|
ACCESS THE FULL ARTICLE
No SPIE Account? Create one
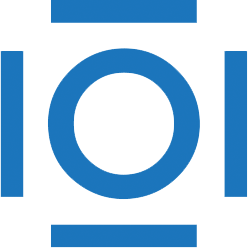
CITATIONS
Cited by 14 scholarly publications.
Detection and tracking algorithms
Remote sensing
Binary data
Statistical analysis
Chemical elements
Expectation maximization algorithms
Image resolution