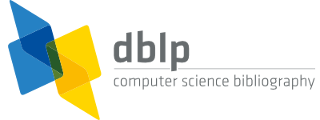


default search action
Henrik Boström
Person information
- affiliation: Stockholm University
Refine list

refinements active!
zoomed in on ?? of ?? records
view refined list in
export refined list as
2020 – today
- 2024
- [j28]Amir Hossein Akhavan Rahnama
, Judith Bütepage, Pierre Geurts, Henrik Boström:
Can local explanation techniques explain linear additive models? Data Min. Knowl. Discov. 38(1): 237-280 (2024) - [c120]Sofiane Ennadir, Yassine Abbahaddou, Johannes F. Lutzeyer, Michalis Vazirgiannis, Henrik Boström:
A Simple and Yet Fairly Effective Defense for Graph Neural Networks. AAAI 2024: 21063-21071 - [c119]Amr Alkhatib, Sofiane Ennadir, Henrik Boström, Michalis Vazirgiannis:
Interpretable Graph Neural Networks for Tabular Data. ECAI 2024: 1848-1855 - [c118]Yassine Abbahaddou, Sofiane Ennadir, Johannes F. Lutzeyer, Michalis Vazirgiannis, Henrik Boström:
Bounding the Expected Robustness of Graph Neural Networks Subject to Node Feature Attacks. ICLR 2024 - [c117]Henrik Boström
:
Example-Based Explanations of Random Forest Predictions. IDA (2) 2024: 185-196 - [c116]Amr Alkhatib, Henrik Boström:
Fast Approximation of Shapley Values with Limited Data. SCAI 2024: 95-100 - [c115]Amir Hossein Akhavan Rahnama, Judith Bütepage, Henrik Boström:
Local Point-wise Explanations of LambdaMART. SCAI 2024: 121-130 - [c114]Amir Hossein Akhavan Rahnama
, Judith Bütepage
, Henrik Boström
:
Local List-Wise Explanations of LambdaMART. xAI (2) 2024: 369-392 - [i13]Sofiane Ennadir, Yassine Abbahaddou, Johannes F. Lutzeyer, Michalis Vazirgiannis, Henrik Boström:
A Simple and Yet Fairly Effective Defense for Graph Neural Networks. CoRR abs/2402.13987 (2024) - [i12]Yassine Abbahaddou, Sofiane Ennadir, Johannes F. Lutzeyer, Michalis Vazirgiannis, Henrik Boström:
Bounding the Expected Robustness of Graph Neural Networks Subject to Node Feature Attacks. CoRR abs/2404.17947 (2024) - [i11]Amr Alkhatib, Henrik Boström, Michalis Vazirgiannis:
Explaining Predictions by Characteristic Rules. CoRR abs/2405.21003 (2024) - [i10]Amr Alkhatib, Henrik Boström:
Interpretable Graph Neural Networks for Heterogeneous Tabular Data. CoRR abs/2408.07661 (2024) - 2023
- [c113]Sofiane Ennadir, Amr Alkhatib, Giannis Nikolentzos, Michalis Vazirgiannis, Henrik Boström:
UnboundAttack: Generating Unbounded Adversarial Attacks to Graph Neural Networks. COMPLEX NETWORKS (1) 2023: 100-111 - [c112]Sofiane Ennadir, Amr Alkhatib, Henrik Boström, Michalis Vazirgiannis:
Conformalized Adversarial Attack Detection for Graph Neural Networks. COPA 2023: 311-323 - [c111]Henrik Boström, Henrik Linusson, Anders Vesterberg:
Mondrian Predictive Systems for Censored Data. COPA 2023: 399-412 - [c110]Amr Alkhatib, Henrik Boström, Sofiane Ennadir, Ulf Johansson:
Approximating Score-based Explanation Techniques Using Conformal Regression. COPA 2023: 450-469 - [c109]Niharika Gauraha, Henrik Boström:
Investigating the Contribution of Privileged Information in Knowledge Transfer LUPI by Explainable Machine Learning. COPA 2023: 470-484 - [c108]Ulf Johansson, Cecilia Sönströd, Tuwe Löfström, Henrik Boström:
Confidence Classifiers with Guaranteed Accuracy or Precision. COPA 2023: 513-533 - [c107]Tuwe Löfström, Alexander Bondaletov, Artem Ryasik, Henrik Boström, Ulf Johansson:
Tutorial on using Conformal Predictive Systems in KNIME. COPA 2023: 602-620 - [e3]Harris Papadopoulos, Khuong An Nguyen, Henrik Boström, Lars Carlsson:
Conformal and Probabilistic Prediction with Applications, 13-15 September 2023, Limassol, Cyprus. Proceedings of Machine Learning Research 204, PMLR 2023 [contents] - [i9]Amr Alkhatib, Sofiane Ennadir, Henrik Boström, Michalis Vazirgiannis:
Interpretable Graph Neural Networks for Tabular Data. CoRR abs/2308.08945 (2023) - [i8]Amr Alkhatib, Henrik Boström, Sofiane Ennadir, Ulf Johansson:
Approximating Score-based Explanation Techniques Using Conformal Regression. CoRR abs/2308.11975 (2023) - [i7]Henrik Boström:
Example-Based Explanations of Random Forest Predictions. CoRR abs/2311.14581 (2023) - 2022
- [j27]Sampath Deegalla
, Keerthi Walgama, Panagiotis Papapetrou, Henrik Boström
:
Random subspace and random projection nearest neighbor ensembles for high dimensional data. Expert Syst. Appl. 191: 116078 (2022) - [j26]Ulf Johansson, Cecilia Sönströd, Tuwe Löfström
, Henrik Boström:
Rule extraction with guarantees from regression models. Pattern Recognit. 126: 108554 (2022) - [c106]Ulf Johansson, Henrik Boström, Khuong An Nguyen, Zhiyuan Luo, Lars Carlsson:
Preface. COPA 2022: 1-3 - [c105]Henrik Boström:
crepes: a Python Package for Generating Conformal Regressors and Predictive Systems. COPA 2022: 24-41 - [c104]Amr Alkhatib, Henrik Boström, Ulf Johansson:
Assessing Explanation Quality by Venn Prediction. COPA 2022: 42-54 - [c103]Amr Alkhatib
, Henrik Boström
, Michalis Vazirgiannis
:
Explaining Predictions by Characteristic Rules. ECML/PKDD (1) 2022: 389-403 - [e2]Ulf Johansson, Henrik Boström, Khuong An Nguyen, Zhiyuan Luo, Lars Carlsson:
Conformal and Probabilistic Prediction with Applications, 24-26 August 2022, Brighton, UK. Proceedings of Machine Learning Research 179, PMLR 2022 [contents] - [i6]Nancy Xu, Giannis Nikolentzos, Michalis Vazirgiannis, Henrik Boström:
Image Keypoint Matching using Graph Neural Networks. CoRR abs/2205.14275 (2022) - 2021
- [c102]Nancy Xu, Giannis Nikolentzos, Michalis Vazirgiannis, Henrik Boström:
Image Keypoint Matching Using Graph Neural Networks. COMPLEX NETWORKS 2021: 441-451 - [c101]Henrik Boström, Ulf Johansson, Tuwe Löfström:
Mondrian conformal predictive distributions. COPA 2021: 24-38 - [c100]Ulf Johansson, Tuwe Löfström, Henrik Boström:
Calibrating multi-class models. COPA 2021: 111-130 - [c99]Hugo Werner, Lars Carlsson, Ernst Ahlberg, Henrik Boström:
Evaluation of updating strategies for conformal predictive systems in the presence of extreme events. COPA 2021: 229-242 - [c98]Ulf Johansson
, Tuwe Löfström
, Henrik Boström
:
Well-Calibrated and Sharp Interpretable Multi-Class Models. MDAI 2021: 193-204 - [c97]Ulf Johansson, Henrik Boström, Tuwe Löfström
:
Investigating Normalized Conformal Regressors. SSCI 2021: 1-8 - [i5]Negar Safinianaini, Henrik Boström:
Towards interpretability of Mixtures of Hidden Markov Models. CoRR abs/2103.12576 (2021) - [i4]Amir Hossein Akhavan Rahnama, Judith Bütepage, Pierre Geurts, Henrik Boström:
Evaluation of Local Model-Agnostic Explanations Using Ground Truth. CoRR abs/2106.02488 (2021) - 2020
- [j25]Ricardo Aler
, José María Valls
, Henrik Boström:
Study of Hellinger Distance as a splitting metric for Random Forests in balanced and imbalanced classification datasets. Expert Syst. Appl. 149: 113264 (2020) - [j24]Henrik Linusson, Ulf Johansson, Henrik Boström:
Efficient conformal predictor ensembles. Neurocomputing 397: 266-278 (2020) - [j23]Jing Zhao, Panagiotis Papapetrou, Lars Asker, Henrik Boström:
Corrigendum to 'Learning from heterogeneous temporal data in electronic health records'. [J. Biomed. Inform. 65 (2017) 105-119]. J. Biomed. Informatics 101: 103352 (2020) - [c96]Henrik Boström, Ulf Johansson:
Mondrian conformal regressors. COPA 2020: 114-133 - [c95]Hugo Werner, Lars Carlsson, Ernst Ahlberg, Henrik Boström:
Evaluating different approaches to calibrating conformal predictive systems. COPA 2020: 134-150 - [c94]Linn Karlsson, Henrik Boström, Paul Zieger:
Classication of aerosol particles using inductive conformal prediction. COPA 2020: 257-268 - [c93]Henrik Boström, Peter Höglund, Sven-Olof Junker, Ann-Sofie Öberg, Martin Sparr:
Explaining Multivariate Time Series Forecasts: An Application to Predicting the Swedish GDP. XI-ML@KI 2020 - [c92]Negar Safinianaini, Camila P. E. de Souza
, Henrik Boström, Jens Lagergren:
Orthogonal Mixture of Hidden Markov Models. ECML/PKDD (1) 2020: 509-525
2010 – 2019
- 2019
- [j22]Theodore Vasiloudis, Gianmarco De Francisci Morales, Henrik Boström:
Quantifying Uncertainty in Online Regression Forests. J. Mach. Learn. Res. 20: 155:1-155:35 (2019) - [j21]Alexander Gammerman
, Vladimir Vovk, Henrik Boström, Lars Carlsson:
Conformal and probabilistic prediction with applications: editorial. Mach. Learn. 108(3): 379-380 (2019) - [j20]Ulf Johansson
, Tuve Löfström
, Henrik Linusson, Henrik Boström:
Efficient Venn predictors using random forests. Mach. Learn. 108(3): 535-550 (2019) - [c91]Negar Safinianaini, Henrik Boström, Viktor Kaldo:
Gated Hidden Markov Models for Early Prediction of Outcome of Internet-Based Cognitive Behavioral Therapy. AIME 2019: 160-169 - [c90]Ulf Johansson, Tuwe Löfström, Henrik Boström, Cecilia Sönströd:
Interpretable and specialized conformal predictors. COPA 2019: 3-22 - [c89]Henrik Boström, Ulf Johansson, Anders Vesterberg:
Predicting with Confidence from Survival Data. COPA 2019: 123-141 - [c88]Ulf Johansson, Cecilia Sönströd, Tuwe Löfström
, Henrik Boström:
Customized Interpretable Conformal Regressors. DSAA 2019: 221-230 - [c87]Ulf Johansson, Tuwe Löfström
, Henrik Boström:
Calibrating Probability Estimation Trees using Venn-Abers Predictors. SDM 2019: 28-36 - [c86]Theodore Vasiloudis, Hyunsu Cho, Henrik Boström:
Block-distributed Gradient Boosted Trees. SIGIR 2019: 1025-1028 - [i3]Theodore Vasiloudis, Hyunsu Cho, Henrik Boström:
Block-distributed Gradient Boosted Trees. CoRR abs/1904.10522 (2019) - [i2]Amir Hossein Akhavan Rahnama, Henrik Boström:
A study of data and label shift in the LIME framework. CoRR abs/1910.14421 (2019) - 2018
- [j19]Ulf Johansson, Henrik Linusson, Tuve Löfström
, Henrik Boström:
Interpretable regression trees using conformal prediction. Expert Syst. Appl. 97: 394-404 (2018) - [c85]Ulf Johansson, Tuwe Löfström, Håkan Sundell, Henrik Linusson, Anders Gidenstam, Henrik Boström:
Venn predictors for well-calibrated probability estimation trees. COPA 2018: 3-14 - [c84]Henrik Linusson, Ulf Johansson, Henrik Boström, Tuve Löfström
:
Classification with Reject Option Using Conformal Prediction. PAKDD (1) 2018: 94-105 - [c83]Jaakko Hollmén, Lars Asker, Isak Karlsson, Panagiotis Papapetrou, Henrik Boström, Birgitta Norstedt Wikner, Inger Öhman
:
Exploring epistaxis as an adverse effect of anti-thrombotic drugs and outdoor temperature. PETRA 2018: 1-4 - 2017
- [j18]Henrik Boström
, Henrik Linusson, Tuve Löfström
, Ulf Johansson:
Accelerating difficulty estimation for conformal regression forests. Ann. Math. Artif. Intell. 81(1-2): 125-144 (2017) - [j17]Jing Zhao, Panagiotis Papapetrou, Lars Asker, Henrik Boström:
Learning from heterogeneous temporal data in electronic health records. J. Biomed. Informatics 65: 105-119 (2017) - [c82]Henrik Linusson, Ulf Norinder, Henrik Boström, Ulf Johansson, Tuve Löfström:
On the Calibration of Aggregated Conformal Predictors. COPA 2017: 154-173 - [c81]Ernst Ahlberg, Susanne Winiwarter, Henrik Boström, Henrik Linusson, Tuve Löfström, Ulf Norinder, Ulf Johansson, Ola Engkvist, Oscar Hammar, Claus Bendtsen, Lars Carlsson:
Using Conformal Prediction to Prioritize Compound Synthesis in Drug Discovery. COPA 2017: 174-184 - [c80]Henrik Boström, Lars Asker, Ram B. Gurung, Isak Karlsson, Tony Lindgren, Panagiotis Papapetrou:
Conformal Prediction Using Random Survival Forests. ICMLA 2017: 812-817 - [c79]Ulf Johansson, Henrik Linusson, Tuve Löfström
, Henrik Boström:
Model-agnostic nonconformity functions for conformal classification. IJCNN 2017: 2072-2079 - [c78]Isak Karlsson, Panagiotis Papapetrou, Lars Asker, Henrik Boström, Hans E. Persson:
Mining disproportional itemsets for characterizing groups of heart failure patients from administrative health records. PETRA 2017: 394-398 - 2016
- [j16]Isak Karlsson
, Panagiotis Papapetrou, Henrik Boström:
Generalized random shapelet forests. Data Min. Knowl. Discov. 30(5): 1053-1085 (2016) - [j15]Aron Henriksson, Jing Zhao, Hercules Dalianis, Henrik Boström:
Ensembles of randomized trees using diverse distributed representations of clinical events. BMC Medical Informatics Decis. Mak. 16(S-2): 69 (2016) - [c77]Lars Asker, Henrik Boström, Panagiotis Papapetrou, Hans E. Persson:
Identifying Factors for the Effectiveness of Treatment of Heart Failure: A Registry Study. CBMS 2016: 205-206 - [c76]Henrik Boström, Henrik Linusson, Tuve Löfström
, Ulf Johansson:
Evaluation of a Variance-Based Nonconformity Measure for Regression Forests. COPA 2016: 75-89 - [c75]Isak Karlsson, Panagiotis Papapetrou, Henrik Boström:
Early Random Shapelet Forest. DS 2016: 261-276 - [c74]Ram B. Gurung, Tony Lindgren, Henrik Boström:
Learning Decision Trees from Histogram Data Using Multiple Subsets of Bins. FLAIRS 2016: 430-435 - [c73]Isak Karlsson, Henrik Boström:
Predicting Adverse Drug Events Using Heterogeneous Event Sequences. ICHI 2016: 356-362 - [c72]Henrik Linusson, Ulf Johansson, Henrik Boström, Tuve Löfström
:
Reliable Confidence Predictions Using Conformal Prediction. PAKDD (1) 2016: 77-88 - [c71]Lars Asker, Panagiotis Papapetrou, Henrik Boström:
Learning from Swedish Healthcare Data. PETRA 2016: 47 - [e1]Henrik Boström, Arno J. Knobbe, Carlos Soares
, Panagiotis Papapetrou:
Advances in Intelligent Data Analysis XV - 15th International Symposium, IDA 2016, Stockholm, Sweden, October 13-15, 2016, Proceedings. Lecture Notes in Computer Science 9897, 2016, ISBN 978-3-319-46348-3 [contents] - [i1]Andreas Henelius, Kai Puolamäki, Henrik Boström, Panagiotis Papapetrou:
Clustering with Confidence: Finding Clusters with Statistical Guarantees. CoRR abs/1612.08714 (2016) - 2015
- [j14]Catarina Dudas, Amos H. C. Ng, Henrik Boström:
Post-analysis of multi-objective optimization solutions using decision trees. Intell. Data Anal. 19(2): 259-278 (2015) - [j13]Tuve Löfström
, Henrik Boström, Henrik Linusson, Ulf Johansson:
Bias reduction through conditional conformal prediction. Intell. Data Anal. 19(6): 1355-1375 (2015) - [j12]Jing Zhao, Aron Henriksson, Lars Asker, Henrik Boström:
Predictive modeling of structured electronic health records for adverse drug event detection. BMC Medical Informatics Decis. Mak. 15-S(4): S1 (2015) - [c70]Jing Zhao, Aron Henriksson, Maria Kvist, Lars Asker, Henrik Boström:
Handling Temporality of Clinical Events for Drug Safety Surveillance. AMIA 2015 - [c69]Aron Henriksson, Jing Zhao, Henrik Boström, Hercules Dalianis:
Modeling electronic health records in ensembles of semantic spaces for adverse drug event detection. BIBM 2015: 343-350 - [c68]Aron Henriksson, Jing Zhao, Henrik Boström, Hercules Dalianis:
Modeling heterogeneous clinical sequence data in semantic space for adverse drug event detection. DSAA 2015: 1-8 - [c67]Jing Zhao, Aron Henriksson, Henrik Boström:
Cascading adverse drug event detection in electronic health records. DSAA 2015: 1-8 - [c66]Andreas Henelius, Kai Puolamäki
, Isak Karlsson, Jing Zhao, Lars Asker, Henrik Boström, Panagiotis Papapetrou:
GoldenEye++: A Closer Look into the Black Box. SLDS 2015: 96-105 - [c65]Isak Karlsson, Panagiotis Papapetrou, Henrik Boström:
Forests of Randomized Shapelet Trees. SLDS 2015: 126-136 - [c64]Lars Carlsson, Ernst Ahlberg, Henrik Boström, Ulf Johansson, Henrik Linusson:
Modifications to p-Values of Conformal Predictors. SLDS 2015: 251-259 - [c63]Ulf Johansson, Ernst Ahlberg, Henrik Boström, Lars Carlsson, Henrik Linusson, Cecilia Sönströd:
Handling Small Calibration Sets in Mondrian Inductive Conformal Regressors. SLDS 2015: 271-280 - 2014
- [j11]Andreas Henelius, Kai Puolamäki
, Henrik Boström, Lars Asker, Panagiotis Papapetrou
:
A peek into the black box: exploring classifiers by randomization. Data Min. Knowl. Discov. 28(5-6): 1503-1529 (2014) - [j10]Catarina Dudas, Amos H. C. Ng, Leif Pehrsson, Henrik Boström:
Integration of data mining and multi-objective optimisation for decision support in production systems development. Int. J. Comput. Integr. Manuf. 27(9): 824-839 (2014) - [j9]Ulf Johansson, Henrik Boström, Tuve Löfström
, Henrik Linusson:
Regression conformal prediction with random forests. Mach. Learn. 97(1-2): 155-176 (2014) - [c62]Jing Zhao, Aron Henriksson, Lars Asker, Henrik Boström:
Detecting adverse drug events with multiple representations of clinical measurements. BIBM 2014: 536-543 - [c61]Ulf Johansson, Cecilia Sönströd, Henrik Linusson, Henrik Boström:
Regression trees for streaming data with local performance guarantees. IEEE BigData 2014: 461-470 - [c60]Isak Karlsson, Henrik Boström:
Handling Sparsity with Random Forests When Predicting Adverse Drug Events from Electronic Health Records. ICHI 2014: 17-22 - [c59]Jing Zhao, Aron Henriksson, Henrik Boström:
Detecting Adverse Drug Events Using Concept Hierarchies of Clinical Codes. ICHI 2014: 285-293 - [c58]Henrik Linusson, Ulf Johansson, Henrik Boström, Tuve Löfström
:
Efficiency Comparison of Unstable Transductive and Inductive Conformal Classifiers. AIAI Workshops 2014: 261-270 - [c57]Ulf Johansson, Rikard König, Henrik Linusson, Tuve Löfström
, Henrik Boström:
Rule Extraction with Guaranteed Fidelity. AIAI Workshops 2014: 281-290 - [c56]Karl Jansson, Håkan Sundell, Henrik Boström:
gpuRF and gpuERT: Efficient and Scalable GPU Algorithms for Decision Tree Ensembles. IPDPS Workshops 2014: 1612-1621 - [c55]Lars Asker, Henrik Boström, Isak Karlsson, Panagiotis Papapetrou, Jing Zhao:
Mining candidates for adverse drug interactions in electronic patient records. PETRA 2014: 22:1-22:4 - 2013
- [j8]Thashmee Karunaratne, Henrik Boström, Ulf Norinder
:
Comparative analysis of the use of chemoinformatics-based and substructure-based descriptors for quantitative structure-activity relationship (QSAR) modeling. Intell. Data Anal. 17(2): 327-341 (2013) - [c54]Orlando P. Zacarias, Henrik Boström:
Generalization of Malaria Incidence Prediction Models by Correcting Sample Selection Bias. ADMA (2) 2013: 189-200 - [c53]Isak Karlsson, Jing Zhao, Lars Asker, Henrik Boström:
Predicting Adverse Drug Events by Analyzing Electronic Patient Records. AIME 2013: 125-129 - [c52]Ulf Johansson, Rikard König, Tuve Löfström
, Henrik Boström:
Evolved decision trees as conformal predictors. IEEE Congress on Evolutionary Computation 2013: 1794-1801 - [c51]Ulf Johansson, Tuve Löfström
, Henrik Boström:
Overproduce-and-select: The grim reality. CIEL 2013: 52-59 - [c50]Ulf Johansson, Henrik Boström, Tuve Löfström
:
Conformal Prediction Using Decision Trees. ICDM 2013: 330-339 - [c49]Ulf Johansson, Tuve Löfström
, Henrik Boström:
Random brains. IJCNN 2013: 1-8 - [c48]Tuve Löfström
, Ulf Johansson, Henrik Boström:
Effective utilization of data in inductive conformal prediction using ensembles of neural networks. IJCNN 2013: 1-8 - [c47]Amos H. C. Ng, Catarina Dudas, Henrik Boström, Kalyanmoy Deb:
Interleaving Innovization with Evolutionary Multi-Objective Optimization in Production System Simulation for Faster Convergence. LION 2013: 1-18 - 2012
- [j7]Ulf Johansson, Cecilia Sönströd, Tuve Löfström
, Henrik Boström:
Obtaining accurate and comprehensible classifiers using oracle coaching. Intell. Data Anal. 16(2): 247-263 (2012) - [j6]Henrik Boström:
Forests of Probability Estimation Trees. Int. J. Pattern Recognit. Artif. Intell. 26(2) (2012) - [j5]Ulf Norinder
, Henrik Boström:
Introducing Uncertainty in Predictive Modeling - Friend or Foe? J. Chem. Inf. Model. 52(11): 2815-2822 (2012) - [c46]Sampath Deegalla, Henrik Boström, Keerthi Walgama:
Choice of dimensionality reduction methods for feature and classifier fusion with nearest neighbor classifiers. FUSION 2012: 875-881 - [c45]Thashmee Karunaratne, Henrik Boström:
Can Frequent Itemset Mining Be Efficiently and Effectively Used for Learning from Graph Data? ICMLA (1) 2012: 409-414 - [c44]Constantino Sotomane, Jordi Gallego-Ayala, Lars Asker, Henrik Boström, Venâncio Massingue:
Extracting Patterns from Socioeconomic Databases to Characterize Small Farmers with High and Low Corn Yields in Mozambique: a Data Mining Approach. ICDM (Workshops) 2012: 99-108 - 2011
- [c43]Henrik Boström:
Concurrent Learning of Large-Scale Random Forests. SCAI 2011: 20-29 - 2010
- [c42]Thashmee Karunaratne, Henrik Boström, Ulf Norinder
:
Pre-Processing Structured Data for Standard Machine Learning Algorithms by Supervised Graph Propositionalization - A Case Study with Medicinal Chemistry Datasets. ICMLA 2010: 828-833 - [c41]Tuve Löfström
, Ulf Johansson, Henrik Boström:
Comparing methods for generating diverse ensembles of artificial neural networks. IJCNN 2010: 1-6 - [c40]Cecilia Sönströd, Ulf Johansson, Henrik Boström, Ulf Norinder
:
Pin-pointing concept descriptions. SMC 2010: 2956-2963
2000 – 2009
- 2009
- [c39]Tuve Löfström
, Ulf Johansson, Henrik Boström:
Ensemble member selection using multi-objective optimization. CIDM 2009: 245-251 - [c38]Sampath Deegalla, Henrik Boström:
Fusion of dimensionality reduction methods: A case study in microarray classification. FUSION 2009: 460-465 - [c37]Thashmee Karunaratne, Henrik Boström:
Graph Propositionalization for Random Forests. ICMLA 2009: 196-201 - [c36]Sampath Deegalla, Henrik Boström:
Improving Fusion of Dimensionality Reduction Methods for Nearest Neighbor Classification. ICMLA 2009: 771-775 - [c35]Catarina Dudas, Henrik Boström:
Using uncertain chemical and thermal data to predict product quality in a casting process. KDD Workshop on Knowledge Discovery from Uncertain Data 2009: 57-61 - 2008
- [c34]Ulf Johansson, Henrik Boström, Rikard König:
Extending Nearest Neighbor Classification with Spheres of Confidence. FLAIRS 2008: 282-287 - [c33]Henrik Boström, Ronnie Johansson, Alexander Karlsson:
On evidential combination rules for ensemble classifiers. FUSION 2008: 1-8 - [c32]Henrik Boström:
Calibrating Random Forests. ICMLA 2008: 121-126 - [c31]Tuve Löfström
, Ulf Johansson, Henrik Boström:
On the Use of Accuracy and Diversity Measures for Evaluating and Selecting Ensembles of Classifiers. ICMLA 2008: 127-132 - [c30]Cecilia Sönströd, Ulf Johansson, Ulf Norinder
, Henrik Boström:
Comprehensible Models for Predicting Molecular Interaction with Heart-Regulating Genes. ICMLA 2008: 559-564 - [c29]Ulf Johansson, Tuve Löfström
, Henrik Boström:
The problem with ranking ensembles based on training or validation performance. IJCNN 2008: 3222-3228 - [c28]Ronnie Johansson, Henrik Boström, Alexander Karlsson:
A study on class-specifically discounted belief for ensemble classifiers. MFI 2008: 614-619 - [c27]Ulf Johansson, Cecilia Sönströd, Tuve Löfström, Henrik Boström:
Chipper - A Novel Algorithm for Concept Description. SCAI 2008: 133-140 - 2007
- [c26]Henrik Boström:
Feature vs. classifier fusion for predictive data mining a case study in pesticide classification. FUSION 2007: 1-7 - [c25]Henrik Boström:
Estimating class probabilities in random forests. ICMLA 2007: 211-216 - [c24]Sampath Deegalla, Henrik Boström:
Classification of Microarrays with kNN: Comparison of Dimensionality Reduction Methods. IDEAL 2007: 800-809 - [c23]Thashmee Karunaratne, Henrik Boström:
Using Background Knowledge for Graph Based Learning: A Case Study in Chemoinformatics. IMECS 2007: 153-157 - [c22]Henrik Boström:
Maximizing the Area under the ROC Curve with Decision Lists and Rule Sets. SDM 2007: 27-34 - 2006
- [c21]Thashmee Karunaratne, Henrik Boström:
Learning to classify structured data by graph propositionalization. Computational Intelligence 2006: 283-288 - [c20]Sampath Deegalla, Henrik Boström:
Reducing High-Dimensional Data by Principal Component Analysis vs. Random Projection for Nearest Neighbor Classification. ICMLA 2006: 245-250 - 2004
- [j4]Tony Lindgren, Henrik Boström:
Resolving rule conflicts with double induction. Intell. Data Anal. 8(5): 457-468 (2004) - 2003
- [c19]Tony Lindgren, Henrik Boström:
Resolving Rule Conflicts with Double Induction. IDA 2003: 60-67 - 2002
- [c18]Tony Lindgren, Henrik Boström:
Classification with Intersecting Rules. ALT 2002: 395-402 - [c17]Per Lidén, Lars Asker, Henrik Boström:
Rule Induction for Classification of Gene Expression Array Data. PKDD 2002: 338-347 - 2001
- [j3]Juan J. Rodríguez Diez, Carlos Alonso González, Henrik Boström:
Boosting interval based literals. Intell. Data Anal. 5(3): 245-262 (2001) - [c16]Anette Hulth, Jussi Karlgren, Anna Jonsson, Henrik Boström, Lars Asker:
Automatic Keyword Extraction Using Domain Knowledge. CICLing 2001: 472-482 - [c15]Martin Eineborg, Henrik Boström:
Classifying Uncovered Examples by Rule Stretching. ILP 2001: 41-50 - [c14]Mikael Huss, Henrik Boström, Lars Asker, Joakim Cöster:
Learning to recognize brain specific proteins based on low-level features from on-line prediction servers. BIOKDD 2001: 45-49 - 2000
- [c13]Juan José Rodríguez, Carlos J. Alonso, Henrik Boström:
Learning First Order Logic Time Series Classifiers. ILP Work-in-progress reports 2000 - [c12]Juan J. Rodríguez Diez, Carlos Alonso González, Henrik Boström:
Learning First Order Logic Time Series Classifiers: Rules and Boosting. PKDD 2000: 299-308
1990 – 1999
- 1999
- [j2]Henrik Boström, Peter Idestam-Almquist:
Induction of Logic Programs by Example-Guided Unfolding. J. Log. Program. 40(2-3): 159-183 (1999) - [c11]Henrik Boström, Lars Asker:
Combining Divide-and-Conquer and Separate-and-Conquer for Efficient and Effective Rule Induction. ILP 1999: 33-43 - [c10]Henrik Boström:
Induction of Recursive Transfer Rules. Learning Language in Logic 1999: 237-246 - 1998
- [c9]Henrik Boström:
Predicate Invention and Learning from Positive Examples Only. ECML 1998: 226-237 - 1997
- [j1]Zoltán Alexin, Tibor Gyimóthy, Henrik Boström:
IMPUT: An Interactive Learning Tool Based on Program Specialization. Intell. Data Anal. 1(1-4): 219-244 (1997) - 1996
- [c8]Zoltán Alexin, Tibor Gyimóthy, Henrik Boström:
Integrating Algorithmic Debugging and Unfolding Transformation in an Interactive Learner. ECAI 1996: 403-407 - [c7]Henrik Boström:
Theory-Guideed Induction of Logic Programs by Inference of Regular Languages. ICML 1996: 46-53 - 1995
- [c6]Henrik Boström:
Specialization of Recursive Predicates. ECML 1995: 92-106 - [c5]Hilde Adé, Henrik Boström:
JIGSAW: Puzzling together RUTH and SPECTRE (Extended Abstract). ECML 1995: 263-266 - [c4]Henrik Boström:
Covering vs. Divide-and-Conquer for Top-Down Induction of Logic Programs. IJCAI 1995: 1194-1200 - 1993
- [c3]Henrik Boström:
Improving Example-Guided Unfolding. ECML 1993: 124-135 - 1991
- [c2]Carl Gustaf Jansson, Henrik Boström, Peter Idestam-Almquist:
Optimizing Horn Clause Logic Programs for Particular Modes of Use: An Analysis of Explanation-Based Learning and Partial Evaluation. SCAI 1991: 252-257 - 1990
- [c1]Henrik Boström:
Generalizing the Order of Goals as an Approach to Generalizing Number. ML 1990: 260-267
Coauthor Index

manage site settings
To protect your privacy, all features that rely on external API calls from your browser are turned off by default. You need to opt-in for them to become active. All settings here will be stored as cookies with your web browser. For more information see our F.A.Q.
Unpaywalled article links
Add open access links from to the list of external document links (if available).
Privacy notice: By enabling the option above, your browser will contact the API of unpaywall.org to load hyperlinks to open access articles. Although we do not have any reason to believe that your call will be tracked, we do not have any control over how the remote server uses your data. So please proceed with care and consider checking the Unpaywall privacy policy.
Archived links via Wayback Machine
For web page which are no longer available, try to retrieve content from the of the Internet Archive (if available).
Privacy notice: By enabling the option above, your browser will contact the API of archive.org to check for archived content of web pages that are no longer available. Although we do not have any reason to believe that your call will be tracked, we do not have any control over how the remote server uses your data. So please proceed with care and consider checking the Internet Archive privacy policy.
Reference lists
Add a list of references from ,
, and
to record detail pages.
load references from crossref.org and opencitations.net
Privacy notice: By enabling the option above, your browser will contact the APIs of crossref.org, opencitations.net, and semanticscholar.org to load article reference information. Although we do not have any reason to believe that your call will be tracked, we do not have any control over how the remote server uses your data. So please proceed with care and consider checking the Crossref privacy policy and the OpenCitations privacy policy, as well as the AI2 Privacy Policy covering Semantic Scholar.
Citation data
Add a list of citing articles from and
to record detail pages.
load citations from opencitations.net
Privacy notice: By enabling the option above, your browser will contact the API of opencitations.net and semanticscholar.org to load citation information. Although we do not have any reason to believe that your call will be tracked, we do not have any control over how the remote server uses your data. So please proceed with care and consider checking the OpenCitations privacy policy as well as the AI2 Privacy Policy covering Semantic Scholar.
OpenAlex data
Load additional information about publications from .
Privacy notice: By enabling the option above, your browser will contact the API of openalex.org to load additional information. Although we do not have any reason to believe that your call will be tracked, we do not have any control over how the remote server uses your data. So please proceed with care and consider checking the information given by OpenAlex.
last updated on 2024-10-28 20:15 CET by the dblp team
all metadata released as open data under CC0 1.0 license
see also: Terms of Use | Privacy Policy | Imprint