Probabilistic modelling of pancreatic cancer survival: can Markov chains predict survival in stage IV pancreatic cancer?
Highlight box
Key findings
• Markov modelling is based on a number of assumptions that may not stand at the more granular sub-stage level of analysis. Despite accurate modelling across pancreatic cancer stages, Markov chains do not appear to provide additional insights at the sub-stage level of stage IV.
What is known and what is new?
• Markov chains have been used to model patient progression and predict survival in cancer patients. Modelling at a more granular level within disease stage IV has not been reported previously and has the potential to enhance patient care. The investigation revealed limitations in extending Markov modelling into the sub-stage level of patient analysis and confirmed superiority of the less complex decision tree analysis for the same.
What is the implication and what should change now?
• Oncology patients present to medical imaging with heightened vulnerability and varying degrees of uncertainty with respect to the path ahead. The ability to provide patients with more refined timelines does not change their outcomes but does afford them the opportunity for better informed decision-making. This approach is consistent with person-centred care but requires exploration of alternative modelling approaches to enhance insights at the more granular level of stage IV.
Introduction
Pancreatic cancer accounts for only 2% of all cancers but is responsible for 5% of cancer deaths (1-3). Symptoms are not obvious early which makes early diagnosis difficult and leaves many patients presenting with advanced metastatic disease (3-5). Surgery is not an option for the 80% of patients presenting with locally advanced or distant metastatic spread (6). Even with treatment, relapse is common for this typically aggressive cancer and 5-year survival is only 2–9% (2-4,6). While radiographic imaging plays an important role in the assessment of the pancreatic cancer patient, novel radiopharmaceuticals targeting the hallmarks of cancer have changed the diagnostic and therapeutic landscape in nuclear medicine. Indeed, neuroendocrine tumours represent the original prototype for theranostics.
Staging employs the TNM (tumour, nodes, metastases) approach and is used to predict prognosis (6). Treatment options also depend on the stage and grade of pancreatic cancer at presentation and include combinations of surgery, chemotherapy, radiation therapy, immunotherapy (including radioimmunotherapy), hormone therapy, peptide receptor radionuclide therapy and palliative care (1,3,7). While survival prediction of patients in early stages of disease is accurate within a wide window depending on therapies, stage IV in particular, with fewer treatment options, is harder to define an accurate life expectancy. For example, stage IV simply means the patient has distant metastases and has 3–7 months median life expectancy (6,8-10). Within that group, metastatic spread to specific organs or tissues may alter life expectancy and it is in these patients that more accurate prediction of life expectancy will be of most benefit.
Staging alone is not the only predictor of survival in pancreatic cancer. Older patients have poorer survival and treatments options also vary survival outcomes. Pancreatic cancer survival is also influenced, to varying degrees, by genetics, gender, ethnicity, marital status, histology, site of primary and sites of metastatic spread (8,10). The relationship between these variables and survival outcomes can be evaluated using conventional inferential statistical approaches and these multivariate approaches are the foundation of current survival predictions (7,11). The increasing digital footprint of patients provides richer data for computational analyses of survival. Various machine learning methods have been applied to understand the weighted and scaled combination of different variables to enhance survival prediction (7,11); quantifying relationships among variables not evident or intuitive to the human observer. For example, Wang et al. (12) used 86 different signatures (variables) and 76 artificial intelligence machine learning algorithms to predict 1-, 2-, and 3-year survival in pancreatic cancer patients. Predictive accuracy, despite complexity, was in the order of 60–75% depending on the subgroup.
Markov chains are another way to mathematically model a sequence of probability vectors or eigenvalues (13-15). If pancreatic cancer survival is considered from the perspective of a dynamic system comprised of variables that change over time, then the probability of a variable (survival) being in any given state (vector) can be predicted for a point in time (13,16). This stochastic process predicts a state based on the previously known state. Markov modelling of random process sequences (Markov chain) takes into account the dependencies among variables (14). In the case of pancreatic cancer, stage II is preceded by stage I, stage III is always preceded by stage II, and stage IV comes after stage III. Within stages, treatment options change from optimal to less optimal with disease progression and with that, a decline in probability of survival. Markov chains, named after Russian mathematician Andrei Markov (16), could provide a simple yet accurate method for predicting pancreatic cancer survival. Limited predictive insights associated with conventional inferential statistical approaches and Kaplan-Meier survival analysis mean that a probabilistic model using Markov chains may provide more accurate information on survival which could then allow better end of life planning for patients and their loved ones, particularly in stage IV; consistent with person centred healthcare.
Methods
The aim of this investigation was to use Markov chains to predict survival rates in pancreatic cancer patients based on stage, particularly stage IV. Matrices and eigenvalues/eigenvectors were used to create transition coefficients that were subsequently feed into the Markov chain and modelling. Outcomes were compared to literature values and decision tree analysis. Ethical considerations were taken into account but this literature-based enquiry was exempt from institutional ethics approval because no human data was collected.
Principal component analysis
When data is high dimensionality, like the variables that may influence cancer survival, there could be hundreds of variables with varying degrees of influence on the outcome of interest. Principal component analysis was used to identify the few variables that account for the greatest variability in survival (17) by assessing the recent literature for published survival predictors. Four recent publications were identified based on reliability of data and were used for analysis (6,9,10,18). In each of these published works, a large patient database was used to evaluate the hazard ratio (HR) of variables to determine which variables most strongly predicted overall survival (Table 1). A number of sociodemographic variables were omitted from principal components because reports varied, lacked statistical power or were made redundant by other included variables (e.g., ethnicity, gender and marital status). Primary site and grade were also excluded because both are made redundant by inclusion of disease stage as a principal component. The principal components for pancreatic cancer survival relate to stage of disease (Table 1) (6,9,10,18). Importantly, representativeness of data was assessed by inclusion of large multi-center analysis of databases [e.g., the Surveillance, Epidemiology and End Results (SEER) registry in the USA] and calibration and rationalization of that data against smaller single centre datasets. Large multi-center registries provide rich data but can suffer the effect of data variability. In this case, the principal component analysis and modelling data contained in Tables 1,2 and consistent and representative across large-multi-center registries and region specific single centre datasets (Table 3).
Table 1
Sources | Component | Class | HR for OS | P value |
---|---|---|---|---|
Shi et al., 2022 and Li et al., 2022 | Age | ≥65 years | 1.49 | <0.01 |
Stage | II | 1.58 | <0.01 | |
III | 2.48 | <0.01 | ||
IV over III | 1.31 | <0.01 | ||
IV | 3.44 | <0.01 | ||
Yao et al., 2022 and Liu et al. 2019 | Metastases | Solitary of liver, lung, lymph nodes or peritoneum | 1.51 | <0.01 |
Multiple including bone | 1.36 | 0.03 | ||
Multiple including brain | 1.21 | 0.64 | ||
Shi et al., 2022, Li et al., 2022 and Yao et al., 2022 | Radiation therapy | Yes | 0.74 | <0.01 |
No | 1.08 | 0.08 | ||
Surgery | Yes | 0.56 | <0.01 | |
No | 2.15 | <0.01 | ||
Chemotherapy | Yes | 0.27 | <0.01 | |
No | 1.82 | <0.01 |
The principal components for further modelling relate to stage, sub-stage and treatment approaches. HR, hazard ratio; OS, overall survival.
Table 2
Items | Stage I | Stage II | Stage III | Stage IV |
---|---|---|---|---|
HR for death | Reference | 1.58 | 2.48 | 3.44 |
Incremental HR for death | – | – | Reference | 1.31 |
Proportion of patients | 0.08 | 0.21 | 0.16 | 0.55 |
No therapy (proportion) | 0.32 | 0.08 | 0.19 | 0.32 |
No chemotherapy proportion (HR) | – | – | 0.35 (1.82) | 0.35 (1.82) |
No surgery proportion (HR) | – | – | 0.75 (2.15) | 0.75 (2.15) |
No radiation therapy proportion (HR) | – | – | 0.87 (1.27) | 0.87 (1.27) |
1-month survival with resection (proportion) | 0.96 | 0.96 | 0.90 | 0.90 |
1-month survival without resection (proportion) | 0.86 | 0.86 | 0.63 | 0.63 |
Solitary metastases proportion (HR) | – | – | – | 0.50 (1.51) |
Multiple metastases proportion (HR) | – | – | – | 0.20 (1.36) |
Brain metastases proportion (HR) | – | – | – | 0.0033 (1.21) |
HR, hazard ratio.
Table 3
Studies | Study type | Source | Subject number | Collection period |
---|---|---|---|---|
Zhang et al., 2024 | Retrospective | SEER national registry in USA | 14,406 | 2010–2015 |
Zhang et al., 2023 | Retrospective | SEER national registry in USA | 2,709 | 2010–2015 |
Liu et al., 2019 | Retrospective | Multi-center [4] registry in Taiwan | 746 | 2010–2016 |
Yao et al., 2022 | Retrospective | SEER national registry in USA | 11,287 | 2010–2016 |
Shi et al., 2022 | Retrospective | SEER national registry in USA | 1,256 | 2000–2018 |
Li et al., 2022 | Retrospective | Single-center study in China | 625 | 2013–2017 |
Yu et al., 2015 | Retrospective | SEER national registry in USA | 13,131 | 2004–2011 |
Lee et al., 2018 | Retrospective | Single-centre study in Korea | 1,646 | 2007–2013 |
Huang et al., 2018 | Retrospective | Multi-national investigation using multiple European and USA registries | 125,183 | 2003–2014 |
Shakeel et al., 2020 | Retrospective | Ontario (Canada) multi-center registry | 6,437 | 2007–2015 |
Zhang et al., 2021 | Retrospective | SEER national registry in USA | 18,832 | 2010–2016 |
SEER, Surveillance, Epidemiology, and End Results.
Markov model
Mathematically, the challenge associated with pancreatic cancer survival modelling is that information about disease progression is generally expressed as a disease state (e.g., stage) at a specific point in time (13). Markov modelling is useful to estimate survival based on disease state. Consider the model outlined in Figure 1 using the data in Table 2. The model assumes stage IV in the sequence must pass through previous stages (even if early stages occur prior to presentation) and that spontaneous regression is not possible (14,24). At time n the state of the system S can be expressed as a state matrix Sn which represents the proportion of patients in a given state starting at time zero (S0) and the state matrix takes the form (13):

where, μ is the single absorbing state (death) rate for each state and λ is the transition rate.
Decision tree analysis
Decision tree analysis is a technique used to provide evidence-based problem solving (25,26). Decision tree analysis allows complex medical scenarios to be mathematically modelled and displayed in a simplified manner while removing subjectivity (25,26). There are other evidence-based approaches to decision making analysis (e.g., Bayes’ theorem and receiver operator characteristic curves) but decision tree analysis is used here due to its versatility to help populate the Markov chain (Figure 1). The data sources for the decision tree analysis are summarised in Tables 1,2.
The initial decision tree analysis (Figure 2) highlights a number of favourable utilities. Good stage I survival is influenced numerically by a very low proportion (0.08) of patients presenting with stage I pancreatic cancer. Good stage II survival is influenced numerically by a high proportion (0.92) of patients suitable for therapy. Poorer survival for stage IV is a product of the nature of late-stage pancreatic cancer, the high proportion of patients who first present at late stage (0.55) and the high proportion of patients not suitable for therapy (0.32). This decision tree analysis does, however, pool sub-stages within stage IV and given the purpose of this modelling was to improve survival prediction among the difficult stage IV cohort, further decision tree analysis was undertaken in isolation for stage IV (Figure 3).


For those pancreatic cancer patients first presenting with stage I or stage II disease, if resection of tumour and therapy are an option, there is a 4-week mortality of just 4% for those under 65 years of age and 6% for those 65 years or older. For those where treatment is not an option (e.g., not available or unsuitable), 4-week mortality is 14% for those under 65 years and 21% for those 65 years or older. This observation is consistent with therapy reducing the tumour doubling rate by 70% (20). Understandably, these values are higher for stage III and stage IV pancreatic cancer. For those where therapy is suitable when patients first present with stage III or stage IV disease, 10% of those under 65 years and 15% of those 65 years and older will die in the 4 weeks after diagnosis. This increases to 37% for under 65 years and 55% for 65 years or older when therapy is not available or unsuitable. This will result in many more actual deaths because there is a predominance of patients who present with advanced disease.
Statistical analysis
Correlation was determined using a match pairs t-test and a P value less than 0.05 was considered significant.
Results
Markov chain calculations
Median survival for stage I, II and III pancreatic cancer are sufficiently long to allow planning, even with some variability in survival predictions. Stage IV pancreatic cancer has a typically short median survival with variability producing significant inaccuracies that, in effect, negatively impact the patient and their families (7,27). Stage I, II and III survival predictions are robust to variations in key variables, within an acceptable margin of error. A more detailed Markov model for stage I can be used to demonstrate the complexity of the process (Figure 4). Stage IV has significant compounding of variables shortening survival. For example, by definition stage IV pancreatic cancer patients have metastases but the extent and the specific organs involved substantially vary survival. While the main goal of this investigation was to delineate stage IV survival using Markov chains (advanced disease), each stage (I, II, III and IV) has been modelled.
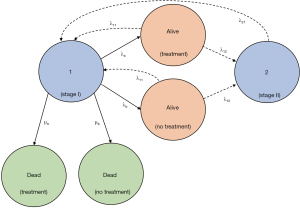
For any given stage, the starting status is alive and thus the probability of a patient starting a stage of pancreatic cancer dying in the subsequent 4-week window can be expressed in the transition matrix (T) form where T = tij (the probability of state i moving to state j) or eigenvalue (13). Each column in the transition matrix represents the current state and each row represents the next state and the values (P) are the eigenvectors (13).
From Table 2;
At time n the state of the system S can be expressed as a state matrix Sn which represents the proportion of patients in a given state starting at time zero (S0), where μ is the single absorbing state (death) rate for each state and λ is the transition rate, with 4-week steps the matrix taking the form (13):
Pooling all stage IV pancreatic cancer patients does not provide the insights expected nor does it demonstrate a full understanding of the disease process. Stage IV alone represents its own Markov chain and can be modelled as such (Figure 5). A simple view using the data tabulated in Table 3 would generate the following matrices:

Stage 4 is differentiated from earlier stages by the presence of distant metastases (mets) and disease progression is characterised by involvement of different organs. The most commonly reported solitary metastases are liver and lung while multiple metastases almost always involved liver or lung plus bone (8,9,18-21). The presence of bone metastases (multiple metastases) can be viewed as meeting the Markov properties of a new sub-stage. Other less common sites of metastases like brain, muscle and nerves can be classified as a subsequent sub-stage. This allows Markov modelling to predict 1 month survival. The state matrix takes the form (13):
For any given stage, the starting status is alive and thus the probability of a patient starting a stage of pancreatic cancer dying in the subsequent month can be expressed in the transition matrix (T) form where T = tij (the probability of state j moving to state i). Each column in the transition matrix represents the current state and each row represents the next state and the values (P) are the eigenvectors. Mathematical resolution of such Markov chains is complex but matrices allow simpler calculations.
Matrix calculations and probability determination
The following Markov chain probability vectors have been calculated on the basis that the Markov property has been satisfied. For the model depicted in Figure 1 for stages I to IV for pancreatic cancer with death being the absorbing state, the matrix calculations are outlined below. The probability vector for the Markov model indicates that the probability of death in any given 4-week window for pancreatic patients is 15%.
Multiply the rows of the transition matrix with the columns of population matrix:
Thus, at the end of 4 weeks, 15% will have died, 7.4% will remain in stage I, 20% will be in stage II, 13.6% will be in stage III and 44% will now be in stage IV. Following the same method, multiple steps in the Markov chain can be determined. After a second step of 4 weeks (8 weeks), 27.3% will have died, 37.5% by week 12, 53% by week 20, 74.3% by 40 weeks, and 80.9% at 12 months.
For the model depicted in Figure 5 for stage IV pancreatic cancer of different sub-stages of metastatic spread, with death being the absorbing state, the probability vectors for each stage for patients undergoing resection are:
Multiply the rows of the transition matrix with the columns of population matrix:
Thus, at the end of 4 weeks, 13.3% will have died. Following the same method, multiple steps (8, 12 and 20 weeks) in the Markov chain can be determined.
After a second step of 4 weeks (8 weeks):
Multiply the rows of the transition matrix with the columns of population matrix:
And then for 12 weeks (3 steps):
Multiply the rows of the transition matrix with the columns of population matrix:
The process could continue through to 5 steps (20 weeks) where 49.9% would have died, 10 steps (40 weeks) for 73.7% dead, and to 12 months at 79.7% dead.
These resection cohort calculations can be compared to those not undergoing resection using the same model depicted in Figure 5. For stage IV for pancreatic cancer of different sub-stages of metastatic spread, with death being the absorbing state, the probability vectors for each stage for patients not undergoing resection are:
Multiply the rows of the transition matrix with the columns of population matrix:
Thus, at the end of 4 weeks, 21.3% will have died. Following the same method, multiple steps (8, 12 and 20 weeks) in the Markov chain can be determined. After a second step of 4 weeks (8 weeks):
Multiply the rows of the transition matrix with the columns of population matrix:
And then for 12 weeks (3 steps):
Multiply the rows of the transition matrix with the columns of population matrix:
The process could continue through to 5 steps (20 weeks) where 59.7% would have died, 10 steps (40 weeks) for 79.1% dead, and to 12 months at 83.8% dead.
Correlation
With the exception of unresected 4-week survival for stage IV pancreatic cancer, there was close agreement between the modelling methods and the literature. Matched pairs t-test revealed that Markov modelling (Table 4) had a 0.798 correlation coefficient compared to decision tree analysis (R2=0.637) and similarly 0.804 with the published literature (R2=0.647). The difference largely reflected unresected stage IV data. There was a close correlation (0.999) between decision tree analysis and the published literature (R2=0.997) which is likely to reflect the derivation of decision tree analysis from the published literature while the Markov approach adopted a different model. Correlation does not equate to agreement between matched pairs and despite the high correlation coefficient and coefficient of determination, the magnitude of values has some differences.
Table 4
Analysis | All (%) | Stage IV (%) | |||||
---|---|---|---|---|---|---|---|
Markov | Decision tree | Literature | Markov | Decision tree | Literature | ||
Overall 4 weeks survival | 85 | 83 | 85.5 | 80 | 76 | 81 | |
Resected 4 weeks survival | – | – | – | 86.9 | 87.0 | 90 | |
Unresected 4 weeks survival | – | – | – | 79.7 | 51.9 | 63 |
The values reflect the probability of surviving for those alive after the next 4 weeks for each Markov chain, decision tree analysis and literature values.
Discussion
Markov modelling has been previously published for survival prediction in breast cancer and thyroid cancer, and for assessing treatment in breast, lung and bladder cancer (13,14,18,24,28,29). Markov chains have not previously been described for survival prediction in pancreatic cancer, however, recent studies investigated the use of Markov modelling to guide decision making for treatment management of pancreatic cancer patients with operable disease (30,31). Duffy et al. (24) used the Markov modelling to successfully predict tumour numbers using node status, size and grade before using the results to predict 10-year survival but, like this investigation, did not reveal any new insights that could not be derived from readily available multi-variate regression analysis. In a recent breast cancer analysis, Markov chains have been used to show an increased probability of both metastases and death with increasing stage (32). One important result was that the Markov model underestimated survival at initial steps but converged on actual survival rates with each additional step. This is consistent with the observations in this investigation.
The novel use of Markov modelling accurately predicts survival at each step (each 4 weeks) but does not allow the more granular insights of decision tree analysis at the survival for each stage or sub-stage. The Markov modelling revealed that, for those with advanced disease (stage IV) at presentation, 4-week mortality is 13.3% for those where treatment is undertaken and 21.3% where treatment is not an option. This is a pooling of all sub-stages that reflect the extent of metastatic spread. Without the capacity to identify the specific probabilities of dying in the subsequent 4-week period for each stage or sub-stage, the insights do not provide patients or their families with any more accurate predictions for survival. The Markov modelling confirms predictions already known but does not inform additional decision making. Patients survive, even with advanced disease, defying probability. Consequently, while a diagnosis of late-stage pancreatic cancer with extensive metastatic spread should drive urgency in getting ones “affairs in order”, it does not mean hope should be abandoned for a longer survival window.
For the decision tree analysis, the probability of dying in the 4-weeks after diagnosis is well supported by literature, however, the specific number or proportion of patients first presenting in each stage might vary from site to site. Consequently, the line probabilities for 4-week mortality (utilities) can be considered externally valid while the death rates per 100,000 of presenting population have internal validity without external transferability. Unlike the Markov modelling, the stage IV decision tree analysis allows prediction of 4-week mortality based on the sub-stage or extent of metastases (Figure 3). A positive outcome for those presenting with stage IV pancreatic cancer would be restriction of metastases to a single site with suitability for therapy; a 15% 4-week mortality. In the same circumstance where therapy was not available or suitable, the 4-week mortality increases to 55%. Patients with multiple (including bone) metastases are less likely to be suitable for therapy but if they are they face a 20% 4-week mortality compared to those not suitable for therapy facing a 76% 4-week mortality. In dire circumstances where they are presenting for the first time with advanced disease and metastatic spread to multiple other organs (e.g., brain, muscle, nerves), they are almost certainly not suited to therapy (other than symptom relief or palliation) and have a 92% 4-week mortality; virtually 100% for those over 75 years. There are some limitations associated with the probabilities (Figure 3) because the 50% not having therapy but yet to develop solitary metastases is an over-estimation since many will be re-staged and subsequently have therapy. Conversely a large proportion of those with multiple metastases and almost all with brain metastases will not have therapy if that is the state they present. This is reflected in Figure 6 inclusion of case shunting (dashed lines). Shunting refers to the redirection of flow in the decision tree due to later adjustments to the decision tree. Specifically, for patients without liver metastases but also without surgery being redirected to the therapy line (25%) and those patients undergoing therapy becoming ineligible due to multiple metastases being redirected to the no therapy arms of the decision tree. This model does not change the line probabilities but does change the number per 100,000 who die along each line which would be important if the decision tree model were used for cost:benefit or cost:effectiveness analysis.

Limitations
A key consideration is whether the model adhered to the Markovian assumptions. The Markov chain is valid for progression among pancreatic cancer stages (I, II, III to IV) but maybe not for the stage IV sub-stage analysis. This analysis may not fit the Markov chain assumption of future dependence on the previous state. For example, to fit the Markov chain model, the sub-stage of having multiple metastases depends on a previous stage of solitary metastases that is not bone. While uncommon, it is possible in the real world for solitary bone metastases to develop. The uncommon nature of this means that any errors are likely to be small. It should also be noted that the decision tree analysis was also modelled on Markovian assumptions and so non-Markov processes were omitted. Beyond Markovian assumptions, the modelling framework is conceptual in nature and limited by model constraints and the data used.
The other important Markovian assumption is time homogeneity. While the very nature of modelling is to simplify complex processes, some consideration needs to be given to the complexity of pancreatic cancer progression. The model adopted assumes a constant transition rate. There is debate in literature whether tumour growth is exponential (stage I and II in particular) with a Gompertzian tail (stage IV) or linear mostly throughout (20,33-35). The manner in which a tumour grows and spreads will change outcomes. The transition probabilities used in this investigation reflect the previously published doubling rate for pancreatic cancer of 40–60 days (20). A stage transition rate of 40–60 days is also consistent with the exponential model during stages I and II based on the size criteria for tumours with a Gompertzian drop off normalising the stage transition rate to be approximately linear. On this basis, the model meets the time homogeneity assumption and this is supported by application of the model being consistent with the 6–12 months life expectancy (20,27).
The data used (Tables 1,2) for modelling are based on previous studies that may lack generalisability. As result, as a model there is a degree of insight from internal validity but external validity remains contentious. Extrapolation of data needs to consider local data which are readily transferable into the models outlined. Nonetheless, as a model, the data adopted provided consistency to allow a robust analysis which informs generalisable conclusions.
Recommendations
The most important recommendation from this investigation is to employ the survival insights from the decision tree analysis to provide patients and their families with more realistic predictions. Predictions should not be seen as pessimistic or optimistic, but rather realistic. A 76% chance of dying in the next 4 weeks for a patient with untreatable stage IV pancreatic cancer with liver and bone metastases allows patients and families to make decisions and arrangements while holding hope that they might be among the 24% that survive 4 weeks. Adopting broad survival predictions inclusive of all stages or sub-stages over-estimates survival time in those presenting with advanced or untreatable pancreatic cancer.
Conclusions
While Markov modelling allowed survival prediction among pancreatic cancer patients, the insights were limited to an all-stage cohort. Despite this, transition probabilities could be used to assess risk at a stage or sub-stage level but these values are more accurately applied for survival prediction using decision tree analysis. The decision tree analysis provided modelling of survival at a more granular level and as a result, would be more suitable than Markov modelling or current models based on regression analysis for predicting survival for patients and their families.
Acknowledgments
Funding: None.
Footnote
Data Sharing Statement: Available at https://apc.amegroups.com/article/view/10.21037/apc-24-8/dss
Peer Review File: Available at https://apc.amegroups.com/article/view/10.21037/apc-24-8/prf
Conflicts of Interest: All authors have completed the ICMJE uniform disclosure form (available at https://apc.amegroups.com/article/view/10.21037/apc-24-8/coif). The authors have no conflicts of interest to declare.
Ethical Statement: The authors are accountable for all aspects of the work in ensuring that questions related to the accuracy or integrity of any part of the work are appropriately investigated and resolved. Ethical considerations were taken into account but this literature-based enquiry was exempt from institutional ethics approval because no human data was collected.
Open Access Statement: This is an Open Access article distributed in accordance with the Creative Commons Attribution-NonCommercial-NoDerivs 4.0 International License (CC BY-NC-ND 4.0), which permits the non-commercial replication and distribution of the article with the strict proviso that no changes or edits are made and the original work is properly cited (including links to both the formal publication through the relevant DOI and the license). See: https://creativecommons.org/licenses/by-nc-nd/4.0/.
References
- Loveday BPT, Lipton L, Thomson BN. Pancreatic cancer: An update on diagnosis and management. Aust J Gen Pract 2019;48:826-31. [Crossref] [PubMed]
- Shin DW, Kim J. The American Joint Committee on Cancer 8th edition staging system for the pancreatic ductal adenocarcinoma: is it better than the 7th edition? Hepatobiliary Surg Nutr 2020;9:98-100.
- Zhao Z, Liu W. Pancreatic Cancer: A Review of Risk Factors, Diagnosis, and Treatment. Technol Cancer Res Treat 2020;19:1533033820962117. [Crossref] [PubMed]
- Lee DH, Jang JY, Kang JS, et al. Recent treatment patterns and survival outcomes in pancreatic cancer according to clinical stage based on single-center large-cohort data. Ann Hepatobiliary Pancreat Surg 2018;22:386-96. [Crossref] [PubMed]
- Torphy RJ, Fujiwara Y, Schulick RD. Pancreatic cancer treatment: better, but a long way to go. Surg Today 2020;50:1117-25. [Crossref] [PubMed]
- Shi H, Chen Z, Dong S, et al. A nomogram for predicting survival in patients with advanced (stage III/IV) pancreatic body tail cancer: a SEER-based study. BMC Gastroenterol 2022;22:279. [Crossref] [PubMed]
- Bakasa W, Viriri S. Pancreatic Cancer Survival Prediction: A Survey of the State-of-the-Art. Comput Math Methods Med 2021;2021:1188414. [Crossref] [PubMed]
- Shakeel S, Finley C, Akhtar-Danesh G, et al. Trends in survival based on treatment modality in patients with pancreatic cancer: a population-based study. Curr Oncol 2020;27:e1-8. [Crossref] [PubMed]
- Li Q, Feng Z, Miao R, et al. Prognosis and survival analysis of patients with pancreatic cancer: retrospective experience of a single institution. World J Surg Oncol 2022;20:11. [Crossref] [PubMed]
- Yao ZX, Tu JH, Zhou B, et al. Risk factors and survival prediction of pancreatic cancer with lung metastases: A population-based study. Front Oncol 2022;12:952531. [Crossref] [PubMed]
- Kaissis G, Ziegelmayer S, Lohöfer F, et al. A machine learning model for the prediction of survival and tumor subtype in pancreatic ductal adenocarcinoma from preoperative diffusion-weighted imaging. Eur Radiol Exp 2019;3:41. [Crossref] [PubMed]
- Wang L, Liu Z, Liang R, et al. Comprehensive machine-learning survival framework develops a consensus model in large-scale multicenter cohorts for pancreatic cancer. Elife 2022;11:e80150. [Crossref] [PubMed]
- Kay R. A Markov model for analysing cancer markers and disease states in survival studies. Biometrics 1986;42:855-65. [Crossref] [PubMed]
- Cong C, Tsokos CP. Markov modelling of breast cancer. Journal of Modern Applied Statistical Methods 2009;8:626-31. [Crossref]
- Lay DC, Lay SR, McDonald JJ. Linear algebra and its applications, 6th edn. Pearson, Harlow UK; 2022.
- Anton H, Rorres C, Kaul A. Elementary linear algebra, 12th edn. Wiley, New Jersey; 2019.
- Chan YH. Principal component and factor analysis. In: Marcoulides GA, Hershberger SL. editors. Multivariate Statistical Methods. 1st edn. New York: Psychology Press; 2004.
- Liu KH, Hung CY, Hsueh SW, et al. Lung Metastases in Patients with Stage IV Pancreatic Cancer: Prevalence, Risk Factors, and Survival Impact. J Clin Med 2019;8:1402. [Crossref] [PubMed]
- Zhang W, Ji L, Wang X, et al. Nomogram Predicts Risk and Prognostic Factors for Bone Metastasis of Pancreatic Cancer: A Population-Based Analysis. Front Endocrinol (Lausanne) 2021;12:752176. [Crossref] [PubMed]
- Yu J, Blackford AL, Dal Molin M, et al. Time to progression of pancreatic ductal adenocarcinoma from low-to-high tumour stages. Gut 2015;64:1783-9. [Crossref] [PubMed]
- Zhang L, Jin R, Yang X, et al. A population-based study of synchronous distant metastases and prognosis in patients with PDAC at initial diagnosis. Front Oncol 2023;13:1087700. [Crossref] [PubMed]
- Huang L, Jansen L, Balavarca Y, et al. Stratified survival of resected and overall pancreatic cancer patients in Europe and the USA in the early twenty-first century: a large, international population-based study. BMC Med 2018;16:125. [Crossref] [PubMed]
- Zhang H, Tan Q, Xiang C, et al. Increased risk of multiple metastases and worse overall survival of metastatic pancreatic body and tail cancer: a retrospective cohort study. Gland Surg 2024;13:480-9. [Crossref] [PubMed]
- Duffy SW, Day NE, Tabár L, et al. Markov models of breast tumor progression: some age-specific results. J Natl Cancer Inst Monogr 1997;93-7. [Crossref] [PubMed]
- Bae JM. The clinical decision analysis using decision tree. Epidemiol Health 2014;36:e2014025. [Crossref] [PubMed]
- Karacan I, Sennaroglu B, Vayvay O. Analysis of life expectancy across countries using a decision tree. East Mediterr Health J 2020;26:143-51. [Crossref] [PubMed]
- Chakraborty A, Tsokos CP. A modern approach of survival analysis of patients with pancreatic cancer. Am J Cancer Res 2021;11:4725-45. [PubMed]
- Newton PK, Mason J, Bethel K, et al. A stochastic Markov chain model to describe lung cancer growth and metastasis. PLoS One 2012;7:e34637. [Crossref] [PubMed]
- Montoro-Cazorla D, Pérez-Ocón R. Pereira das Neves-Yedig A. A longitudinal study of the bladder cancer applying a state-space model with non-exponential staying time in states. Mathematics 2021;9:363. [Crossref]
- Bradley A, Van Der Meer R. Neoadjuvant therapy versus upfront surgery for potentially resectable pancreatic cancer: A Markov decision analysis. PLoS One 2019;14:e0212805. [Crossref] [PubMed]
- Rieser CJ, Narayanan S, Bahary N, et al. Optimal management of patients with operable pancreatic head cancer: A Markov decision analysis. J Surg Oncol 2021;124:801-9. [Crossref] [PubMed]
- Lin RH, Lin CS, Chuang CL, et al. Breast Cancer Survival Analysis Model. Appl Sci 2022;12:1971. [Crossref]
- Norton L. Cancer stem cells, self-seeding, and decremented exponential growth: theoretical and clinical implications. Breast Dis 2008;29:27-36. [Crossref] [PubMed]
- Benzekry S, Lamont C, Beheshti A, et al. Classical mathematical models for description and prediction of experimental tumor growth. PLoS Comput Biol 2014;10:e1003800. [Crossref] [PubMed]
- Retsky MW, Swartzendruber DE, Wardwell RH, et al. Is Gompertzian or exponential kinetics a valid description of individual human cancer growth? Med Hypotheses 1990;33:95-106. [Crossref] [PubMed]
Cite this article as: Currie J, Currie G, Rohren E. Probabilistic modelling of pancreatic cancer survival: can Markov chains predict survival in stage IV pancreatic cancer? Ann Pancreat Cancer 2024;7:7.