-
-
Notifications
You must be signed in to change notification settings - Fork 26k
[MRG] Speed up plot_digits_linkage.py example #21598 #21678
New issue
Have a question about this project? Sign up for a free GitHub account to open an issue and contact its maintainers and the community.
By clicking “Sign up for GitHub”, you agree to our terms of service and privacy statement. We’ll occasionally send you account related emails.
Already on GitHub? Sign in to your account
[MRG] Speed up plot_digits_linkage.py example #21598 #21678
Conversation
There was a problem hiding this comment.
Choose a reason for hiding this comment
The reason will be displayed to describe this comment to others. Learn more.
Otherwise LGTM
There was a problem hiding this comment.
Choose a reason for hiding this comment
The reason will be displayed to describe this comment to others. Learn more.
LGTM, if you merge with the latest main
, your CI would be green. Then we wait for a second reviewer to check the code :)
There was a problem hiding this comment.
Choose a reason for hiding this comment
The reason will be displayed to describe this comment to others. Learn more.
Thanks for the PR @yarkhinephyo!
I left a couple of comments in the code 🤓. Let me know what do you think.
There was a problem hiding this comment.
Choose a reason for hiding this comment
The reason will be displayed to describe this comment to others. Learn more.
I think the main message of the example is quite clearly visible without the nudging data augmentation that also makes the code more complex for little benefit.
However the analysis could be improved to better reflect what we observe (both in main
and in this branch). Let me suggest the following:
What this example shows us is the behavior "rich getting richer" of
agglomerative clustering that tends to create uneven cluster sizes.
This behavior is pronounced for the average linkage strategy,
that ends up with a couple of clusters with few datapoints.
The case of single linkage is even more pathologic with a very
large cluster covering most digits, an intermediate size (clean)
cluster with most zero digits and all other clusters being drawn
from noise points around the fringes.
The other linkage strategies lead to more evenly distributed
clusters that are therefore likely to be less sensible to a
random resampling of the dataset.
```
…t-learn#21678) * Reduce num of samples in plot-digit-linkage example * Remove unnecessary random_state * Remove nudge_images * Address PR comment, elaborate analysis
That's right @siavrez. I've just tested it and it runs 17 times faster. The original implementation runs slower because we used What do you think @ogrisel, @adrinjalali? |
…t-learn#21678) * Reduce num of samples in plot-digit-linkage example * Remove unnecessary random_state * Remove nudge_images * Address PR comment, elaborate analysis
…t-learn#21678) * Reduce num of samples in plot-digit-linkage example * Remove unnecessary random_state * Remove nudge_images * Address PR comment, elaborate analysis
…t-learn#21678) * Reduce num of samples in plot-digit-linkage example * Remove unnecessary random_state * Remove nudge_images * Address PR comment, elaborate analysis
Reference Issues/PRs
#21598
What does this implement/f 10000 ix? Explain your changes.
Speeds up
../examples/cluster/plot_digits_linkage.py
from 32 sec to 20 sec by reducing the number of digits dataset samples from 1800 to 800.Additionally, increased the font size of the numbers and added a random state for
manifold.SpectralEmbedding
.Before:
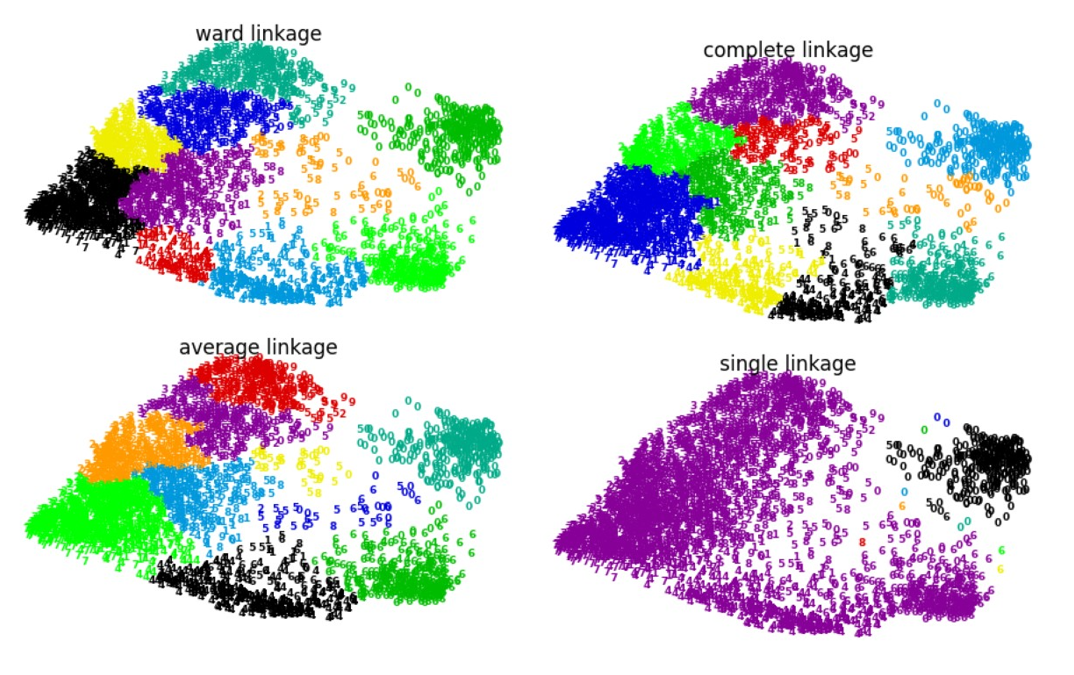
After:

Any other comments?
Nil