full article ">Figure 3
<p>Example results for Floating-PV suitability showing an overlay of the 1 × 1 m raster containing the MCDA scores and their vectorized aggregation.</p> Full article ">Figure 4
<p>Example result for Parking-PV suitability showing an overlay of the 1 × 1 raster containing the MCDA scores and their vectorized aggregation.</p> Full article ">
Open AccessArticle
The Spatial Equilibrium Model of Elderly Care Facilities with High Spatiotemporal Sensitivity and Its Economic Associations Study
by
Hongyan Li, Rui Li, Jing Cai and Shunli Wang
ISPRS Int. J. Geo-Inf. 2024, 13(8), 268; https://doi.org/10.3390/ijgi13080268 - 27 Jul 2024
Abstract
►▼
Show Figures
The global population aging poses new challenges in allocating care facilities for the elderly. This demographic trend also influences economic development and the quality of urban life. However, current research focuses on the supply of elderly care facilities and primarily uses administrative divisions
[...] Read more.
The global population aging poses new challenges in allocating care facilities for the elderly. This demographic trend also influences economic development and the quality of urban life. However, current research focuses on the supply of elderly care facilities and primarily uses administrative divisions as a scale, resulting in low spatiotemporal sensitivity in evaluating the spatial equilibrium of elderly care facilities (SEECF). The relationship between the SEECF and economic development is not clear. In response to these problems, we proposed a spatial equilibrium model of elderly care facilities with high spatiotemporal sensitivity (SEM-HSTS) and explored the spatiotemporal associations between the SEECF and economic development. Considering the spatial accessibility rate of elderly care services (SARecs) and the spatiotemporal supply–demand ratio for elderly care services (STSDRecs), two types of supply–demand relationship factors were constructed. Then, a spatiotemporal accessibility of medical services (STAms) factor was obtained based on a modified two-step floating catchment area (M2SFCA) method. On this basis, the SEM-HSTS was constructed based on the theory of coordinated development. Further, a panel threshold model was employed to evaluate the influence relationships among population aging, SEECF, and gross domestic product (GDP) in different phases. Finally, spatial autocorrelation and Geodetector explored the spatial associations between SEECF and GDP across complex urban land use categories (ULUC). The experimental results at a 100-m grid scale showed that the SEM-HSTS exhibited higher spatiotemporal heterogeneity than the classical accessibility method, with elevated spatiotemporal sensitivity. Effectively identified various spatial imbalances, such as undersupply and resource waste. The panel model captured phased relationship changes, showing that SEECF had inhibitory and promotional effects on GDP in pre- and post-aging societies, with stronger effects as balance approached. Moreover, the combined interaction of ULUC and GDP had a more significant influence on SEECF than any individual factor, with GDP exerting a more significant influence. This study provides an empirical basis for creating resource-efficient elderly care facility systems and optimizing layouts.
Full article
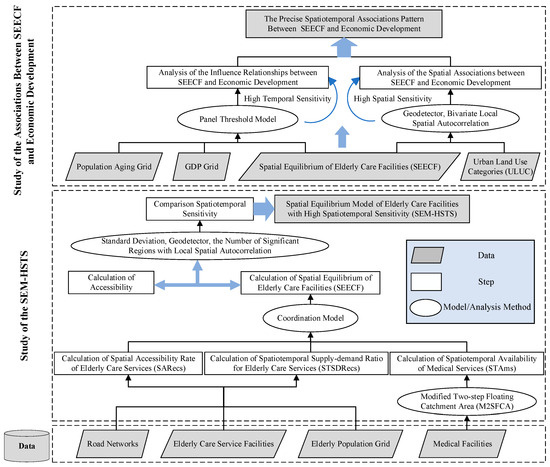
Figure 1
Figure 1
<p>Technology roadmap.</p> Full article ">Figure 2
<p>Location of the study area. (<b>a</b>) Location of Wuhan in Hubei Province; (<b>b</b>) location of central urban area in Wuhan; and (<b>c</b>) seven central districts.</p> Full article ">Figure 3
<p>SEECF (spatial equilibrium of elderly care facilities) (<b>a</b>–<b>c</b>) and accessibility (<b>d</b>–<b>f</b>) distribution maps of elderly care facilities in 2010, 2015, and 2020.</p> Full article ">Figure 4
<p>Distribution maps of kernel density of elderly care facilities and number of elderly populations in 2020.</p> Full article ">Figure 5
<p>BiLISA cluster map of SEECF and GDP in 2020.</p> Full article ">Figure 6
<p>The relative influences of ULUC (urban land use categories), GDP, and their interaction on SESECF (spatial equilibrium state of elderly care facilities). (<b>a</b>) The relative influences in spatial imbalance regions; (<b>b</b>) the relative influences in spatial balance regions.</p> Full article ">Figure 7
<p>Distribution maps of SEECF, GDP, and ULUC in 2020.</p> Full article ">Figure 8
<p>Statistical chart of SEECF, GDP, and ULUC.</p> Full article ">
<p>Technology roadmap.</p> Full article ">Figure 2
<p>Location of the study area. (<b>a</b>) Location of Wuhan in Hubei Province; (<b>b</b>) location of central urban area in Wuhan; and (<b>c</b>) seven central districts.</p> Full article ">Figure 3
<p>SEECF (spatial equilibrium of elderly care facilities) (<b>a</b>–<b>c</b>) and accessibility (<b>d</b>–<b>f</b>) distribution maps of elderly care facilities in 2010, 2015, and 2020.</p> Full article ">Figure 4
<p>Distribution maps of kernel density of elderly care facilities and number of elderly populations in 2020.</p> Full article ">Figure 5
<p>BiLISA cluster map of SEECF and GDP in 2020.</p> Full article ">Figure 6
<p>The relative influences of ULUC (urban land use categories), GDP, and their interaction on SESECF (spatial equilibrium state of elderly care facilities). (<b>a</b>) The relative influences in spatial imbalance regions; (<b>b</b>) the relative influences in spatial balance regions.</p> Full article ">Figure 7
<p>Distribution maps of SEECF, GDP, and ULUC in 2020.</p> Full article ">Figure 8
<p>Statistical chart of SEECF, GDP, and ULUC.</p> Full article ">
Open AccessArticle
Exploring the Spatiotemporal Patterns of Passenger Flows in Expanding Urban Metros: A Case Study of Shenzhen
by
Sirui Lv, Hu Yang, Xin Lu, Fan Zhang and Pu Wang
ISPRS Int. J. Geo-Inf. 2024, 13(8), 267; https://doi.org/10.3390/ijgi13080267 - 26 Jul 2024
Abstract
►▼
Show Figures
Despite extensive investigations on urban metro passenger flows, their evolving spatiotemporal patterns with the extensions of urban metro networks have not been well understood. Using Shenzhen as a case study city, our study initiates an investigation into this matter by analyzing the evolving
[...] Read more.
Despite extensive investigations on urban metro passenger flows, their evolving spatiotemporal patterns with the extensions of urban metro networks have not been well understood. Using Shenzhen as a case study city, our study initiates an investigation into this matter by analyzing the evolving network topology of Shenzhen Metro. Subsequently, leveraging long-term smart card data, we analyze the evolving spatiotemporal patterns of passenger flows and develop an analytical approach to pinpoint the major passenger sources of urban metro congestion. While the passenger travel demand and the passenger flow volumes kept increasing with the extension of the urban metro network, the major passenger sources were very stable in space, highlighting the inherent invariance in the evolution of the urban metro system. Finally, we analyze the impact of population and land use factors on passenger flow contributions of passenger sources, obtaining useful clues to foresee future passenger flow conditions.
Full article
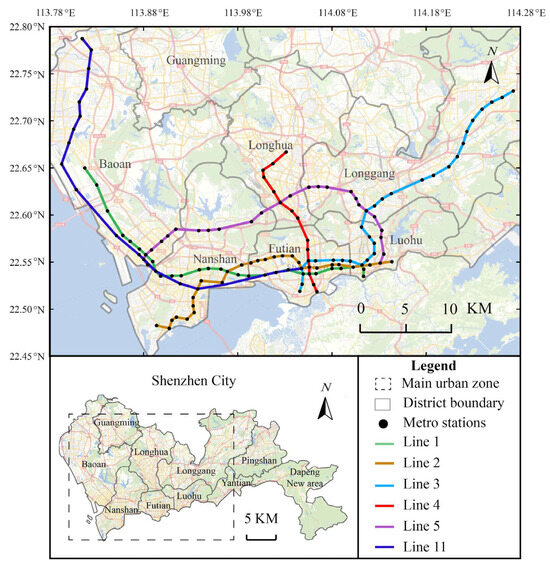
Figure 1
Figure 1
<p>The studied area and the Shenzhen Metro network.</p> Full article ">Figure 2
<p>Analytical framework of this study.</p> Full article ">Figure 3
<p>The evolving networks of Shenzhen Metro. (<b>a</b>) The Shenzhen Metro network in November 2014 and April 2016. (<b>b</b>) The Shenzhen Metro network in August 2016. (<b>c</b>) The Shenzhen Metro network in December 2016 and January 2018. (<b>d</b>) Enlarged view of Line 7 and Line 9.</p> Full article ">Figure 4
<p>The network topology changes in the expanding urban metro. (<b>a</b>) The number of stations. (<b>b</b>) The number of sections. (<b>c</b>) The total length of the sections. (<b>d</b>) The number of transfer stations. (<b>e</b>) The average degree of the network. (<b>f</b>) The average path length of the network.</p> Full article ">Figure 5
<p>The evolution of passenger travel demand. (<b>a</b>) The passenger travel demand. (<b>b</b>) The average trip distance.</p> Full article ">Figure 6
<p>The average hourly passenger flow volumes during the operation period (5:30 a.m.–11:30 p.m.).</p> Full article ">Figure 7
<p>Distribution of OD passenger travel demand. (<b>a</b>) November 2014; (<b>b</b>) April 2016; (<b>c</b>) August 2016; (<b>d</b>) December 2016; (<b>e</b>) January 2018.</p> Full article ">Figure 8
<p>The evolution of section passenger flow. (<b>a</b>) Distribution of section passenger flow. (<b>b</b>) The Gini coefficient of section passenger flow.</p> Full article ">Figure 9
<p>The number of congested sections (<b>a</b>) and the number of passengers using congested sections (<b>b</b>) throughout the five studied time periods.</p> Full article ">Figure 10
<p>The changes in passenger flow volumes of the congested sections during a morning peak hour (7:30–8:30 a.m.). (<b>a</b>) Comparison between November 2014 and April 2016. (<b>b</b>) Comparison between April 2016 and August 2016. (<b>c</b>) Comparison between August 2016 and December 2016. (<b>d</b>) Comparison between December 2016 and January 2018.</p> Full article ">Figure 11
<p>The evolution of passenger flow contributions of passenger sources. (<b>a</b>) Passenger flow contributions of passenger sources across the five studied time periods. The scattered points of different colors represent the actual data, and corresponding colored lines represent the fitted functions. (<b>b</b>) The Gini coefficient of passenger flow contributions.</p> Full article ">Figure 12
<p>The major passenger sources of Shenzhen Metro in January 2018.</p> Full article ">
<p>The studied area and the Shenzhen Metro network.</p> Full article ">Figure 2
<p>Analytical framework of this study.</p> Full article ">Figure 3
<p>The evolving networks of Shenzhen Metro. (<b>a</b>) The Shenzhen Metro network in November 2014 and April 2016. (<b>b</b>) The Shenzhen Metro network in August 2016. (<b>c</b>) The Shenzhen Metro network in December 2016 and January 2018. (<b>d</b>) Enlarged view of Line 7 and Line 9.</p> Full article ">Figure 4
<p>The network topology changes in the expanding urban metro. (<b>a</b>) The number of stations. (<b>b</b>) The number of sections. (<b>c</b>) The total length of the sections. (<b>d</b>) The number of transfer stations. (<b>e</b>) The average degree of the network. (<b>f</b>) The average path length of the network.</p> Full article ">Figure 5
<p>The evolution of passenger travel demand. (<b>a</b>) The passenger travel demand. (<b>b</b>) The average trip distance.</p> Full article ">Figure 6
<p>The average hourly passenger flow volumes during the operation period (5:30 a.m.–11:30 p.m.).</p> Full article ">Figure 7
<p>Distribution of OD passenger travel demand. (<b>a</b>) November 2014; (<b>b</b>) April 2016; (<b>c</b>) August 2016; (<b>d</b>) December 2016; (<b>e</b>) January 2018.</p> Full article ">Figure 8
<p>The evolution of section passenger flow. (<b>a</b>) Distribution of section passenger flow. (<b>b</b>) The Gini coefficient of section passenger flow.</p> Full article ">Figure 9
<p>The number of congested sections (<b>a</b>) and the number of passengers using congested sections (<b>b</b>) throughout the five studied time periods.</p> Full article ">Figure 10
<p>The changes in passenger flow volumes of the congested sections during a morning peak hour (7:30–8:30 a.m.). (<b>a</b>) Comparison between November 2014 and April 2016. (<b>b</b>) Comparison between April 2016 and August 2016. (<b>c</b>) Comparison between August 2016 and December 2016. (<b>d</b>) Comparison between December 2016 and January 2018.</p> Full article ">Figure 11
<p>The evolution of passenger flow contributions of passenger sources. (<b>a</b>) Passenger flow contributions of passenger sources across the five studied time periods. The scattered points of different colors represent the actual data, and corresponding colored lines represent the fitted functions. (<b>b</b>) The Gini coefficient of passenger flow contributions.</p> Full article ">Figure 12
<p>The major passenger sources of Shenzhen Metro in January 2018.</p> Full article ">
Open AccessArticle
Built Environment Effect on Metro Ridership in Metropolitan Area of Valparaíso, Chile, under Different Influence Area Approaches
by
Vicente Aprigliano, Sebastian Seriani, Catalina Toro, Gonzalo Rojas, Mitsuyoshi Fukushi, Marcus Cardoso, Marcelino Aurelio Vieira da Silva, Cristo Cucumides, Ualison Rébula de Oliveira, Cristián Henríquez, Andreas Braun and Volker Hochschild
ISPRS Int. J. Geo-Inf. 2024, 13(8), 266; https://doi.org/10.3390/ijgi13080266 - 26 Jul 2024
Abstract
The growing relevance of promoting a transition of urban mobility toward more sustainable modes of transport is leading to efforts to understand the effects of the built environment on the use of railway systems. In this direction, there are challenges regarding the creation
[...] Read more.
The growing relevance of promoting a transition of urban mobility toward more sustainable modes of transport is leading to efforts to understand the effects of the built environment on the use of railway systems. In this direction, there are challenges regarding the creation of coherence between the locations of metro stations and their surroundings, which has been explored extensively in the academic community. This process is called Transit-Oriented Development (TOD). Within the context of Latin America, this study seeks to assess the influence of the built environment on the metro ridership in the metropolitan area of Valparaíso, Chile, testing two approaches of influence area definition, one of which is a fixed distance from the stations, and the other is based on the origin and destination survey of the study area. The analysis is based on Ordinary Least Squares regression (OLS) to identify the factors from the built environment, which affects the metro’s ridership. Results show that the models based on the area of influence defined through the use of the origin and destination survey explain the metro ridership better. Moreover, this study reveals that the metro system in Greater Valparaíso was not planned in harmony with urban development. The models demonstrate an inverse effect of the built environment on ridership, contrasting with the expected outcomes of a metro station designed following a Transit-Oriented Development approach.
Full article
(This article belongs to the Special Issue Harnessing the Geospatial Data Revolution for Promoting Sustainable Transport Systems)
►▼
Show Figures
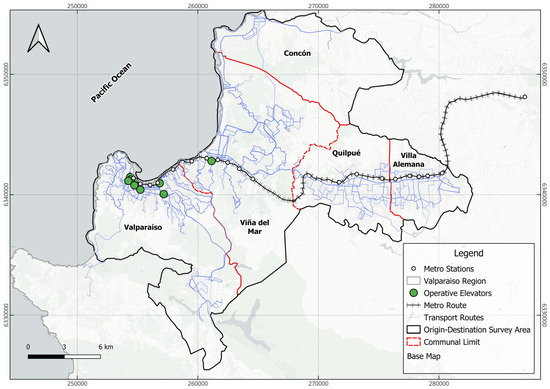
Figure 1
Figure 1
<p>Transportation context in the Metropolitan Area of Valparaíso. Observation: the names on the map refer to the cities in the Metropolitan Area of Valparaíso. Source: Elaborated by authors.</p> Full article ">Figure 2
<p>EFE Valparaíso metro network and stations within the Greater Valparaíso. Observation: the names on the map refer to the cities in the Metropolitan Area of Valparaíso. Source: elaborated by authors.</p> Full article ">Figure 3
<p>Walkable influence areas of origin of people heading 250 m, 500 m, and 750 m from metro stations. Observation: the names on the map refer to the metro stations. Source: elaborated by authors.</p> Full article ">Figure 4
<p>Density of origin of people who go to an area of influence of 250 m from the metro stations of Greater Valparaíso by modal choice: (<b>a</b>) Walking; (<b>b</b>) Bicycle; (<b>c</b>) Public transport; (<b>d</b>) Private transport. Source: elaborated by authors.</p> Full article ">Figure 5
<p>(<b>a</b>) Annual metro passenger flow between the years 2018 and 2023 for the Greater Valparaíso area that covers the metro network. (<b>b</b>) Average annual flow in the metro stations of Greater Valparaíso for work and holiday months. Source: elaborated by authors.</p> Full article ">Figure 6
<p>Average passenger flow per month between 2018 and 2023, categorized by work and holiday months. Source: elaborated by authors.</p> Full article ">Figure 7
<p>Areas of influence of metro stations in Greater Valparaíso with a radius of 400 meters. Observation: the names on the top of each part of the figure refer to the city on the map. Source: elaborated by authors.</p> Full article ">Figure 8
<p>Influence areas of metro stations in Greater Valparaíso reclassified and vectorized using KDE. Observation: the names on the top of each part of the figure refer to the city on the map and the numbers refers to the information in <a href="#ijgi-13-00266-f002" class="html-fig">Figure 2</a>. Source: elaborated by authors.</p> Full article ">
<p>Transportation context in the Metropolitan Area of Valparaíso. Observation: the names on the map refer to the cities in the Metropolitan Area of Valparaíso. Source: Elaborated by authors.</p> Full article ">Figure 2
<p>EFE Valparaíso metro network and stations within the Greater Valparaíso. Observation: the names on the map refer to the cities in the Metropolitan Area of Valparaíso. Source: elaborated by authors.</p> Full article ">Figure 3
<p>Walkable influence areas of origin of people heading 250 m, 500 m, and 750 m from metro stations. Observation: the names on the map refer to the metro stations. Source: elaborated by authors.</p> Full article ">Figure 4
<p>Density of origin of people who go to an area of influence of 250 m from the metro stations of Greater Valparaíso by modal choice: (<b>a</b>) Walking; (<b>b</b>) Bicycle; (<b>c</b>) Public transport; (<b>d</b>) Private transport. Source: elaborated by authors.</p> Full article ">Figure 5
<p>(<b>a</b>) Annual metro passenger flow between the years 2018 and 2023 for the Greater Valparaíso area that covers the metro network. (<b>b</b>) Average annual flow in the metro stations of Greater Valparaíso for work and holiday months. Source: elaborated by authors.</p> Full article ">Figure 6
<p>Average passenger flow per month between 2018 and 2023, categorized by work and holiday months. Source: elaborated by authors.</p> Full article ">Figure 7
<p>Areas of influence of metro stations in Greater Valparaíso with a radius of 400 meters. Observation: the names on the top of each part of the figure refer to the city on the map. Source: elaborated by authors.</p> Full article ">Figure 8
<p>Influence areas of metro stations in Greater Valparaíso reclassified and vectorized using KDE. Observation: the names on the top of each part of the figure refer to the city on the map and the numbers refers to the information in <a href="#ijgi-13-00266-f002" class="html-fig">Figure 2</a>. Source: elaborated by authors.</p> Full article ">
Open AccessArticle
Building Height Extraction Based on Spatial Clustering and a Random Forest Model
by
Jingxin Chang, Yonghua Jiang, Meilin Tan, Yunming Wang and Shaodong Wei
ISPRS Int. J. Geo-Inf. 2024, 13(8), 265; https://doi.org/10.3390/ijgi13080265 - 26 Jul 2024
Abstract
►▼
Show Figures
Building height (BH) estimation is crucial for urban spatial planning and development. BH estimation using digital surface model data involves obtaining ground and roof elevations. However, vegetation and shadows around buildings affect the selection of the required elevation, resulting in large BH estimation
[...] Read more.
Building height (BH) estimation is crucial for urban spatial planning and development. BH estimation using digital surface model data involves obtaining ground and roof elevations. However, vegetation and shadows around buildings affect the selection of the required elevation, resulting in large BH estimation errors. In highly urbanized areas, buildings of similar heights often have similar characteristics and spatial proximity, which have reference significance in BH estimation but are rarely utilized. Herein, we propose a BH estimation method based on BIRCH clustering and a random forest (RF) model. We obtain the initial BH results using a method based on the optimal ground search area and a multi-index evaluation. BIRCH clustering and an RF classification model are used to match buildings of similar heights based on their spatial distance and attribute characteristics. Finally, the BH is adjusted based on the ground elevation obtained from the secondary screening and the BH matching. The validation results from two areas with over 12,000 buildings show that the proposed method reduces the root-mean-square error of the final BH results compared with the initial results. Comparing the obtained height maps shows that the final results produce a relatively accurate BH in areas with high shading and vegetation coverage, as well as in areas with dense buildings. Thus, the proposed method has been validated for its effectiveness and reliability.
Full article
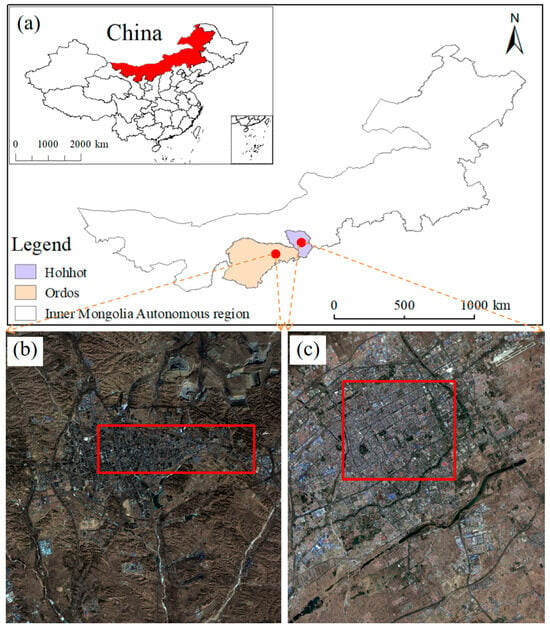
Figure 1
Figure 1
<p>Study areas and corresponding validation areas. (<b>a</b>) Geographical location of the study area. (<b>b</b>) Main built-up area in Ordos City; the area within the red box is verification area S1. (<b>c</b>) Main built-up area in Hohhot City; the area within the red box is verification area S2.</p> Full article ">Figure 2
<p>Flowchart of the proposed framework.</p> Full article ">Figure 3
<p>Calculation of the SD length of a building. (<b>a</b>) Point set and SD area of BC. (<b>b</b>) Area in which the building outline expands and overlaps with the SD. (<b>c</b>) Point set of the SD closest to the outline of the building. (<b>d</b>) Filtered point set of SD. (<b>e</b>) Classification of calculation methods for the SD length. (<b>f</b>) Final calculation result for the SD length of the example building.</p> Full article ">Figure 4
<p>Classification results for different validation areas based on the building ground elevation and building height. (<b>a</b>,<b>b</b>) are the classification results of S1 based on BH and building ground elevation, respectively. (<b>c</b>,<b>d</b>) are the classification results of S2 based on BH and building ground elevation, respectively.</p> Full article ">Figure 4 Cont.
<p>Classification results for different validation areas based on the building ground elevation and building height. (<b>a</b>,<b>b</b>) are the classification results of S1 based on BH and building ground elevation, respectively. (<b>c</b>,<b>d</b>) are the classification results of S2 based on BH and building ground elevation, respectively.</p> Full article ">Figure 5
<p>Comparison of the initial BH and NBH height maps of the IBH in validation region S1. (<b>a</b>) Reference height map of IBH. (<b>b</b>) Height map of IBH. (<b>c</b>) Height map of the NBH for IBH.</p> Full article ">Figure 6
<p>Comparison of the initial BH and NBH height maps of the IBH in validation region S2. (<b>a</b>) Reference height map of the IBH. (<b>b</b>) Height map of the IBH. (<b>c</b>) Height map of the NBH for the IBH.</p> Full article ">Figure 7
<p>Evaluation of the accuracy of the initial BH and NBH for IBH buildings in validation regions S1 and S2. (<b>a</b>,<b>b</b>) are the accuracy evaluations of the initial BH and NBH of IBH buildings in the validation area S1, respectively. (<b>c</b>,<b>d</b>) are the accuracy evaluations of the initial BH and NBH of IBH buildings in the validation area S2, respectively.</p> Full article ">Figure 8
<p>Multispectral images of validation regions S1 and S2 and the locations of small regions. (<b>a</b>) Multispectral image of validation region S1 and the locations of small regions A1–A3. (<b>b</b>) Multispectral image of validation region S2 and the locations of small regions A4–A6.</p> Full article ">Figure 8 Cont.
<p>Multispectral images of validation regions S1 and S2 and the locations of small regions. (<b>a</b>) Multispectral image of validation region S1 and the locations of small regions A1–A3. (<b>b</b>) Multispectral image of validation region S2 and the locations of small regions A4–A6.</p> Full article ">Figure 9
<p>Comparison of the results for small areas in validation regions S1 and S2.</p> Full article ">
<p>Study areas and corresponding validation areas. (<b>a</b>) Geographical location of the study area. (<b>b</b>) Main built-up area in Ordos City; the area within the red box is verification area S1. (<b>c</b>) Main built-up area in Hohhot City; the area within the red box is verification area S2.</p> Full article ">Figure 2
<p>Flowchart of the proposed framework.</p> Full article ">Figure 3
<p>Calculation of the SD length of a building. (<b>a</b>) Point set and SD area of BC. (<b>b</b>) Area in which the building outline expands and overlaps with the SD. (<b>c</b>) Point set of the SD closest to the outline of the building. (<b>d</b>) Filtered point set of SD. (<b>e</b>) Classification of calculation methods for the SD length. (<b>f</b>) Final calculation result for the SD length of the example building.</p> Full article ">Figure 4
<p>Classification results for different validation areas based on the building ground elevation and building height. (<b>a</b>,<b>b</b>) are the classification results of S1 based on BH and building ground elevation, respectively. (<b>c</b>,<b>d</b>) are the classification results of S2 based on BH and building ground elevation, respectively.</p> Full article ">Figure 4 Cont.
<p>Classification results for different validation areas based on the building ground elevation and building height. (<b>a</b>,<b>b</b>) are the classification results of S1 based on BH and building ground elevation, respectively. (<b>c</b>,<b>d</b>) are the classification results of S2 based on BH and building ground elevation, respectively.</p> Full article ">Figure 5
<p>Comparison of the initial BH and NBH height maps of the IBH in validation region S1. (<b>a</b>) Reference height map of IBH. (<b>b</b>) Height map of IBH. (<b>c</b>) Height map of the NBH for IBH.</p> Full article ">Figure 6
<p>Comparison of the initial BH and NBH height maps of the IBH in validation region S2. (<b>a</b>) Reference height map of the IBH. (<b>b</b>) Height map of the IBH. (<b>c</b>) Height map of the NBH for the IBH.</p> Full article ">Figure 7
<p>Evaluation of the accuracy of the initial BH and NBH for IBH buildings in validation regions S1 and S2. (<b>a</b>,<b>b</b>) are the accuracy evaluations of the initial BH and NBH of IBH buildings in the validation area S1, respectively. (<b>c</b>,<b>d</b>) are the accuracy evaluations of the initial BH and NBH of IBH buildings in the validation area S2, respectively.</p> Full article ">Figure 8
<p>Multispectral images of validation regions S1 and S2 and the locations of small regions. (<b>a</b>) Multispectral image of validation region S1 and the locations of small regions A1–A3. (<b>b</b>) Multispectral image of validation region S2 and the locations of small regions A4–A6.</p> Full article ">Figure 8 Cont.
<p>Multispectral images of validation regions S1 and S2 and the locations of small regions. (<b>a</b>) Multispectral image of validation region S1 and the locations of small regions A1–A3. (<b>b</b>) Multispectral image of validation region S2 and the locations of small regions A4–A6.</p> Full article ">Figure 9
<p>Comparison of the results for small areas in validation regions S1 and S2.</p> Full article ">
Open AccessArticle
Automatic Vehicle Trajectory Behavior Classification Based on Unmanned Aerial Vehicle-Derived Trajectories Using Machine Learning Techniques
by
Tee-Ann Teo, Min-Jhen Chang and Tsung-Han Wen
ISPRS Int. J. Geo-Inf. 2024, 13(8), 264; https://doi.org/10.3390/ijgi13080264 - 26 Jul 2024
Abstract
This study introduces an innovative scheme for classifying uncrewed aerial vehicle (UAV)-derived vehicle trajectory behaviors by employing machine learning (ML) techniques to transform original trajectories into various sequences: space–time, speed–time, and azimuth–time. These transformed sequences were subjected to normalization for uniform data analysis,
[...] Read more.
This study introduces an innovative scheme for classifying uncrewed aerial vehicle (UAV)-derived vehicle trajectory behaviors by employing machine learning (ML) techniques to transform original trajectories into various sequences: space–time, speed–time, and azimuth–time. These transformed sequences were subjected to normalization for uniform data analysis, facilitating the classification of trajectories into six distinct categories through the application of three ML classifiers: random forest, time series forest (TSF), and canonical time series characteristics. Testing was performed across three different intersections to reveal an accuracy exceeding 90%, underlining the superior performance of integrating azimuth–time and speed–time sequences over conventional space–time sequences for analyzing trajectory behaviors. This research highlights the TSF classifier’s robustness when incorporating speed data, demonstrating its efficiency in feature extraction and reliability in intricate trajectory pattern handling. This study’s results indicate that integrating direction and speed information significantly enhances predictive accuracy and model robustness. This comprehensive approach, which leverages UAV-derived trajectories and advanced ML techniques, represents a significant step forward in understanding vehicle trajectory behaviors, aligning with the goals of enhancing traffic control and management strategies for better urban mobility.
Full article
(This article belongs to the Special Issue Harnessing the Geospatial Data Revolution for Promoting Sustainable Transport Systems)
►▼
Show Figures
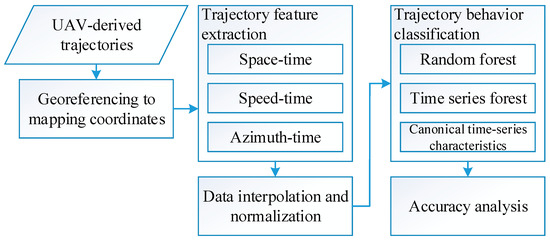
Figure 1
Figure 1
<p>Architecture of the proposed method.</p> Full article ">Figure 2
<p>Processes of transforming a trajectory: (<b>a</b>) original trajectory, (<b>b</b>) space–time, (<b>c</b>) velocity, (<b>d</b>) original azimuth–time, (<b>e</b>) relative azimuth–time, (<b>f</b>) normalized azimuth, (<b>g</b>) normalized distance, and (<b>h</b>) normalized speed.</p> Full article ">Figure 2 Cont.
<p>Processes of transforming a trajectory: (<b>a</b>) original trajectory, (<b>b</b>) space–time, (<b>c</b>) velocity, (<b>d</b>) original azimuth–time, (<b>e</b>) relative azimuth–time, (<b>f</b>) normalized azimuth, (<b>g</b>) normalized distance, and (<b>h</b>) normalized speed.</p> Full article ">Figure 3
<p>Illustration of trajectory behaviors.</p> Full article ">Figure 4
<p>Illustrations of the three intersections (the blue and yellow solid lines indicate different road center lines; the yellow dashed line indicates the extension of road center line): (<b>a</b>) intersection A, (<b>b</b>) intersection B, and (<b>c</b>) intersection C.</p> Full article ">Figure 5
<p>Results of the test data (intersection A) classified using RF.</p> Full article ">Figure 6
<p>Confusion matrix for the test data (intersection A) using azimuth– and speed–time with RF.</p> Full article ">Figure 7
<p>Incorrectly classified trajectory at the beginning of the dataset.</p> Full article ">Figure 8
<p>Results of intersection B classified using TSF.</p> Full article ">Figure 9
<p>Confusion matrix for intersection B using azimuth– and speed–time with TSF.</p> Full article ">Figure 10
<p>Incorrectly identified trajectories in category 4. (<b>a</b>,<b>b</b>) IDs 2150 and 3038, misclassification of trajectories due to traffic jams. (<b>c</b>,<b>d</b>) IDs 3335 and 3403, traffic delay due to left turn signal wait.</p> Full article ">Figure 11
<p>Results of intersection C classified using TSF: (<b>a</b>) go straight; (<b>b</b>) turn left; (<b>c</b>) turn right; (<b>d</b>) stop and go straight; (<b>e</b>) stop and turn left; (<b>f</b>) stop and turn right.</p> Full article ">Figure 12
<p>Confusion matrix for intersection C using azimuth– and speed–time with TSF.</p> Full article ">Figure 13
<p>Incorrectly identified trajectories in intersection C. (<b>a</b>,<b>b</b>) IDs 43 and 2714, incorrect classification due sudden change in direction; (<b>c</b>,<b>d</b>) ID 4937 and 4967, incorrect classification due to traffic jams.</p> Full article ">
<p>Architecture of the proposed method.</p> Full article ">Figure 2
<p>Processes of transforming a trajectory: (<b>a</b>) original trajectory, (<b>b</b>) space–time, (<b>c</b>) velocity, (<b>d</b>) original azimuth–time, (<b>e</b>) relative azimuth–time, (<b>f</b>) normalized azimuth, (<b>g</b>) normalized distance, and (<b>h</b>) normalized speed.</p> Full article ">Figure 2 Cont.
<p>Processes of transforming a trajectory: (<b>a</b>) original trajectory, (<b>b</b>) space–time, (<b>c</b>) velocity, (<b>d</b>) original azimuth–time, (<b>e</b>) relative azimuth–time, (<b>f</b>) normalized azimuth, (<b>g</b>) normalized distance, and (<b>h</b>) normalized speed.</p> Full article ">Figure 3
<p>Illustration of trajectory behaviors.</p> Full article ">Figure 4
<p>Illustrations of the three intersections (the blue and yellow solid lines indicate different road center lines; the yellow dashed line indicates the extension of road center line): (<b>a</b>) intersection A, (<b>b</b>) intersection B, and (<b>c</b>) intersection C.</p> Full article ">Figure 5
<p>Results of the test data (intersection A) classified using RF.</p> Full article ">Figure 6
<p>Confusion matrix for the test data (intersection A) using azimuth– and speed–time with RF.</p> Full article ">Figure 7
<p>Incorrectly classified trajectory at the beginning of the dataset.</p> Full article ">Figure 8
<p>Results of intersection B classified using TSF.</p> Full article ">Figure 9
<p>Confusion matrix for intersection B using azimuth– and speed–time with TSF.</p> Full article ">Figure 10
<p>Incorrectly identified trajectories in category 4. (<b>a</b>,<b>b</b>) IDs 2150 and 3038, misclassification of trajectories due to traffic jams. (<b>c</b>,<b>d</b>) IDs 3335 and 3403, traffic delay due to left turn signal wait.</p> Full article ">Figure 11
<p>Results of intersection C classified using TSF: (<b>a</b>) go straight; (<b>b</b>) turn left; (<b>c</b>) turn right; (<b>d</b>) stop and go straight; (<b>e</b>) stop and turn left; (<b>f</b>) stop and turn right.</p> Full article ">Figure 12
<p>Confusion matrix for intersection C using azimuth– and speed–time with TSF.</p> Full article ">Figure 13
<p>Incorrectly identified trajectories in intersection C. (<b>a</b>,<b>b</b>) IDs 43 and 2714, incorrect classification due sudden change in direction; (<b>c</b>,<b>d</b>) ID 4937 and 4967, incorrect classification due to traffic jams.</p> Full article ">
Open AccessArticle
Measuring Reliable Accessibility to High-Speed Railway Stations by Integrating the Utility-Based Model and Multimodal Space–Time Prism under Travel Time Uncertainty
by
Yongsheng Zhang, Kangyu Liang, Enjian Yao and Mingyi Gu
ISPRS Int. J. Geo-Inf. 2024, 13(8), 263; https://doi.org/10.3390/ijgi13080263 - 25 Jul 2024
Abstract
►▼
Show Figures
Measuring the accessibility of each traffic zone to high-speed railway stations can evaluate the ease of the transportation hub in the transportation system. The utility-based model, which captures individual travel behavior and subjective perception, is often used to quantify the travel impedance on
[...] Read more.
Measuring the accessibility of each traffic zone to high-speed railway stations can evaluate the ease of the transportation hub in the transportation system. The utility-based model, which captures individual travel behavior and subjective perception, is often used to quantify the travel impedance on accessibility for a given origin–destination pair. However, existing studies neglect the impacts of travel time uncertainty on utility and possible choice set when measuring accessibility, especially in high-timeliness travel (e.g., railway stations or airports). This study proposes a novel integration of the utility-based model and multimodal space–time prism under travel time uncertainty to measure reliable accessibility to high-speed railway stations. First, the reliable multimodal space–time prism is developed to generate a reliable travel mode choice set constrained by travel time budgets. Then, the reliable choice set is integrated into the utility-based model with the utility function derived from a proposed mean–standard deviation logit-based mode choice model. Finally, this study contributes to measuring reliable accessibility within areas from Beijing’s 5th Ring Road to the Beijing South Railway Station. Based on the results, policymakers can effectively evaluate the distribution of transportation resources and urban planning.
Full article
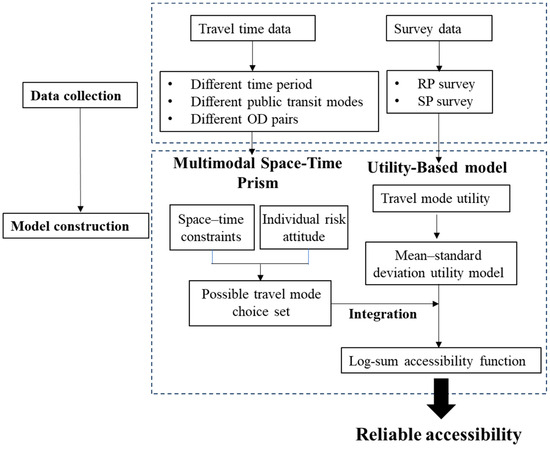
Figure 1
Figure 1
<p>The framework of the model.</p> Full article ">Figure 2
<p>Multimodal STPs in a given OD pair. (<b>a</b>) STP under the risk attitude of 5%; (<b>b</b>) STP under the risk attitude of 95%.</p> Full article ">Figure 3
<p>Multimodal STPs under the travel time constraint of 30 min and 60 min.</p> Full article ">Figure 4
<p>Study area and location of Beijing South Railway Station.</p> Full article ">Figure 5
<p>The statistical results of the collected travel time data. (<b>a</b>) The variation in travel time by taxi in a day from Donghuamen Street to Beijing South Railway Station. (<b>b</b>) Travel time distribution from Donghuamen Street to Beijing South Railway Station.</p> Full article ">Figure 6
<p>The statistical results of the survey data. (<b>a</b>) The proportion of travel time budgets. (<b>b</b>) The sample size of different public transit modes.</p> Full article ">Figure 7
<p>Accessibility at different reliability levels.</p> Full article ">Figure 8
<p>Accessibility for different travel time budgets.</p> Full article ">Figure 9
<p>Accessibility with different risk attitudes for 60 min travel time budget.</p> Full article ">Figure 10
<p>A comparison of accessibility in the Summer Palace, the National Stadium, and the Wangjing area.</p> Full article ">
<p>The framework of the model.</p> Full article ">Figure 2
<p>Multimodal STPs in a given OD pair. (<b>a</b>) STP under the risk attitude of 5%; (<b>b</b>) STP under the risk attitude of 95%.</p> Full article ">Figure 3
<p>Multimodal STPs under the travel time constraint of 30 min and 60 min.</p> Full article ">Figure 4
<p>Study area and location of Beijing South Railway Station.</p> Full article ">Figure 5
<p>The statistical results of the collected travel time data. (<b>a</b>) The variation in travel time by taxi in a day from Donghuamen Street to Beijing South Railway Station. (<b>b</b>) Travel time distribution from Donghuamen Street to Beijing South Railway Station.</p> Full article ">Figure 6
<p>The statistical results of the survey data. (<b>a</b>) The proportion of travel time budgets. (<b>b</b>) The sample size of different public transit modes.</p> Full article ">Figure 7
<p>Accessibility at different reliability levels.</p> Full article ">Figure 8
<p>Accessibility for different travel time budgets.</p> Full article ">Figure 9
<p>Accessibility with different risk attitudes for 60 min travel time budget.</p> Full article ">Figure 10
<p>A comparison of accessibility in the Summer Palace, the National Stadium, and the Wangjing area.</p> Full article ">
Open AccessArticle
The Influence of Perceptions of the Park Environment on the Health of the Elderly: The Mediating Role of Social Interaction
by
Xiuhai Xiong, Jingjing Wang, Hao Wu and Zhenghong Peng
ISPRS Int. J. Geo-Inf. 2024, 13(7), 262; https://doi.org/10.3390/ijgi13070262 - 22 Jul 2024
Abstract
The aging population has brought increased attention to the urgent need to address social isolation and health risks among the elderly. While previous research has established the positive effects of parks in promoting social interaction and health among older adults, further investigation is
[...] Read more.
The aging population has brought increased attention to the urgent need to address social isolation and health risks among the elderly. While previous research has established the positive effects of parks in promoting social interaction and health among older adults, further investigation is required to understand the complex relationships between perceptions of the park environment, social interaction, and elderly health. In this study, structural equation modeling (SEM) was employed to examine these relationships, using nine parks in Wuhan as a case study. The findings indicate that social interaction serves as a complete mediator between perceptions of the park environment and elderly health (path coefficients: park environment on social interaction = 0.45, social interaction on health = 0.46, and indirect effect = 0.182). Furthermore, the results of the multi-group SEM analysis revealed that the mediating effect was moderated by the pattern of social interaction (the difference test: the friend companionship group vs. the family companionship group (Z = 1.965 > 1.96)). Notably, family companionship had a significantly stronger positive impact on the health of older adults compared to friend companionship. These findings contribute to our understanding of the mechanisms through which urban parks support the physical and mental well-being of the elderly and provide a scientific foundation for optimizing urban park environments.
Full article
(This article belongs to the Topic Spatial Epidemiology and GeoInformatics)
►▼
Show Figures
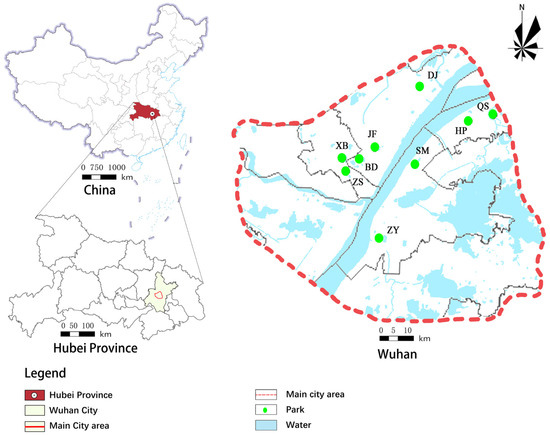
Figure 1
Figure 1
<p>Spatial distribution of surveyed parks. Note: “ZY”: Ziyang Park, “SM”:Simeitang Park, “JF”: Jiefang Park, “BD”: Baodao Park, “ZS”: Zhongshan Park, “XB”: Xibeihu Park, “QS”: Qingshan Park, “DJ”: Dijiao Park, “HP”: Heping Park.</p> Full article ">Figure 2
<p>Path coefficients of the structural equation model.</p> Full article ">
<p>Spatial distribution of surveyed parks. Note: “ZY”: Ziyang Park, “SM”:Simeitang Park, “JF”: Jiefang Park, “BD”: Baodao Park, “ZS”: Zhongshan Park, “XB”: Xibeihu Park, “QS”: Qingshan Park, “DJ”: Dijiao Park, “HP”: Heping Park.</p> Full article ">Figure 2
<p>Path coefficients of the structural equation model.</p> Full article ">
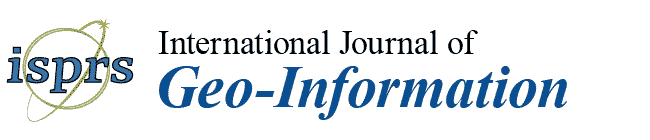
Journal Menu
► ▼ Journal Menu-
- IJGI Home
- Aims & Scope
- Editorial Board
- Reviewer Board
- Topical Advisory Panel
- Instructions for Authors
- Special Issues
- Topics
- Topical Collections
- Article Processing Charge
- Indexing & Archiving
- Editor’s Choice Articles
- Most Cited & Viewed
- Journal Statistics
- Journal History
- Journal Awards
- Society Collaborations
- Conferences
- Editorial Office
Journal Browser
► ▼ Journal BrowserHighly Accessed Articles
Latest Books
E-Mail Alert
News
Topics
Topic in
Applied Sciences, Atmosphere, IJGI, Remote Sensing, Sustainability
Technological Innovation and Emerging Operational Applications in Digital Earth
Topic Editors: Zhihua Zhang, M. James C. CrabbeDeadline: 17 October 2024
Topic in
Climate, Drones, Forests, Land, Remote Sensing, IJGI
Advances in Multi-Scale Geographic Environmental Monitoring: Theory, Methodology and Applications
Topic Editors: Jingzhe Wang, Yangyi Wu, Yinghui Zhang, Ivan Lizaga, Zipeng ZhangDeadline: 31 October 2024
Topic in
Agronomy, Applied Sciences, Climate, Forests, Remote Sensing, Sustainability, IJGI
Climate Change Impacts and Adaptation: Interdisciplinary Perspectives
Topic Editors: Cheng Li, Fei Zhang, Mou Leong Tan, Kwok Pan ChunDeadline: 31 December 2024
Topic in
Applied Sciences, GeoHazards, Geosciences, Remote Sensing, Minerals, IJGI
Geotechnics for Hazard Mitigation
Topic Editors: Mowen Xie, Yan Du, Yujing Jiang, Bo Li, Xuepeng ZhangDeadline: 31 March 2025

Conferences
Special Issues
Special Issue in
IJGI
HealthScape: Intersections of Health, Environment, and GIS&T
Guest Editors: Lan Mu, Jue YangDeadline: 31 August 2024
Special Issue in
IJGI
Advances in AI-Driven Geospatial Analysis and Data Generation
Guest Editors: Hao Li, Hartwig H. Hochmair, Levente JuhászDeadline: 31 August 2024
Special Issue in
IJGI
Unlocking the Power of Geospatial Data: Semantic Information Extraction, Ontology Engineering, and Deep Learning for Knowledge Discovery
Guest Editors: Margarita Kokla, Mara Nikolaidou, Christos Chalkias, Marinos KavourasDeadline: 30 September 2024
Special Issue in
IJGI
Geographic Information Systems and Cartography for a Sustainable World
Guest Editors: Andriani Skopeliti, Anastasia Stratigea, Vassilios Krassanakis, Apostolos LagariasDeadline: 31 December 2024
Topical Collections
Topical Collection in
IJGI
Spatial Components of COVID-19 Pandemic
Collection Editors: Fazlay S. Faruque, Peng Jia, Mo Ashifur Rahim
Topical Collection in
IJGI
Spatial and Temporal Modelling of Renewable Energy Systems
Collection Editors: Luis Ramirez Camargo, Johannes Schmidt, Wolfgang Dorner