High-resolution reconstruction of flow-field data from low-resolution and noisy measurements is of interest due to the prevalence of such problems in experimental fluid mechanics, where the measurement data are in general sparse, incomplete and noisy. Deep-learning approaches have been shown suitable for such super-resolution tasks. However, a high number of high-resolution examples is needed, which may not be available for many cases. Moreover, the obtained predictions may lack in complying with the physical principles, e.g. mass and momentum conservation. Physics-informed deep learning provides frameworks for integrating data and physical laws for learning. In this study, we apply physics-informed neural networks (PINNs) for super-resolution of flow-field data both in time and space from a limited set of noisy measurements without having any high-resolution reference data. Our objective is to obtain a continuous solution of the problem, providing a physically-consistent prediction at any point in the solution domain. We demonstrate the applicability of PINNs for the super-resolution of flow-field data in time and space through three canonical cases: Burgers' equation, two-dimensional vortex shedding behind a circular cylinder and the minimal turbulent channel flow. The robustness of the models is also investigated by adding synthetic Gaussian noise. Furthermore, we show the capabilities of PINNs to improve the resolution and reduce the noise in a real experimental dataset consisting of hot-wire-anemometry measurements. Our results show the adequate capabilities of PINNs in the context of data augmentation for experiments in fluid mechanics.
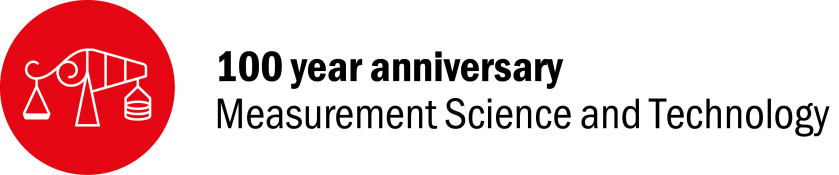

Purpose-led Publishing is a coalition of three not-for-profit publishers in the field of physical sciences: AIP Publishing, the American Physical Society and IOP Publishing.
Together, as publishers that will always put purpose above profit, we have defined a set of industry standards that underpin high-quality, ethical scholarly communications.
We are proudly declaring that science is our only shareholder.
ISSN: 1361-6501
Launched in 1923 Measurement Science and Technology was the world's first scientific instrumentation and measurement journal and the first research journal produced by the Institute of Physics. It covers all aspects of the theory, practice and application of measurement, instrumentation and sensing across science and engineering.
Hamidreza Eivazi et al 2024 Meas. Sci. Technol. 35 075303
Petr Klapetek et al 2024 Meas. Sci. Technol. 35 125026
Image stitching is a technique that can significantly enlarge the scan area of scanning probe microscope (SPM) images. It is also the most commonly used method to cover large areas in high-speed SPM. In this paper, we provide details on stitching algorithms developed specifically to mitigate the effects of SPM error sources, namely the presence of scanner non-flatness. Using both synthetic data and flat samples we analyse the potential uncertainty contributions related to stitching, showing that the drift and line mismatch are the dominant sources of uncertainty. We also present the 'flatten base' algorithm that can significantly improve the stitched data results, at the cost of losing the large area form information about the sample.
Eduardo Nunes dos Santos et al 2024 Meas. Sci. Technol. 35 125302
In the oil and gas sector, the design of monitoring equipment usually prioritizes durability and long-term reliability. However, such equipment does not provide resolution for scientific research, where capturing transient and dynamic events is crucial to enhancing flow understanding. This work describes the development of a capacitive sensor system optimized for phase fraction measurements in oil–gas industrial environments. The sensor features high sensitivity and temporal resolution to meet flow measurement investigative requirements. The measurement technique is based on the electrical capacitance variations of the flowing media and was validated with reference equipment. Six sensors were deployed across multiple test stations to analyze the slug flow regime and its evolution along the pipe. The data collected from these experiments were processed, and flow parameters were compared with a model that describes the elongated bubble shape found in the slug flow pattern. Results show a good agreement between the experimental data and the model, validating its capability to track the fast-changing phases of multiphase flow. The uncertainty analysis revealed a maximum absolute uncertainty of 1.41% for the gas fraction measurements. Further, the gas flow rate was evaluated with a good agreement against the reference gas flow meter, ensuring the sensor's reliability in dynamic multiphase flow environments. By providing accurate experimental data from real-world industrial conditions, the developed sensor can significantly enhance the precision of flow models, thereby improving the understanding of complex flow phenomena.
Ruidong Xue et al 2025 Meas. Sci. Technol. 36 012002
This literature review investigates the integration of machine learning (ML) into optical metrology, unveiling enhancements in both efficiency and effectiveness of measurement processes. With a focus on phase demodulation, unwrapping, and phase-to-height conversion, the review highlights how ML algorithms have transformed traditional optical metrology techniques, offering improved speed, accuracy, and data processing capabilities. Efficiency improvements are underscored by advancements in data generation, intelligent sampling, and processing strategies, where ML algorithms have accelerated the metrological evaluations. Effectiveness is enhanced in measurement precision, with ML providing robust solutions to complex pattern recognition and noise reduction challenges. Additionally, the role of parallel computing using graphics processing units and field programmable gate arrays is emphasised, showcasing their importance in supporting the computationally intensive ML algorithms for real-time processing. This review culminates in identifying future research directions, emphasising the potential of advanced ML models and broader applications within optical metrology. Through this investigation, the review articulates a future where optical metrology, empowered by ML, achieves improved levels of operational efficiency and effectiveness.
Andrew James Murray 2024 Meas. Sci. Technol. 35 127002
Single quantum particle detectors (e.g. photo-multiplier tubes and electron multipliers) produce ns-output pulses that time-correlate to particles that enter them. The detector output pulses usually require amplification at source, before being sent to counting electronics for analysis. A laser-based transport system is detailed here that converts the detector output into light pulses that are injected into single mode fibre. Pulse delivery is then by fibre, so that electrical interference from the laboratory environment and earth grounding loops are eliminated. A fast photodiode and differential amplifier converts the signal exiting the fibre into a voltage pulse for subsequent timing experiments.
Zijian Qiao and Zhengrong Pan 2015 Meas. Sci. Technol. 26 085014
Aiming at solving the existing sharp problems by using singular value decomposition (SVD) in the fault diagnosis of rolling bearings, such as the determination of the delay step k for creating the Hankel matrix and selection of effective singular values, the present study proposes a novel adaptive SVD method for fault feature detection based on the correlation coefficient by analyzing the principles of the SVD method. This proposed method achieves not only the optimal determination of the delay step k by means of the absolute value of the autocorrelation function sequence of the collected vibration signal, but also the adaptive selection of effective singular values using the index
corresponding to useful component signals including weak fault information to detect weak fault signals for rolling bearings, especially weak impulse signals. The effectiveness of this method has been verified by contrastive results between the proposed method and traditional SVD, even using the wavelet-based method through simulated experiments. Finally, the proposed method has been applied to fault diagnosis for a deep-groove ball bearing in which a single point fault located on either the inner or outer race of rolling bearings is obtained successfully. Therefore, it can be stated that the proposed method is of great practical value in engineering applications.
Simon Laflamme et al 2023 Meas. Sci. Technol. 34 093001
Structural health monitoring (SHM) is the automation of the condition assessment process of an engineered system. When applied to geometrically large components or structures, such as those found in civil and aerospace infrastructure and systems, a critical challenge is in designing the sensing solution that could yield actionable information. This is a difficult task to conduct cost-effectively, because of the large surfaces under consideration and the localized nature of typical defects and damages. There have been significant research efforts in empowering conventional measurement technologies for applications to SHM in order to improve performance of the condition assessment process. Yet, the field implementation of these SHM solutions is still in its infancy, attributable to various economic and technical challenges. The objective of this Roadmap publication is to discuss modern measurement technologies that were developed for SHM purposes, along with their associated challenges and opportunities, and to provide a path to research and development efforts that could yield impactful field applications. The Roadmap is organized into four sections: distributed embedded sensing systems, distributed surface sensing systems, multifunctional materials, and remote sensing. Recognizing that many measurement technologies may overlap between sections, we define distributed sensing solutions as those that involve or imply the utilization of numbers of sensors geometrically organized within (embedded) or over (surface) the monitored component or system. Multi-functional materials are sensing solutions that combine multiple capabilities, for example those also serving structural functions. Remote sensing are solutions that are contactless, for example cell phones, drones, and satellites. It also includes the notion of remotely controlled robots.
Martin Kögler and Bryan Heilala 2020 Meas. Sci. Technol. 32 012002
Time-gated (TG) Raman spectroscopy (RS) has been shown to be an effective technical solution for the major problem whereby sample-induced fluorescence masks the Raman signal during spectral detection. Technical methods of fluorescence rejection have come a long way since the early implementations of large and expensive laboratory equipment, such as the optical Kerr gate. Today, more affordable small sized options are available. These improvements are largely due to advances in the production of spectroscopic and electronic components, leading to the reduction of device complexity and costs. An integral part of TG Raman spectroscopy is the temporally precise synchronization (picosecond range) between the pulsed laser excitation source and the sensitive and fast detector. The detector is able to collect the Raman signal during the short laser pulses, while fluorescence emission, which has a longer delay, is rejected during the detector dead-time. TG Raman is also resistant against ambient light as well as thermal emissions, due to its short measurement duty cycle.
In recent years, the focus in the study of ultra-sensitive and fast detectors has been on gated and intensified charge coupled devices (ICCDs), or on CMOS single-photon avalanche diode (SPAD) arrays, which are also suitable for performing TG RS. SPAD arrays have the advantage of being even more sensitive, with better temporal resolution compared to gated CCDs, and without the requirement for excessive detector cooling. This review aims to provide an overview of TG Raman from early to recent developments, its applications and extensions.
Jiaqi Wang et al 2024 Meas. Sci. Technol. 35 126313
Aiming at the problem of low measurement resolution and poor tracking accuracy in high-frequency surface wave radar (HFSWR) tracking field, a tracking method that combines the heading constraint filter and extreme learning machine (ELM) is proposed. Compared with the existing research, the innovation of this study lies in proposing a two-stage tracking method based on the short-term stable state of the target, and similarity of long-term trajectory. Firstly, the heading constraint information is introduced into the estimator and improve the estimation accuracy. In multi-target tracking scenarios, many tracklets can be obtained. Then, the ELM learns robust features of tracklets to achieve the trajectory segment classification from the same target. This method can be widely applied to long-term tracking of cargo or passenger ships with fixed destinations, significantly improving tracking performance by utilizing implicit domain knowledge without additional information. The actual data of this paper comes from HFSWR on Bohai Bay. Both the simulation and actual measurement experiments show that the proposed cascaded tracking method achieves long-term continuous tracking, and generates more complete trajectories. Moreover, the proposed Extended Kalman filter based on pseudo-measurement and intercept parameters exhibits better tracking stability and accuracy in limited scan steps. This study provides new ideas and methods for improving the tracking performance of special targets in the HFSWR field by utilizing motion features.
Tim A van Kempen et al 2024 Meas. Sci. Technol. 35 125805
The TROPOMI-SWIR HgCdTe detector on the Sentinel-5 Precursor mission has been performing in-orbit measurements of molecular absorption in Earth's atmosphere since its launch in October 2017. In its polar orbit the detector is continuously exposed to potentially harmful energetic particles. Calibration measurements taken during the eclipse are used to inspect the performance of this detector. This paper explores the in-orbit degradation of the HgCdTe detector. After five years, the detector is still performing within specifications, even though pixels are continuously hit by cosmic radiation. The bulk of the impacts have no lasting effects, and most of the damaged pixels (95%) appear to recover on the order of a few days to several months, attributed to a slow spontaneous recovery of defects in the HgCdTe detector material. This is observed at the operational temperature of 140 K. The distribution of the observed recovery times has a mean around nine days with a significant tail towards several months. Pixels that have degraded have a significant probability to degrade again. The location of faulty pixels follows a Poissonian distribution across the detector. No new clusters have appeared, revealing that impacts are dominated by relatively low energetic protons and electrons. Due to the observed spontaneous recovery of pixels, the fraction of pixels meeting all quality requirements in the nominal operations phase has always been over 98.7%. The observed performance of the TROPOMI-SWIR detector in-flight impacts selection criteria of HgCdTe detectors for future space instrumentation.
Maximilian Dreisbach et al 2025 Meas. Sci. Technol. 36 015304
The present work introduces a deep learning approach for the three-dimensional reconstruction of the spatio-temporal dynamics of the gas–liquid interface on the basis of monocular images obtained via optical measurement techniques. The method is tested and evaluated at the example of liquid droplets impacting on structured solid substrates. The droplet dynamics are captured through high-speed imaging in an extended shadowgraphy setup with additional glare points from lateral light sources that encode further three-dimensional information of the gas–liquid interface in the images. A neural network is trained for the physically correct reconstruction of the droplet dynamics on a labeled dataset generated by synthetic image rendering on the basis of gas–liquid interface shapes obtained from direct numerical simulation. The employment of synthetic image rendering allows for the efficient generation of training data and circumvents the introduction of errors resulting from the inherent discrepancy of the droplet shapes between experiment and simulation. The accurate reconstruction of the three-dimensional shape of the gas–liquid interface during droplet impingement on the basis of images obtained in the experiment demonstrates the practicality of the presented approach based on neural networks and synthetic training data generation. The introduction of glare points from lateral light sources in the experiments is shown to improve the reconstruction accuracy, which indicates that the neural network learns to leverage the additional three-dimensional information encoded in the images for a more accurate depth estimation. By the successful reconstruction of obscured areas in the input images, it is demonstrated that the neural network has the capability to learn a physically correct interpolation of missing data from the numerical simulation. Furthermore, the physically reasonable reconstruction of unknown gas–liquid interface shapes for drop impact regimes that were not contained in the training dataset indicates that the neural network learned a versatile model of the involved two-phase flow phenomena during droplet impingement.
Lieping Zhang et al 2025 Meas. Sci. Technol. 36 016321
In a mobile sensor network, a traditional positioning algorithm is unable to locate unknown nodes when losing anchor positions caused by communication interference. To solve this problem, an improved DV-Hop algorithm based on a geometric Brownian motion (GBM) model was proposed including two main stages: location of sink node (LSN) and location of blind node (LBN). In the LSN stage, if the signal transmission of anchors is normal, the GBM model records the moving positions of the anchors. If not, the GBM model predicts the estimated average positions of the anchors using recorded data. Then, the trial count of the GBM model is optimized to further improve the prediction accuracy and computational overhead. In the LBN stage, the unknown nodes' positions are obtained by the DV-Hop algorithm. In a traditional DV-Hop algorithm, the approximate minimum hop number and average hop distance may lead to huge deviation between true position and estimated position. To improve the positioning accuracy in the LBN stage, the strategies of multi-communication radius and hop distance weighting were adopted. The simulation results demonstrated that the proposed algorithm has the capability to resist communication interference and adaptability at different node speeds , maintaining a relatively high accuracy in locating unknown nodes.
Zhu Xiaoxun et al 2025 Meas. Sci. Technol. 36 015420
Anomaly detection (AD) plays a crucial role in various fields, from industrial defect inspection to geological detection. However, traditional approaches often struggle with insufficient discriminability and an inability to generalize to unseen anomalies. These limitations stem from the practical difficulty in gathering a comprehensive set of anomalies and the tendency to overlook anomalous instances in favor of normal samples. To address these challenges, we propose a novel Dynamic AD Enhancement Framework, integrating three key innovations: (1) SaliencyAug: An adaptive saliency-guided augmentation method that generates realistic pseudo-samples to enhance learning of rare anomalies, improving model generalization. (2) DynAB: A dynamic attention block that achieves effective multi-level feature fusion while minimizing redundant information, enhancing detection accuracy. (3) DualOM: A dual-head optimization module which employs separate heads for normal and anomalous sample learning, creating more explicit and discriminative decision boundaries. Extensive experiments across multiple real-world datasets demonstrate our framework's superior performance in detecting a wide range of anomalies, demonstrating 2.4% improvement over state-of-the-art methods.
Xiaoning Zhao et al 2025 Meas. Sci. Technol. 36 012003
Real-time control systems (RTCSs) have become an indispensable part of modern industry, finding widespread applications in fields such as robotics, intelligent manufacturing and transportation. However, these systems face significant challenges, including complex nonlinear dynamics, uncertainties and various constraints. These challenges result in weakened disturbance rejection and reduced adaptability, which make it difficult to meet increasingly stringent performance requirements. In fact, RTCSs generate a large amount of data, which presents an important opportunity to enhance control effectiveness. Machine learning, with its efficiency in extracting valuable information from big data, holds significant potential for applications in RTCSs. Exploring the applications of machine learning in RTCSs is of great importance for guiding scientific research and industrial production. This paper first analyzes the challenges currently faced by RTCSs, elucidating the motivation for integrating machine learning into these systems. Subsequently, it discusses the applications of machine learning in RTCSs from various aspects, including system identification, controller design and optimization, fault diagnosis and tolerance, and perception. The research indicates that data-driven machine learning methods exhibit significant advantages in addressing the multivariable coupling characteristics of complex nonlinear systems, as well as the uncertainties arising from environmental disturbances and faults, thereby effectively enhancing the system's flexibility and robustness. However, compared to traditional methods, the applications of machine learning also faces issues such as poor model interpretability, high computational requirements leading to insufficient real-time performance, and a strong dependency on high-quality data. This paper discusses these challenges and proposes potential future research directions.
Dexu Xiao et al 2025 Meas. Sci. Technol. 36 015210
Point cloud ground segmentation is a key preprocessing task in mobile laser scanning (MLS)-based measurement and sensing. However, ground segmentation currently faces major challenges such as diverse ground morphology, sparse point cloud data, and interference from reflection noise. Meanwhile, since the existing principal component analysis-based ground plane fitting methods lack the judgment of iterative convergence and automatic correction of non-ground plane fitting results, this not only leads to unnecessary computational overhead, but also affects the accuracy of ground segmentation. To address these issues, this paper proposes a three-stage MLS point cloud ground segmentation method based on ground plane fitting, called GPF-Plus. This method adopts a three-stage strategy based on ground plane fitting to achieve ground segmentation, which is able to effectively deal with the challenges of various terrains. Firstly, the initial ground segmentation of the original point cloud is performed to quickly produce a coarse segmentation result. Secondly, the false negative points extraction is performed to improve the recall. Finally, the false positive points extraction is performed to improve the precision. At the same time, the infinite polar grid model is used to divide the point cloud, which reduces the number of grids and effectively alleviates the problem caused by point cloud sparsity. The reflection noise removal mechanism is introduced to enhance the robustness to reflection noise. In addition, the improved ground plane fitting improves the accuracy and speed of ground plane fitting. In this paper, experimental validation is carried out using the SemanticKITTI dataset, the SimKITTI32 dataset, and the collected point clouds of the mine environment. Compared with the state-of-the-art methods, GPF-Plus has excellent accuracy, real-time performance and robustness, and has high application potential in the field of measurement and sensing.
Xiaoning Zhao et al 2025 Meas. Sci. Technol. 36 012003
Real-time control systems (RTCSs) have become an indispensable part of modern industry, finding widespread applications in fields such as robotics, intelligent manufacturing and transportation. However, these systems face significant challenges, including complex nonlinear dynamics, uncertainties and various constraints. These challenges result in weakened disturbance rejection and reduced adaptability, which make it difficult to meet increasingly stringent performance requirements. In fact, RTCSs generate a large amount of data, which presents an important opportunity to enhance control effectiveness. Machine learning, with its efficiency in extracting valuable information from big data, holds significant potential for applications in RTCSs. Exploring the applications of machine learning in RTCSs is of great importance for guiding scientific research and industrial production. This paper first analyzes the challenges currently faced by RTCSs, elucidating the motivation for integrating machine learning into these systems. Subsequently, it discusses the applications of machine learning in RTCSs from various aspects, including system identification, controller design and optimization, fault diagnosis and tolerance, and perception. The research indicates that data-driven machine learning methods exhibit significant advantages in addressing the multivariable coupling characteristics of complex nonlinear systems, as well as the uncertainties arising from environmental disturbances and faults, thereby effectively enhancing the system's flexibility and robustness. However, compared to traditional methods, the applications of machine learning also faces issues such as poor model interpretability, high computational requirements leading to insufficient real-time performance, and a strong dependency on high-quality data. This paper discusses these challenges and proposes potential future research directions.
Ruidong Xue et al 2025 Meas. Sci. Technol. 36 012002
This literature review investigates the integration of machine learning (ML) into optical metrology, unveiling enhancements in both efficiency and effectiveness of measurement processes. With a focus on phase demodulation, unwrapping, and phase-to-height conversion, the review highlights how ML algorithms have transformed traditional optical metrology techniques, offering improved speed, accuracy, and data processing capabilities. Efficiency improvements are underscored by advancements in data generation, intelligent sampling, and processing strategies, where ML algorithms have accelerated the metrological evaluations. Effectiveness is enhanced in measurement precision, with ML providing robust solutions to complex pattern recognition and noise reduction challenges. Additionally, the role of parallel computing using graphics processing units and field programmable gate arrays is emphasised, showcasing their importance in supporting the computationally intensive ML algorithms for real-time processing. This review culminates in identifying future research directions, emphasising the potential of advanced ML models and broader applications within optical metrology. Through this investigation, the review articulates a future where optical metrology, empowered by ML, achieves improved levels of operational efficiency and effectiveness.
Ahmet Koca et al 2025 Meas. Sci. Technol. 36 012001
Despite ongoing improvements and optimisation efforts, the powder bed fusion (PBF) process continues to face challenges related to repeatability, robustness, and stability. These challenges can lead to the formation of microscale surface impurities on each layer, such as balling, spatter and surface pores, which can adversely affect the overall quality of the final part. The layer-by-layer fabrication approach in PBF offers an opportunity to assess fabrication quality in real-time by detecting these impurities at each layer during the manufacturing process through in-situ sensing methods. With advancements in sensing and computing technologies, there has been a significant increase in studies focused on developing in-situ methods for the real-time detection of surface impurities and feedback mechanisms. However, it is necessary to understand the effectiveness and capability of these in-situ methods in detecting microscale surface impurities, as well as to evaluate their potential advantages, drawbacks, and the existing gaps in the literature. This study first summarises the common microscale surface impurities and their potential impacts on part quality, including mechanical properties and surface finish. It then reviews the existing in-situ methods capable of detecting these microscale impurities, providing insights into the strengths and limitations of current techniques, and identifying gaps in the literature while suggesting directions for future research.
Zedong Ju et al 2024 Meas. Sci. Technol. 35 122004
In recent years, research on the intelligent fault diagnosis of rotating machinery has made remarkable progress, bringing considerable economic benefits to industrial production. However, in the industrial environment, the accuracy and stability of the diagnostic model face severe challenges due to the extremely limited fault data. Data augmentation methods have the capability to increase both the quantity and diversity of data without altering the key characteristics of the original data, which is particularly important for the development of intelligent fault diagnosis of rotating machinery under limited data conditions (IFD-RM-LDC). Despite the abundant achievements in research on data augmentation methods, there is a lack of systematic reviews and clear future development directions. Therefore, this paper systematically reviews and discusses data augmentation methods for IFD-RM-LDC. Firstly, existing data augmentation methods are categorized into three groups: synthetic minority over-sampling technique (SMOTE)-based methods, generative model-based methods, and data transformation-based methods. Then, these three methods are introduced in detail and discussed in depth: SMOTE-based methods synthesize new samples through a spatial interpolation strategy; generative model-based methods generate new samples according to the distribution characteristics of existing samples; data transformation-based methods generate new samples through a series of transformation operations. Finally, the challenges faced by current data augmentation methods, including their limitations in generalization, real-time performance, and interpretability, as well as the absence of robust evaluation metrics for generated samples, have been summarized, and potential solutions to address these issues have been explored.
Chao Yu et al 2024 Meas. Sci. Technol. 35 122003
Single-photon detectors (SPDs) are widely used in applications requiring extremely weak light detection. In the near-infrared region, SPDs based on InGaAs/InP single-photon avalanche diodes (SPADs) are the primary candidates for practical applications because of their small size, low cost and ease of operation. Driven by the escalating demands for quantum communication and lidar, the performance of InGaAs/InP SPDs has been continuously enhanced. This paper provides a comprehensive review of advances in InGaAs/InP SPDs over the past 10 years, including the investigation into SPAD structures and mechanisms, as well as emerging readout techniques for both gated and free-running mode SPDs. In addition, future prospects are also summarised.
Li et al
In the actual scenario of fault diagnosis based on deep learning, the diagnosis accuracy is often affected by the lack of fault state data, so the processing of imbalanced data is always a significant challenge. Generative Adversarial Networks(GAN) and Denoising Diffusion Probability Models(DDPM) are widely used for data augmentation. However, GAN often shows sensitivity and instability in the training process, and the sample generation speed of DDPM is slow due to the steps requiring multiple iterations–both of which are limiting factors. To solve these problems, we introduce the Generative Flow Network with Invertible 1×1 Convolutions (GLOW) into fault diagnosis. The GLOW model is optimized by maximum likelihood estimation and does not require multiple iterations to generate samples, avoiding the problems faced by GANandDDPM.Inorder to generate balanced data explicitly, we propose a condition GLOW(CGLOW) to provide class-balanced samples in real time throughout the framework. On the other hand, using the reversibility of CGLOW, we design an end-to-end fault diagnosis framework that is globally optimized to mitigate the decline in diagnostic accuracy caused by the separation of generation and diagnosis and simplify the steps of fault diagnosis. In addition, to accommodate the non-stationary characteristics of fault signals, we propose a new data transformation method to improve the feature mining ability of the model and the diagnostic accuracy. Finally, we conduct extensive experiments to validate the superiority of the proposed approach. The experimental results demonstrate that our method outperforms existing ones.
Cao et al
The development of high-performance fault diagnosis models for specific tasks requires substantial expertise. Neural Architecture Search (NAS) offers a promising solution, but most NAS methodologies are hampered by lengthy search durations and low efficiency, and few researchers have applied these methods within the fault diagnosis domain. This paper introduces a novel differentiable architecture search (DARTS) method tailored for constructing efficient fault diagnosis models for rotating machinery, designed to rapidly and effectively search for network models suitable for specific datasets. Specifically, this study constructs a completely new and advanced search space, incorporating various efficient, lightweight convolutional operations to reduce computational complexity. To enhance the stability of the differentiable network architecture search process and reduce fluctuations in model accuracy, this study proposes a novel Multi-scale Pyramid Squeeze Attention (MPSA) module. This module aids in the learning of richer multi-scale feature representations and adaptively recalibrates the weights of multi-dimensional channel attention. The proposed method was validated on two rotating machinery fault datasets, demonstrating superior performance compared to manually designed networks and general network search methods, with notably improved diagnostic effectiveness.
liu et al
Accurately determining the tested cable's total length is important in cable fault detection and localization. Therefore, an iterative method of relative propagation coefficients based on broadband impedance spectroscopy (BIS) is proposed to solve the actual length of the cable and a phase difference integral transform method for fault detection. First, the overall detection process framework is designed. Then, the cable distribution parameter model and the characteristics of the input impedance spectroscopy are analyzed. The calculation methods for determining the cable length and propagation coefficients are explained, followed by a demonstration of the fault localization process. Finally, the model LCR1000A impedance analyzer is used to measure cable length and actual faults in cables with lengths of 35 m, 100 m, and 500 m. The final fault location error is less than 0.67%, proving that the method can calculate the length of cables and various fault point locations.
Rigamonti et al
Neutron Emission Spectroscopy is an effective nuclear fusion plasma diagnostics technique for diagnosing the fuel ion populations on fusion plasma experiments. The state of the art 2.5 MeV neutron spectrometer for deuterium plasmas is based on the time of flight (TOF) technique, which however requires the development of large scale instruments. In the last years, compact detectors made with Chlorine based scintillators have been explored. In these instruments, neutron detection is based on the 35Cl(n,p)35S nuclear reaction, which results in a Gaussian peak in the recorded neutron energy spectrum. In this context, one option is offered by CLYC scintillators, which have the drawback of a limited counting rate capability (a few tens of kHz). Another option is offered by the LaCl3:(Ce) scintillators, which combine a comparable energy resolution and, most importantly, a faster signal (<1 μs) enabling measurements at higher counting rates. On the other hand, LaCl3 has a more challenging particle discrimination. The standard method based on pulse shape analysis provides a limited particle identification and poses restrictions in the counting rate capability of the instruments. An innovative particle identification algorithm based on Fourier Transforms has been developed providing higher accuracy and effectiveness. In this paper, we present the performance of a 2.5 MeV neutron spectrometer based on a LaCl3 scintillator in terms of pulse shape discrimination and energy resolution. Results are used to discuss their use for neutron spectroscopy applications in tokamak plasmas.
Yan et al
The pneumatic tube plays a critical role in the frequency response of the pressure measurement system, which also greatly affects the accuracy of the measurement data. This paper applied the Monte Carlo uncertainty qualification method to the pressure measurement tube system frequency response and studied the effects of four parameters, including tube length, radius, temperature, and source pressure, on uncertainty characteristics for a given tube system configuration. The reported results show the technique's applicability and flexibility and the limitations of GUM. It is found that the frequency response and corresponding uncertainty are not directly related to frequency but are closely related to the natural frequencies. Comparative experimental results show that the longer tube significantly reduces the system's natural frequencies and intensifies the phase lag. In addition, larger tube radius and source pressure result in larger amplitude response peaks. Closed to the natural frequencies, with the increase of the parameter, the uncertainties of frequency response value and the corresponding variation range increase for tube radius and source pressure while decreasing for temperature and tube length.
Maximilian Dreisbach et al 2025 Meas. Sci. Technol. 36 015304
The present work introduces a deep learning approach for the three-dimensional reconstruction of the spatio-temporal dynamics of the gas–liquid interface on the basis of monocular images obtained via optical measurement techniques. The method is tested and evaluated at the example of liquid droplets impacting on structured solid substrates. The droplet dynamics are captured through high-speed imaging in an extended shadowgraphy setup with additional glare points from lateral light sources that encode further three-dimensional information of the gas–liquid interface in the images. A neural network is trained for the physically correct reconstruction of the droplet dynamics on a labeled dataset generated by synthetic image rendering on the basis of gas–liquid interface shapes obtained from direct numerical simulation. The employment of synthetic image rendering allows for the efficient generation of training data and circumvents the introduction of errors resulting from the inherent discrepancy of the droplet shapes between experiment and simulation. The accurate reconstruction of the three-dimensional shape of the gas–liquid interface during droplet impingement on the basis of images obtained in the experiment demonstrates the practicality of the presented approach based on neural networks and synthetic training data generation. The introduction of glare points from lateral light sources in the experiments is shown to improve the reconstruction accuracy, which indicates that the neural network learns to leverage the additional three-dimensional information encoded in the images for a more accurate depth estimation. By the successful reconstruction of obscured areas in the input images, it is demonstrated that the neural network has the capability to learn a physically correct interpolation of missing data from the numerical simulation. Furthermore, the physically reasonable reconstruction of unknown gas–liquid interface shapes for drop impact regimes that were not contained in the training dataset indicates that the neural network learned a versatile model of the involved two-phase flow phenomena during droplet impingement.
Bryan E Schmidt et al 2025 Meas. Sci. Technol. 36 015303
The influence of several potential error sources and non-ideal experimental effects on the accuracy of a wavelet-based optical flow velocimetry (wOFV) method when applied to tracer particle images is evaluated using data from a series of synthetic flows. Out-of-plane particle displacements, severe image noise, laser sheet thickness reduction, and image intensity non-uniformity are shown to decrease the accuracy of wOFV in a similar manner to correlation-based particle image velocimetry (PIV). For the error sources tested, wOFV displays a similar or slightly increased sensitivity compared to PIV, but the wOFV results are still more accurate than PIV when the magnitude of the non-ideal effects remain within expected experimental bounds. For the majority of test cases, the results are significantly improved by using image pre-processing filters and the magnitude of improvement is consistent between wOFV and PIV. Flow divergence does not appear to have an appreciable effect on the accuracy of wOFV velocity estimation, even though the underlying fluid transport equation on which wOFV is based implicitly assumes that the motion is divergence-free. This is a significant finding for the broader applicability of planar velocimetry measurements using wOFV. Finally, it is noted that the accuracy of wOFV is not reduced notably in regions of the image between tracer particles, as long as the overall seeding density is not too sparse i.e. below 0.02 particles per pixel. This explicitly demonstrates that wOFV (when applied to particle images) yields an accurate whole field measurement, and not only at or adjacent to the discrete particle locations.
Xin Wang et al 2025 Meas. Sci. Technol. 36 016018
Contact forces between raceways and rolling elements of a bearing stand as crucial operational aspects defining the bearing's performance. While monitoring bearing load through load cells or strain gauges on the shaft or housing is feasible, it may not precisely reflect the distributed load transmitted by the rollers directly to the raceways. This paper introduces a method to calculate these contact forces, relying on a linear assumption correlating the loads to the measured strains on the outer race of a bearing. In our research, we conducted both static and dynamic tests on cylindrical roller bearings through simulations and experiments. We designed an experimental test rig to conduct both static and dynamic experiments and utilized FBG optical fiber sensors for contact force measurement in bearings due to their multiple advantages, including high sensitivity, resistance to corrosion and magnetic interference, and ease of installation on the bearing outer race due to their shape and size. Our findings indicate a consistent linear relationship between contact forces and strains measured on the bearing's outer race. Furthermore, we calculated the linear coefficient K values from three test groups: static tests under simulation study, static tests under experimental study, and dynamic tests under experimental study. The K values obtained from static tests (simulation and experimental studies) and dynamic tests (experimental study) align consistently. Following this, we calculated contact forces in both static and dynamic experiments by multiplying the measured strains with K. Our calculations resulted in an error percentage of less than 2% for static tests and below 5% for dynamic tests, highlighting the accuracy of our approach in determining these contact forces.
Davide Rigamonti et al 2024 Meas. Sci. Technol.
Neutron Emission Spectroscopy is an effective nuclear fusion plasma diagnostics technique for diagnosing the fuel ion populations on fusion plasma experiments. The state of the art 2.5 MeV neutron spectrometer for deuterium plasmas is based on the time of flight (TOF) technique, which however requires the development of large scale instruments. In the last years, compact detectors made with Chlorine based scintillators have been explored. In these instruments, neutron detection is based on the 35Cl(n,p)35S nuclear reaction, which results in a Gaussian peak in the recorded neutron energy spectrum. In this context, one option is offered by CLYC scintillators, which have the drawback of a limited counting rate capability (a few tens of kHz). Another option is offered by the LaCl3:(Ce) scintillators, which combine a comparable energy resolution and, most importantly, a faster signal (<1 μs) enabling measurements at higher counting rates. On the other hand, LaCl3 has a more challenging particle discrimination. The standard method based on pulse shape analysis provides a limited particle identification and poses restrictions in the counting rate capability of the instruments. An innovative particle identification algorithm based on Fourier Transforms has been developed providing higher accuracy and effectiveness. In this paper, we present the performance of a 2.5 MeV neutron spectrometer based on a LaCl3 scintillator in terms of pulse shape discrimination and energy resolution. Results are used to discuss their use for neutron spectroscopy applications in tokamak plasmas.
Minghui Yan et al 2024 Meas. Sci. Technol.
The pneumatic tube plays a critical role in the frequency response of the pressure measurement system, which also greatly affects the accuracy of the measurement data. This paper applied the Monte Carlo uncertainty qualification method to the pressure measurement tube system frequency response and studied the effects of four parameters, including tube length, radius, temperature, and source pressure, on uncertainty characteristics for a given tube system configuration. The reported results show the technique's applicability and flexibility and the limitations of GUM. It is found that the frequency response and corresponding uncertainty are not directly related to frequency but are closely related to the natural frequencies. Comparative experimental results show that the longer tube significantly reduces the system's natural frequencies and intensifies the phase lag. In addition, larger tube radius and source pressure result in larger amplitude response peaks. Closed to the natural frequencies, with the increase of the parameter, the uncertainties of frequency response value and the corresponding variation range increase for tube radius and source pressure while decreasing for temperature and tube length.
Tanbo Zhou et al 2025 Meas. Sci. Technol. 36 015302
Background oriented schlieren (BOS) is a non-intrusive optical method for measuring density gradients in a fluid flow based on variations of the local refractive index. The type of BOS optical system used, i.e. entocentric vs. telecentric, and the system design determine the accuracy and quality of the measurement. This work aims to optimize both types of optical systems to minimize the error for measurements of high-speed compressible turbulent boundary layers. Claims of the advantages offered by telecentric optical systems over entocentric systems are investigated, as well as the out-of-focus effects for types of systems. Numerical ray tracing simulations are performed using density fields from large eddy simulations (LES) of a Mach 2 turbulent boundary layer to generate synthetic but realistic BOS images. The results show that telecentric systems have lower overall error and less sensitivity. Contrary to recommendations by early BOS work, the best accuracy is achieved when density gradient object is placed outside of the depth of field of the optical system, for both entocentric and telecentric systems.
Yi Jiang et al 2025 Meas. Sci. Technol. 36 016317
The positioning technology based on ultra-wideband ranging has been widely applied in the field of indoor positioning due to its excellent localization capabilities. However, mixed line-of-sight (LOS) and non-LOS (NLOS) indoor environments severely constrain positioning accuracy. To address this issue, we propose an innovative algorithm based on the adaptive unscented Kalman filter (AUKF) and interactive multiple model (IMM), designed to significantly enhance positioning accuracy in mixed indoor environments by mitigating the impact of NLOS errors and inaccurate process noise. Firstly, recognizing the distinct characteristics of ranging errors in indoor environments, we develop LOS and NLOS ranging models separately. Based on these models, the unscented Kalman filters are constructed for LOS and NLOS environments to accurately simulate the mixed LOS/NLOS indoor environments. Secondly, determining the statistical characteristics of process noise is challenging, often leading to degraded filter performance. We address this issue by proposing an environment-based AUKF algorithm, which significantly enhances the robustness and accuracy of the positioning system. Finally, the environment-based AUKFs are integrated into the IMM framework to constrain NLOS errors and achieve precise positioning effectively. Simulations, open-source dataset validation and experimental results demonstrate that the proposed algorithm significantly enhances the accuracy and stability of mobile target positioning in mixed LOS/NLOS indoor environments.
Enrique Guzman and Brian Hoyle 2025 Meas. Sci. Technol. 36 010202
Fahad Bin Zahid et al 2024 Meas. Sci. Technol.
Novel Impact Synchronous Modal Analysis (ISMA) suffers from inefficient operation. Automated Phase Controlled Impact Device (APCID), a fully automated device, was developed to efficiently perform ISMA. However, actuator, support structure and power supply of the APCID makes it large and heavy, and unsuitable for commercial applications. APCID can be replaced with manual operation while still using its control but there is randomness in human behaviour by nature, which can greatly reduce the effectiveness of the APCID control scheme. A smart semi-automated device for imparting impacts is developed in this study which uses Brain Computer Interface (BCI) for predicting impact time prior to impact. Brainwaves are measured using a portable, wireless and low-cost Electroencephalogram (EEG) device. Using brainwaves, Machine Learning (ML) model is developed to predict impact time. ML model gave Mean Absolute Percentage Error (MAPE) of 7.5% and 8% in evaluation (offline testing) and in real-time testing, respectively, while predicting impact time prior to impact using brainwaves. When integrated with the control of APCID for performing ISMA, the ML model gave MAPE of 8.3% in real-time ISMA while predicting impact time prior to impact and adjusting APCID control for the upcoming impact accordingly. To demonstrate the effectiveness of EEG ML model in performing ISMA, modal testing was performed at 2 different operating speeds. The study is concluded by comparing the developed ISMA method with other ISMA methods. The BCI based device developed in this study for performing ISMA outranks other ISMA methods due to its performance, efficiency and practicality.
Bartosz Czesław Pruchnik et al 2024 Meas. Sci. Technol.
One of the most important limitations of the atomic force microscopy (AFM) is scanning speed, whose high values are required for contemporary high-resolution, long-range diagnostic applications. The measurement bandwidth of an AFM depends on several factors, but usually results from the time constant of the oscillating cantilever, which is correlated with its resonance frequency and quality factor. We propose a method to overcome this problem by performing the surface measurements when the cantilever is vibrating in higher eigenmodes. In this paper we demonstrate the application of active piezoresistive cantilevers operating in this mode. The active piezoresistive cantilever comprises a piezoresistive deflection sensor, a deflection actuator and a nanotip. It is a complete micro-electro-mechanical system (MEMS), ensuring the highest reliability of cantilever vibration control and detection. Higher eigenmode operations are usually difficult to implement as they usually result in lower deflection and lower sensitivity of the probe vibration deflection. Here we present an experimental modification of the structure of an active piezoresistive cantilever using focused ion beam (FIB) machining that mitigates both weaknesses. This has enabled the cantilever to scan the surface at a scanning rate of 10 lines/s with a maximum speed of 500 μm/s and a data acquisition rate of 10 kS/s, when the probe is vibrating at 380 kHz in the second eigenmode. We also describe a traceable calibration routine (based on analysis of the response of the piezoresistive detector, the output of the HeNe interferometer and precise control of the deflection actuator), together with the cantilever modification process and the development of the measurement setup. We show measurement results of dedicated calibration samples and silicon carbide crystal lattice references.