Disclosure of Invention
The invention overcomes the defects of the prior art and provides an order distribution method for the Internet in the textile and clothing industry; the problem of order allocation in the industrial Internet is solved through a heuristic algorithm, and the effects of improving the platform manufacturing efficiency and the on-time delivery rate of products and improving the customer satisfaction degree are achieved.
In order to achieve the purpose, the invention adopts the technical scheme that: an order distribution method used in the textile and clothing industry internet comprises the following steps;
step S1, m orders, wherein m is selected from integers which are more than or equal to 1; disassembling the orders, wherein basic units of the clothing in each order, which can be disassembled, are a plurality of products, and the number of each product is m;
step S2, there are n factories in the textile and clothing industry Internet platform, wherein n is selected from integers of 1 or more, each factory has different production parameters for the production of different products, and the production parameters include one or more of quotation, fixed cost, maximum production and delivery delay rate; aiming at the production parameters of a factory and the optimization goal of order distribution; determining the constraint condition or/and the objective function of the model;
in step S3, m orders are assigned to n factories by a set genetic algorithm.
Specifically, from the perspective of processing habits, the detachable basic units of the garment should be a jacket and trousers. Therefore, the production task of the split order mainly comprises two products, namely m pieces of upper clothes and m pieces of lower clothes.
Specifically, the constraints of the model are the principles that include order allocation, which enables orders to be delivered on schedule and balances plant load within the platform to achieve relatively optimal order allocation goals.
In a preferred embodiment of the present invention, in step S2, an optimization objective is selected according to actual order production requirements, a fitness function is set through the objective function according to production parameters of a plant and characteristics of a genetic algorithm, individual fitness is calculated, population iteration is performed through one or more of selection operation, crossover operation, and mutation operation in the genetic algorithm, and when a termination condition is satisfied, the selected optimal order allocation scheme is output.
In a preferred embodiment of the present invention, the genetic algorithm is configured to include the steps of;
step a, n factories and m orders are arranged in a known platform, and each order comprises a plurality of products; each gene position corresponds to n-1 dividing points from 0 to m, the order is divided into n intervals, the number of the product orders obtained by each factory is obtained after decoding, and the length of the order distribution chromosome is 2 (n-1);
b, determining a fitness function, wherein the fitness function is used for evaluating whether the order distribution chromosome code is inherited into an evaluation function of the next generation, and the fitness function is a basis for executing the eligibility and the rejection of a genetic algorithm; the order dividing point coding chromosome in the order distribution chromosome with high fitness is preferentially inherited to the next generation; establishing an order optimization model through an objective function or/and a fitness function in combination with variables;
c, iteration of the population is performed through selection operation, cross operation and mutation operation in the genetic algorithm in sequence, and the selected optimal order distribution scheme is output after the termination condition is met;
step d, terminating conditions; step d, terminating conditions; and when the actual iteration times of the algorithm are more than or equal to the preset maximum iteration times, stopping the algorithm, and obtaining an optimal solution, namely a relatively optimal order distribution scheme required by the textile garment industry Internet platform in the multiple order distribution schemes.
In a preferred embodiment of the present invention, in step a, dpiDenotes the ith tangent point position, i is 1,2, n-1, then dp is [ dp ]1,dp2,...,dpi,...,dpn-1]An order cut point code chromosome representing an order allocation chromosome; decoding the chromosome to obtain corresponding each order dividing point in the order distribution chromosomeFactory order quantity [ dp ]1,dp2-dp1,...,dpi-dpi-1,...,m-dpn-1]。
In a preferred embodiment of the present invention, in step b, an order optimization model is established using variables;
comprises parameters or/and related parameters, wherein the parameters comprise i, j and yi、xij、aijOne or more of; the relevant parameters include pij、fi、bij、dij、ALi、AUiOne or more of;
i is a factory serial number, i is taken from a natural number not greater than n; n at this time is the total number of plants;
j is order number, and j is taken from a natural number not greater than m; m at this time is the number of orders;
yiis a variable of 0-1, when the platform selection factory i is responsible for the production of the order, y i1 is ═ 1; otherwise, yi=0;
xijIs a variable of 0 to 1, x when factory i produces order j ij1 is ═ 1; otherwise, xij=0;
aijIs the task volume of factory i production order j;
a correlation parameter;
pijis the unit production quote for order j for plant i;
fiis the total fixed cost of production for plant i;
bijis the capacity, i.e., maximum production, of plant i production order j;
dijis the delivery delay rate of plant i for order j;
ALiis the lowest order number acceptable for candidate plant i;
AUiis the highest order quantity acceptable for candidate plant i;
or/and, taking the minimum cost as the optimization target, namely, minimizing the sum of the fixed cost of the factory and the product cost in the production process to obtain the objective function
The fitness function is as follows
Wherein f represents an objective function value of the individual; fmaxThe maximum objective function value that has occurred in the evolution process so far; namely, chromosomes with high fitness are preferentially inherited to the next generation;
or/and setting constraint conditions in the establishment of the order optimization model on the basis of the objective function, wherein the constraint conditions comprise bij、dij、ALi、AUiOne or more of (a).
In a preferred embodiment of the present invention, the crossover in the crossover operation is the most important operator in the genetic algorithm for disseminating the order cut point in the order allocation chromosome encoding the excellent order cut point gene or order cut point gene fragment in the order cut point chromosome; the crossover operator selects any combination point for each group of two order distribution chromosomes participating in crossover operation according to the crossover probability, and in order to ensure that the number of the orders distributed by each factory is not negative, namely to prevent generation of unqualified order distribution chromosome order cut-off point coding chromosomes, the combination point of each group of two order distribution chromosomes participating in crossover operation in the crossover process keeps that the numerical value of the order cut-off point gene positioned at the front position is smaller than the numerical value of the order cut-off point gene positioned at the back position; the order segmentation point code chromosomes in the order distribution chromosomes after the combination point are at least partially interchanged to generate two new order distribution chromosomes.
In a preferred embodiment of the present invention, the mutation in the mutation operation is an order cut point code chromosome that is applied individually to each order allocation chromosome after the crossover operation, helping to extend the search process to the entire solution space; the mutation operation randomly transforms each order cut point gene in order cut point code chromosomes of order distribution chromosomes with a small mutation probability; randomly assigning values according to a method for assigning values to order dividing point code chromosomes of order distribution chromosomes again in initialization during mutation to generate new order dividing point code chromosomes of order distribution chromosomes; the mutation operation adopts substitution mutation, namely, an order segmentation point gene position on an order segmentation point coding chromosome of an order distribution chromosome is randomly selected, and then a nonnegative integer which is not more than the maximum production capacity of a corresponding factory after cross point decoding is randomly generated to replace the original order segmentation point gene on the position.
In a preferred embodiment of the present invention, the selection operation determines which order segmentation point code chromosomes of the order distribution chromosomes are selected by the selection operator to perform an alternating calculation of order segmentation point code chromosomes of a new order distribution chromosome in the next generation population; the selection operator can employ one or more of a roulette selection method, a random traversal sampling method, and a tournament selection method.
In a preferred embodiment of the invention, the roulette selection method accelerates the convergence of the algorithm by selecting a greater probability with a smaller offset; a roulette selection method is adopted; defining the number of times of alternation as a population scale, wherein the population scale is z, and in the roulette selection method, in order to prevent the genetic algorithm from falling into the local optimal solution, the value of z is an integer of 100-200, and then the probability that the order distribution chromosome c is selected is
Among them, Fitness
cAnd allocating fitness function values of the chromosomes c to the orders, wherein the population size z is the total number of the population.
In a preferred embodiment of the invention, the order distribution system is used in the textile and clothing industry internet, and the computer is provided with an order distribution model established by an order distribution method used in the textile and clothing industry internet.
The invention solves the defects existing in the background technology, and has the beneficial effects that:
the invention provides a genetic algorithm based on order allocation, which is used for modeling the manufacturing capacity of all enterprises entering a platform, formulating a reasonable objective function by analyzing parameters and constraints of an Internet platform in the textile and clothing industry and finally providing an order allocation scheme with actual production and relatively optimal comprehensive performance.
Detailed Description
The invention will now be described in further detail with reference to the accompanying drawings and examples, which are simplified schematic drawings and illustrate only the basic structure of the invention in a schematic manner, and thus show only the constituents relevant to the invention.
It should be noted that, if directional indications (such as up, down, bottom, top, etc.) are involved in the embodiment of the present invention, the directional indications are only used for explaining the relative position relationship, motion situation, etc. of each component in a certain posture, and if the certain posture is changed, the directional indications are changed accordingly. The terms "first", "second" and "first" are used for descriptive purposes only and are not to be construed as indicating or implying relative importance or implicitly indicating the number of technical features indicated. Thus, a feature defined as "first" or "second" may explicitly or implicitly include one or more of that feature. Unless expressly stated or limited otherwise, the terms "disposed," "connected," and "connected" are intended to be inclusive and mean, for example, that there may be a fixed connection, a removable connection, or an integral connection; they may be connected directly or indirectly through intervening media, or they may be interconnected between two elements. The specific meanings of the above terms in the present invention can be understood in specific cases to those skilled in the art.
Example one
As shown in fig. 3, an order distribution method for use in the textile garment industry internet comprises the steps of;
step S1, m orders, wherein m is selected from integers which are more than or equal to 1; and (3) disassembling the orders, wherein the basic unit capable of disassembling the clothes in each order is a plurality of products, and the number of each product is m.
Specifically, from the perspective of processing habits, the detachable basic unit of the garment should be an upper garment and a lower garment. Therefore, the production task of the split order mainly comprises two products, namely m pieces of upper clothes and m pieces of lower clothes.
Step S2, there are n factories in the textile and clothing industry Internet platform, wherein n is selected from integers of 1 or more, each factory has different production parameters for the production of different products, and the production parameters include one or more of quotation, fixed cost, maximum production and delivery delay rate; and determining the constraint conditions and the objective function of the model according to the production parameters of the factory and the optimization target of order distribution.
In step S3, m orders are assigned to n factories by a set genetic algorithm. Specifically, the principle of order allocation is to enable orders to be delivered on schedule and to equalize the factory load within the platform to achieve relatively optimal order allocation goals.
Further, in step S2, selecting an optimization target according to the actual order production requirement, setting a fitness function according to the production parameters of the plant and the characteristics of the genetic algorithm through the target function, and calculating the individual fitness; in step S3, iteration of the population is performed through one or more of selection operation, crossover operation, and mutation operation in the genetic algorithm, and when a termination condition is satisfied, the selected optimal order allocation plan is output. Specifically, the optimization objective includes minimizing order costs. Populations refer to sets of chromosomes, and the sets of chromosomes are updated at different stages or steps of the genetic algorithm, i.e., after different numbers of iterations in the genetic algorithm.
Further, setting the genetic algorithm includes the following steps;
step a, n factories and m orders are arranged in a known platform, and each order comprises a plurality of products; each gene position corresponds to n-1 cut points from 0 to m, the order is divided into n intervals, the number of the product orders obtained by each factory is obtained after decoding, and the length of the order distribution chromosome is 2 (n-1).
dpiDenotes the ith tangent point position, i is 1,2, n-1, then dp is [ dp ]1,dp2,...,dpi,...,dpn-1]Representing an order cut point decoding chromosome; the order dividing point decodes the chromosome to obtain the corresponding order quantity [ dp ] of each factory1,dp2-dp1,...,dpi-dpi-1,...,m-dpn-1]。
For example, in a particular embodiment, when m orders are 200, the orders are divided into tops and bottoms. The upper loading cut-out points 35, 77, 144 in fig. 2. The lower garment of fig. 2 has cut points 3, 54, 172. Each group of top loading dividing points and bottom loading dividing points form order dividing points of a factory. Decoding the coding example of the segmentation point chromosome in the figure 2 to form a decoding example of the segmentation point chromosome in the figure 1, and obtaining the number of orders corresponding to different factory allocations; namely the upper assembly of points 35, 42, 67, 56 of fig. 1. The lower garment of fig. 1 has cut points 3, 51, 118, 28. The single upper binding order number and the single lower binding order number form the whole order information of a factory.
B, determining a fitness function, wherein the fitness function is used for evaluating whether the order dividing point coding chromosome of the order distribution chromosome is inherited into an evaluation function of the next generation, and the fitness function is a basis for executing the eligibility and the rejection of a genetic algorithm; the order dividing point coding chromosome of the order distribution chromosome with high fitness is preferentially inherited to the next generation; and establishing an order optimization model by combining an objective function or/and a fitness function with variables.
Establishing an order optimization model by using variables; comprises parameters or/and related parameters, wherein the parameters comprise i, j and yi、xij、aijOne or more of; the relevant parameters include pij、fi、bij、dij、ALi、AUiOne or more of;
i is a factory serial number, i is taken from a natural number not greater than n; n at this time is the total number of plants;
j is order number, and j is taken from a natural number not greater than m; m at this time is the number of orders;
yiis a variable of 0-1, when the platform selection factory i is responsible for the production of the order, y i1 is ═ 1; otherwise, yi=0;
xijIs a variable of 0 to 1, x when factory i produces order j ij1 is ═ 1; otherwise, xij=0;
aijIs the task volume of factory i production order j;
a correlation parameter;
pijis the unit production quote for order j for plant i;
fiis the total fixed cost of production for plant i;
bijis the capacity, i.e., maximum production, of plant i production order j;
dijis the delivery delay rate of plant i for order j;
ALiis the lowest order number acceptable for candidate plant i;
AUiis the highest order quantity acceptable by the candidate factory i.
The minimum cost is taken as an optimization target, namely the sum of the fixed cost of a factory and the product cost in the production process is minimized to obtain an objective function
Wherein f represents an objective function value of the individual; fmaxThe maximum objective function value that has occurred in the evolution process so far; i.e. chromosome preference with high fitnessAnd is inherited to the next generation.
Further, on the basis of the objective function, setting a constraint condition in the establishment of the order optimization model, wherein the constraint condition comprises b
ij、d
ij、AL
i、AU
iOne or more of (a). Further, in this embodiment, the constraint condition is; condition a1, formula b
ij≥a
ij(ii) a I.e., to ensure that the plant capacity load is met, the plant is able to limit within a stage (maximum capacity ≧ production supply) for each product. Condition b1, formula a
ij≥0,
y i0 or 1, x
ij0 or 1; the value ranges of the decision variables are shown to be non-negative constraint of the yield and 0-1 variable constraint respectively. Condition c1, formula a
ij∈[AL
i,AU
i](ii) a I.e. to ensure that the number of orders assigned to plant i is within its acceptable range. Condition d1, equation
That is, a certain proportion of products are guaranteed to be delivered on time (the delivery rate on time is not less than 90%).
And c, sequentially carrying out selection operation, cross operation and mutation operation in the genetic algorithm to iterate the population, and outputting the selected optimal order distribution scheme after the termination condition is met.
The crossover in the crossover operation is the most important operator in the genetic algorithm and is used for disseminating the order cut point genes or order cut point gene segments which are excellent in the order cut point coding chromosome of the order distribution chromosome; the crossover operator selects any combination point for the order cut point code chromosomes of the two order distribution chromosomes of each group participating in the crossover operation according to the crossover probability, and in order to ensure that the number of the orders distributed by each factory is not negative, namely, the order cut point code chromosomes of the unqualified order distribution chromosomes are prevented from being generated, the combination point of the order cut point code chromosomes of the two order distribution chromosomes of each group participating in the crossover operation in the population keeps that the numerical value of the order cut point gene positioned at the front position is smaller than the numerical value of the order cut point gene positioned at the back position in the crossover process; the order segmentation point code chromosomes of the order distribution chromosomes after the combination point are at least partially interchanged to generate order segmentation point code chromosomes of two new order distribution chromosomes.
The mutation in the mutation operation is an order cut point code chromosome that is individually applied to each order allocation chromosome after the crossover operation, contributing to the expansion of the search process to the entire solution space; the mutation operation randomly transforms each order cut point gene in order cut point code chromosomes of order distribution chromosomes with a small mutation probability; randomly assigning values according to an assignment method of re-assigning the order distribution chromosomes in the initialization during mutation to generate order segmentation point coding chromosomes of new order distribution chromosomes; the mutation operation adopts substitution mutation, namely, an order segmentation point gene position on an order segmentation point coding chromosome of an order distribution chromosome is randomly selected, and then a nonnegative integer which is not more than the maximum production capacity of a corresponding factory after cross point decoding is randomly generated to replace the original order segmentation point gene on the position.
The selection operation can decide which order dividing point code chromosomes of the order distribution chromosomes are selected through a selection operator to carry out the alternation calculation of new order distribution chromosomes in the next generation of population; the selection operator can employ one or more of a roulette selection method, a random traversal sampling method, and a tournament selection method. The embodiment adopts a roulette selection method, and the smaller the offset in the roulette selection method, the higher the probability of selection, and the convergence of the algorithm can be accelerated; adopting a roulette selection method; defining the number of times of alternation as a population scale, wherein the population scale is z, and in the roulette selection method, in order to prevent the genetic algorithm from falling into the local optimal solution, the value of z is an integer of 100-200, and then the probability that the order distribution chromosome c is selected is
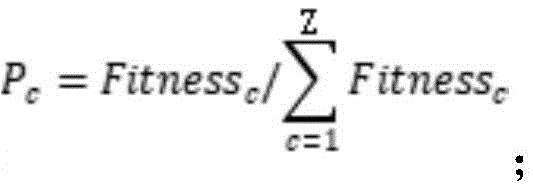
Among them, Fitness
cAnd allocating fitness function values of the chromosomes c to the orders, wherein the population size z is the total number of the population.
Step d, terminating conditions; and when the actual iteration times of the algorithm are more than or equal to the preset maximum iteration times, stopping the algorithm, and obtaining an optimal solution, namely a relatively optimal order distribution scheme required by the textile garment industry Internet platform in the multiple order distribution schemes.
Example two
An order distribution system used in the textile and clothing industry internet is provided, and an order distribution model established by the order distribution method used in the textile and clothing industry internet is arranged in a computer.
The working principle is as follows: and modeling the manufacturing capability of all enterprises entering the platform, formulating a reasonable objective function by analyzing parameters and constraints of the Internet platform of the textile and clothing industry, and finally providing an order distribution scheme with relatively optimal comprehensive performance in actual production.
In light of the foregoing description of the preferred embodiment of the present invention, it is to be understood that various changes and modifications may be made by one skilled in the art without departing from the spirit and scope of the invention. The technical scope of the present invention is not limited to the content of the specification, and must be determined according to the scope of the claims.