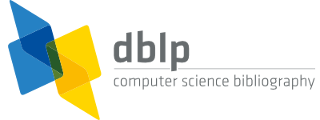


default search action
Markus Heinonen
Person information
Refine list

refinements active!
zoomed in on ?? of ?? records
view refined list in
export refined list as
2020 – today
- 2024
- [c34]Yasmine Nahal, Markus Heinonen, Mikhail Kabeshov, Jon Paul Janet, Eva Nittinger, Ola Engkvist, Samuel Kaski:
Towards Interpretable Models of Chemist Preferences for Human-in-the-Loop Assisted Drug Discovery. AIDD@ICANN 2024: 58-70 - [c33]Muhammad Arslan Masood
, Samuel Kaski
, Hugo Ceulemans
, Dorota Herman
, Markus Heinonen
:
Balancing Imbalanced Toxicity Models: Using MolBERT with Focal Loss. AIDD@ICANN 2024: 82-97 - [c32]Trung Q. Trinh, Markus Heinonen, Luigi Acerbi, Samuel Kaski:
Input-gradient space particle inference for neural network ensembles. ICLR 2024 - [c31]Yogesh Verma, Markus Heinonen, Vikas Garg:
ClimODE: Climate and Weather Forecasting with Physics-informed Neural ODEs. ICLR 2024 - [i43]Alexandru Dumitrescu, Dani Korpela, Markus Heinonen, Yogesh Verma, Valerii Iakovlev, Vikas Garg, Harri Lähdesmäki:
Field-based Molecule Generation. CoRR abs/2402.15864 (2024) - [i42]Yogesh Verma, Markus Heinonen, Vikas Garg:
ClimODE: Climate and Weather Forecasting with Physics-informed Neural ODEs. CoRR abs/2404.10024 (2024) - [i41]Najwa Laabid, Severi Rissanen, Markus Heinonen, Arno Solin, Vikas Garg:
Alignment is Key for Applying Diffusion Models to Retrosynthesis. CoRR abs/2405.17656 (2024) - [i40]Severi Rissanen, Markus Heinonen, Arno Solin:
Improving Discrete Diffusion Models via Structured Preferential Generation. CoRR abs/2405.17889 (2024) - [i39]Markus Heinonen, Ba-Hien Tran, Michael Kampffmeyer, Maurizio Filippone:
Robust Classification by Coupling Data Mollification with Label Smoothing. CoRR abs/2406.01494 (2024) - [i38]Trung Trinh, Markus Heinonen, Luigi Acerbi, Samuel Kaski:
Improving robustness to corruptions with multiplicative weight perturbations. CoRR abs/2406.16540 (2024) - [i37]Rafal Karczewski, Samuel Kaski, Markus Heinonen, Vikas Garg:
What Ails Generative Structure-based Drug Design: Too Little or Too Much Expressivity? CoRR abs/2408.06050 (2024) - 2023
- [j13]Emmi Jokinen
, Alexandru Dumitrescu
, Jani Huuhtanen, Vladimir Gligorijevic, Satu Mustjoki
, Richard Bonneau, Markus Heinonen, Harri Lähdesmäki:
TCRconv: predicting recognition between T cell receptors and epitopes using contextualized motifs. Bioinform. 39(1) (2023) - [j12]Magnus Ross, Markus Heinonen:
Learning Energy Conserving Dynamics Efficiently with Hamiltonian Gaussian Processes. Trans. Mach. Learn. Res. 2023 (2023) - [j11]Oliver Struckmeier, Ievgen Redko, Anton Mallasto, Karol Arndt, Markus Heinonen, Ville Kyrki:
Learning representations that are closed-form Monge mapping optimal with application to domain adaptation. Trans. Mach. Learn. Res. 2023 (2023) - [c30]Vishnu Raj, Tianyu Cui, Markus Heinonen, Pekka Marttinen:
Incorporating functional summary information in Bayesian neural networks using a Dirichlet process likelihood approach. AISTATS 2023: 6741-6763 - [c29]Valerii Iakovlev, Çagatay Yildiz, Markus Heinonen, Harri Lähdesmäki:
Latent Neural ODEs with Sparse Bayesian Multiple Shooting. ICLR 2023 - [c28]Severi Rissanen, Markus Heinonen, Arno Solin:
Generative Modelling with Inverse Heat Dissipation. ICLR 2023 - [c27]Yogesh Verma, Markus Heinonen, Vikas Garg:
AbODE: Ab initio antibody design using conjoined ODEs. ICML 2023: 35037-35050 - [c26]Giulio Franzese, Giulio Corallo, Simone Rossi, Markus Heinonen, Maurizio Filippone, Pietro Michiardi:
Continuous-Time Functional Diffusion Processes. NeurIPS 2023 - [c25]Valerii Iakovlev, Markus Heinonen, Harri Lähdesmäki:
Learning Space-Time Continuous Latent Neural PDEs from Partially Observed States. NeurIPS 2023 - [c24]Aarne Talman, Hande Çelikkanat, Sami Virpioja, Markus Heinonen, Jörg Tiedemann:
Uncertainty-Aware Natural Language Inference with Stochastic Weight Averaging. NoDaLiDa 2023: 358-365 - [i36]Giulio Franzese, Simone Rossi, Dario Rossi, Markus Heinonen, Maurizio Filippone, Pietro Michiardi:
Continuous-Time Functional Diffusion Processes. CoRR abs/2303.00800 (2023) - [i35]Magnus Ross, Markus Heinonen:
Learning Energy Conserving Dynamics Efficiently with Hamiltonian Gaussian Processes. CoRR abs/2303.01925 (2023) - [i34]Aarne Talman, Hande Çelikkanat, Sami Virpioja, Markus Heinonen, Jörg Tiedemann:
Uncertainty-Aware Natural Language Inference with Stochastic Weight Averaging. CoRR abs/2304.04726 (2023) - [i33]Oliver Struckmeier, Ievgen Redko, Anton Mallasto, Karol Arndt, Markus Heinonen, Ville Kyrki:
Beyond invariant representation learning: linearly alignable latent spaces for efficient closed-form domain adaptation. CoRR abs/2305.07500 (2023) - [i32]Yogesh Verma, Markus Heinonen, Vikas Garg:
AbODE: Ab Initio Antibody Design using Conjoined ODEs. CoRR abs/2306.01005 (2023) - [i31]Trung Q. Trinh, Markus Heinonen, Luigi Acerbi, Samuel Kaski:
Input gradient diversity for neural network ensembles. CoRR abs/2306.02775 (2023) - [i30]Valerii Iakovlev, Markus Heinonen, Harri Lähdesmäki:
Learning Space-Time Continuous Neural PDEs from Partially Observed States. CoRR abs/2307.04110 (2023) - [i29]Quentin Bouniot, Ievgen Redko, Anton Mallasto, Charlotte Laclau, Karol Arndt, Oliver Struckmeier, Markus Heinonen, Ville Kyrki, Samuel Kaski:
Understanding deep neural networks through the lens of their non-linearity. CoRR abs/2310.11439 (2023) - 2022
- [j10]Anni A. Antikainen
, Markus Heinonen, Harri Lähdesmäki:
Modeling binding specificities of transcription factor pairs with random forests. BMC Bioinform. 23(1): 212 (2022) - [j9]Alexander Aushev
, Henri Pesonen, Markus Heinonen, Jukka Corander
, Samuel Kaski
:
Likelihood-free inference with deep Gaussian processes. Comput. Stat. Data Anal. 174: 107529 (2022) - [j8]Iiris Sundin
, Alexey Voronov
, Haoping Xiao, Kostas Papadopoulos
, Esben Jannik Bjerrum, Markus Heinonen, Atanas Patronov, Samuel Kaski, Ola Engkvist:
Human-in-the-loop assisted de novo molecular design. J. Cheminformatics 14(1): 86 (2022) - [c23]Trung Q. Trinh
, Markus Heinonen, Luigi Acerbi, Samuel Kaski:
Tackling covariate shift with node-based Bayesian neural networks. ICML 2022: 21751-21775 - [c22]Yogesh Verma, Samuel Kaski, Markus Heinonen, Vikas Garg:
Modular Flows: Differential Molecular Generation. NeurIPS 2022 - [c21]Pashupati Hegde, Çagatay Yildiz, Harri Lähdesmäki, Samuel Kaski, Markus Heinonen:
Variational multiple shooting for Bayesian ODEs with Gaussian processes. UAI 2022: 790-799 - [i28]Trung Q. Trinh, Markus Heinonen, Luigi Acerbi, Samuel Kaski:
Tackling covariate shift with node-based Bayesian neural networks. CoRR abs/2206.02435 (2022) - [i27]Severi Rissanen, Markus Heinonen, Arno Solin:
Generative Modelling With Inverse Heat Dissipation. CoRR abs/2206.13397 (2022) - [i26]Vishnu Raj, Tianyu Cui, Markus Heinonen, Pekka Marttinen:
Look beyond labels: Incorporating functional summary information in Bayesian neural networks. CoRR abs/2207.01234 (2022) - [i25]Valerii Iakovlev, Çagatay Yildiz, Markus Heinonen, Harri Lähdesmäki:
Latent Neural ODEs with Sparse Bayesian Multiple Shooting. CoRR abs/2210.03466 (2022) - [i24]Yogesh Verma, Samuel Kaski, Markus Heinonen, Vikas Garg:
Modular Flows: Differential Molecular Generation. CoRR abs/2210.06032 (2022) - [i23]David Duvenaud, Markus Heinonen, Michael Tiemann, Max Welling:
Differential Equations and Continuous-Time Deep Learning (Dagstuhl Seminar 22332). Dagstuhl Reports 12(8): 20-30 (2022) - 2021
- [j7]Emmi Jokinen
, Jani Huuhtanen
, Satu Mustjoki
, Markus Heinonen, Harri Lähdesmäki:
Predicting recognition between T cell receptors and epitopes with TCRGP. PLoS Comput. Biol. 17(3) (2021) - [c20]Anton Mallasto, Markus Heinonen, Samuel Kaski:
Bayesian Inference for Optimal Transport with Stochastic Cost. ACML 2021: 1601-1616 - [c19]Simone Rossi, Markus Heinonen, Edwin V. Bonilla, Zheyang Shen, Maurizio Filippone:
Sparse Gaussian Processes Revisited: Bayesian Approaches to Inducing-Variable Approximations. AISTATS 2021: 1837-1845 - [c18]Valerii Iakovlev, Markus Heinonen, Harri Lähdesmäki:
Learning continuous-time PDEs from sparse data with graph neural networks. ICLR 2021 - [c17]Çagatay Yildiz, Markus Heinonen, Harri Lähdesmäki:
Continuous-time Model-based Reinforcement Learning. ICML 2021: 12009-12018 - [c16]Zheyang Shen, Markus Heinonen, Samuel Kaski:
De-randomizing MCMC dynamics with the diffusion Stein operator. NeurIPS 2021: 17507-17517 - [i22]Çagatay Yildiz, Markus Heinonen, Harri Lähdesmäki:
Continuous-Time Model-Based Reinforcement Learning. CoRR abs/2102.04764 (2021) - [i21]Anton Mallasto, Karol Arndt, Markus Heinonen, Samuel Kaski, Ville Kyrki:
Affine Transport for Sim-to-Real Domain Adaptation. CoRR abs/2105.11739 (2021) - [i20]Pashupati Hegde, Çagatay Yildiz, Harri Lähdesmäki, Samuel Kaski, Markus Heinonen:
Bayesian inference of ODEs with Gaussian processes. CoRR abs/2106.10905 (2021) - [i19]David Blanco Mulero, Markus Heinonen, Ville Kyrki:
Evolving-Graph Gaussian Processes. CoRR abs/2106.15127 (2021) - [i18]Zheyang Shen, Markus Heinonen, Samuel Kaski:
De-randomizing MCMC dynamics with the diffusion Stein operator. CoRR abs/2110.03768 (2021) - 2020
- [c15]Zheyang Shen, Markus Heinonen, Samuel Kaski:
Learning spectrograms with convolutional spectral kernels. AISTATS 2020: 3826-3836 - [i17]Simone Rossi, Markus Heinonen, Edwin V. Bonilla, Zheyang Shen, Maurizio Filippone:
Rethinking Sparse Gaussian Processes: Bayesian Approaches to Inducing-Variable Approximations. CoRR abs/2003.03080 (2020) - [i16]Valerii Iakovlev, Markus Heinonen, Harri Lähdesmäki:
Learning continuous-time PDEs from sparse data with graph neural networks. CoRR abs/2006.08956 (2020) - [i15]Alexander Aushev, Henri Pesonen, Markus Heinonen, Jukka Corander, Samuel Kaski:
Likelihood-Free Inference with Deep Gaussian Processes. CoRR abs/2006.10571 (2020) - [i14]Anton Mallasto, Markus Heinonen, Samuel Kaski:
Bayesian Inference for Optimal Transport with Stochastic Cost. CoRR abs/2010.09327 (2020) - [i13]Trung Q. Trinh, Samuel Kaski, Markus Heinonen:
Scalable Bayesian neural networks by layer-wise input augmentation. CoRR abs/2010.13498 (2020) - [i12]Charles W. L. Gadd, Markus Heinonen, Harri Lähdesmäki, Samuel Kaski:
Sample-efficient reinforcement learning using deep Gaussian processes. CoRR abs/2011.01226 (2020)
2010 – 2019
- 2019
- [j6]Markus Heinonen, Maria Osmala
, Henrik Mannerström
, Janne Wallenius
, Samuel Kaski, Juho Rousu, Harri Lähdesmäki:
Bayesian metabolic flux analysis reveals intracellular flux couplings. Bioinform. 35(14): i548-i557 (2019) - [c14]Pashupati Hegde, Markus Heinonen, Harri Lähdesmäki, Samuel Kaski:
Deep learning with differential Gaussian process flows. AISTATS 2019: 1812-1821 - [c13]Zheyang Shen, Markus Heinonen, Samuel Kaski:
Harmonizable mixture kernels with variational Fourier features. AISTATS 2019: 3273-3282 - [c12]Çagatay Yildiz, Markus Heinonen, Harri Lähdesmäki:
ODE2VAE: Deep generative second order ODEs with Bayesian neural networks. NeurIPS 2019: 13412-13421 - [c11]Kenneth Blomqvist, Samuel Kaski, Markus Heinonen:
Deep Convolutional Gaussian Processes. ECML/PKDD (2) 2019: 582-597 - [i11]Zheyang Shen, Markus Heinonen, Samuel Kaski:
Learning spectrograms with convolutional spectral kernels. CoRR abs/1905.09917 (2019) - [i10]Çagatay Yildiz, Markus Heinonen, Harri Lähdesmäki:
ODE$^2$VAE: Deep generative second order ODEs with Bayesian neural networks. CoRR abs/1905.10994 (2019) - 2018
- [j5]Emmi Jokinen
, Markus Heinonen, Harri Lähdesmäki:
mGPfusion: predicting protein stability changes with Gaussian process kernel learning and data fusion. Bioinform. 34(13): i274-i283 (2018) - [j4]Anna Cichonska
, Tapio Pahikkala
, Sándor Szedmák
, Heli Julkunen
, Antti Airola
, Markus Heinonen, Tero Aittokallio
, Juho Rousu:
Learning with multiple pairwise kernels for drug bioactivity prediction. Bioinform. 34(13): i509-i518 (2018) - [c10]Markus Heinonen, Çagatay Yildiz, Henrik Mannerström, Jukka Intosalmi, Harri Lähdesmäki:
Learning unknown ODE models with Gaussian processes. ICML 2018: 1964-1973 - [c9]Çagatay Yildiz, Markus Heinonen, Jukka Intosalmi, Henrik Mannerström
, Harri Lähdesmäki:
Learning stochastic differential equations with Gaussian Processes without Gradient Matching. MLSP 2018: 1-6 - [c8]Pashupati Hegde, Markus Heinonen, Samuel Kaski:
Variational zero-inflated Gaussian processes with sparse kernels. UAI 2018: 361-371 - [i9]Markus Heinonen, Maria Osmala, Henrik Mannerström, Janne Wallenius, Samuel Kaski, Juho Rousu, Harri Lähdesmäki:
Bayesian Metabolic Flux Analysis reveals intracellular flux couplings. CoRR abs/1804.06673 (2018) - [i8]Çagatay Yildiz, Markus Heinonen, Jukka Intosalmi, Henrik Mannerström, Harri Lähdesmäki:
Learning Stochastic Differential Equations With Gaussian Processes Without Gradient Matching. CoRR abs/1807.05748 (2018) - [i7]Kenneth Blomqvist, Samuel Kaski, Markus Heinonen:
Deep convolutional Gaussian processes. CoRR abs/1810.03052 (2018) - [i6]Pashupati Hegde, Markus Heinonen, Harri Lähdesmäki, Samuel Kaski:
Deep learning with differential Gaussian process flows. CoRR abs/1810.04066 (2018) - [i5]Zheyang Shen, Markus Heinonen, Samuel Kaski:
Harmonizable mixture kernels with variational Fourier features. CoRR abs/1810.04416 (2018) - [i4]Sami Remes, Markus Heinonen, Samuel Kaski:
Neural Non-Stationary Spectral Kernel. CoRR abs/1811.10978 (2018) - 2017
- [c7]Sami Remes, Markus Heinonen, Samuel Kaski:
A Mutually-Dependent Hadamard Kernel for Modelling Latent Variable Couplings. ACML 2017: 455-470 - [c6]Sami Remes, Markus Heinonen, Samuel Kaski:
Non-Stationary Spectral Kernels. NIPS 2017: 4642-4651 - [i3]Sami Remes, Markus Heinonen, Samuel Kaski:
Non-Stationary Spectral Kernels. CoRR abs/1705.08736 (2017) - 2016
- [c5]Romain Brault, Markus Heinonen, Florence d'Alché-Buc:
Random Fourier Features For Operator-Valued Kernels. ACML 2016: 110-125 - [c4]Markus Heinonen, Henrik Mannerström, Juho Rousu, Samuel Kaski, Harri Lähdesmäki:
Non-Stationary Gaussian Process Regression with Hamiltonian Monte Carlo. AISTATS 2016: 732-740 - [i2]Romain Brault, Florence d'Alché-Buc, Markus Heinonen:
Random Fourier Features for Operator-Valued Kernels. CoRR abs/1605.02536 (2016) - 2015
- [j3]Markus Heinonen, Olivier Guipaud, Fabien Milliat
, Valérie Buard, Béatrice Micheau, Georges Tarlet
, Marc Benderitter, Farida Zehraoui, Florence d'Alché-Buc
:
Detecting time periods of differential gene expression using Gaussian processes: an application to endothelial cells exposed to radiotherapy dose fraction. Bioinform. 31(5): 728-735 (2015) - 2014
- [i1]Markus Heinonen, Florence d'Alché-Buc:
Learning nonparametric differential equations with operator-valued kernels and gradient matching. CoRR abs/1411.5172 (2014) - 2012
- [b1]Markus Heinonen:
Computational methods for small molecules. University of Helsinki, Finland, 2012 - [j2]Markus Heinonen, Huibin Shen, Nicola Zamboni
, Juho Rousu:
Metabolite identification and molecular fingerprint prediction through machine learning. Bioinform. 28(18): 2333-2341 (2012) - [c3]Markus Heinonen, Niko Välimäki, Veli Mäkinen, Juho Rousu:
Efficient Path Kernels for Reaction Function Prediction. BIOINFORMATICS 2012: 202-207 - 2011
- [j1]Markus Heinonen, Sampsa Lappalainen, Taneli Mielikäinen, Juho Rousu:
Computing Atom Mappings for Biochemical Reactions without Subgraph Isomorphism. J. Comput. Biol. 18(1): 43-58 (2011) - 2010
- [c2]Hongyu Su, Markus Heinonen, Juho Rousu:
Structured Output Prediction of Anti-cancer Drug Activity. PRIB 2010: 38-49
2000 – 2009
- 2006
- [c1]Markus Heinonen, Ari Rantanen, Taneli Mielikäinen, Esa Pitkänen, Juha Kokkonen, Juho Rousu:
Ab Initio Prediction of Molecular Fragments from Tandem Mass Spectrometry Data. German Conference on Bioinformatics 2006: 40-53
Coauthor Index

manage site settings
To protect your privacy, all features that rely on external API calls from your browser are turned off by default. You need to opt-in for them to become active. All settings here will be stored as cookies with your web browser. For more information see our F.A.Q.
Unpaywalled article links
Add open access links from to the list of external document links (if available).
Privacy notice: By enabling the option above, your browser will contact the API of unpaywall.org to load hyperlinks to open access articles. Although we do not have any reason to believe that your call will be tracked, we do not have any control over how the remote server uses your data. So please proceed with care and consider checking the Unpaywall privacy policy.
Archived links via Wayback Machine
For web page which are no longer available, try to retrieve content from the of the Internet Archive (if available).
Privacy notice: By enabling the option above, your browser will contact the API of archive.org to check for archived content of web pages that are no longer available. Although we do not have any reason to believe that your call will be tracked, we do not have any control over how the remote server uses your data. So please proceed with care and consider checking the Internet Archive privacy policy.
Reference lists
Add a list of references from ,
, and
to record detail pages.
load references from crossref.org and opencitations.net
Privacy notice: By enabling the option above, your browser will contact the APIs of crossref.org, opencitations.net, and semanticscholar.org to load article reference information. Although we do not have any reason to believe that your call will be tracked, we do not have any control over how the remote server uses your data. So please proceed with care and consider checking the Crossref privacy policy and the OpenCitations privacy policy, as well as the AI2 Privacy Policy covering Semantic Scholar.
Citation data
Add a list of citing articles from and
to record detail pages.
load citations from opencitations.net
Privacy notice: By enabling the option above, your browser will contact the API of opencitations.net and semanticscholar.org to load citation information. Although we do not have any reason to believe that your call will be tracked, we do not have any control over how the remote server uses your data. So please proceed with care and consider checking the OpenCitations privacy policy as well as the AI2 Privacy Policy covering Semantic Scholar.
OpenAlex data
Load additional information about publications from .
Privacy notice: By enabling the option above, your browser will contact the API of openalex.org to load additional information. Although we do not have any reason to believe that your call will be tracked, we do not have any control over how the remote server uses your data. So please proceed with care and consider checking the information given by OpenAlex.
last updated on 2024-10-07 21:22 CEST by the dblp team
all metadata released as open data under CC0 1.0 license
see also: Terms of Use | Privacy Policy | Imprint