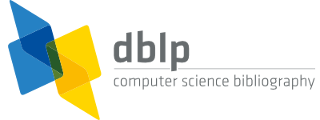


default search action
Jan N. van Rijn
Person information
- affiliation: University of Freiburg, Department of Computer Science, Germany
- affiliation: Leiden University, Leiden Institute of Advanced Computer Science, The Netherlands
Refine list

refinements active!
zoomed in on ?? of ?? records
view refined list in
export refined list as
2020 – today
- 2024
- [j19]Mitra Baratchi, Can Wang, Steffen Limmer, Jan N. van Rijn, Holger H. Hoos, Thomas Bäck, Markus Olhofer:
Automated machine learning: past, present and future. Artif. Intell. Rev. 57(5): 122 (2024) - [j18]Matthias König, Annelot W. Bosman, Holger H. Hoos, Jan N. van Rijn:
Critically Assessing the State of the Art in Neural Network Verification. J. Mach. Learn. Res. 25: 12:1-12:53 (2024) - [j17]Mike Huisman
, Aske Plaat, Jan N. van Rijn:
Subspace Adaptation Prior for Few-Shot Learning. Mach. Learn. 113(2): 725-752 (2024) - [j16]Charles Moussa
, Yash J. Patel
, Vedran Dunjko, Thomas Bäck, Jan N. van Rijn:
Hyperparameter importance and optimization of quantum neural networks across small datasets. Mach. Learn. 113(4): 1941-1966 (2024) - [j15]Mike Huisman
, Aske Plaat, Jan N. van Rijn:
Understanding transfer learning and gradient-based meta-learning techniques. Mach. Learn. 113(7): 4113-4132 (2024) - [j14]Ran Cheng
, Hugo Jair Escalante
, Wei-Wei Tu, Jan N. van Rijn
, Shuo Wang
, Yun Yang
:
Guest Editorial: AutoML for Nonstationary Data. IEEE Trans. Artif. Intell. 5(6): 2456-2457 (2024) - [c31]Matthias König, Holger H. Hoos, Jan N. van Rijn:
Accelerating Adversarially Robust Model Selection for Deep Neural Networks via Racing. AAAI 2024: 21267-21275 - [c30]Lionel Kielhöfer, Felix Mohr
, Jan N. van Rijn
:
Learning Curve Extrapolation Methods Across Extrapolation Settings. IDA (2) 2024: 145-157 - [c29]Matthias König, Xiyue Zhang, Holger H. Hoos, Marta Kwiatkowska, Jan N. van Rijn:
Automated Design of Linear Bounding Functions for Sigmoidal Nonlinearities in Neural Networks. ECML/PKDD (7) 2024: 383-398 - [c28]Annelot W. Bosman
, Anna L. Münz
, Holger H. Hoos
, Jan N. van Rijn
:
A Preliminary Study to Examining Per-class Performance Bias via Robustness Distributions. SAIV 2024: 116-133 - [i27]Romain Egele, Júlio C. S. Jacques Júnior, Jan N. van Rijn, Isabelle Guyon, Xavier Baró, Albert Clapés, Prasanna Balaprakash, Sergio Escalera, Thomas B. Moeslund, Jun Wan:
AI Competitions and Benchmarks: Dataset Development. CoRR abs/2404.09703 (2024) - [i26]Matthias König, Xiyue Zhang, Holger H. Hoos, Marta Kwiatkowska, Jan N. van Rijn:
Automated Design of Linear Bounding Functions for Sigmoidal Nonlinearities in Neural Networks. CoRR abs/2406.10154 (2024) - [i25]Inês Gomes, Luís F. Teixeira, Jan N. van Rijn, Carlos Soares, André Restivo, Luís Cunha, Moisés Santos:
Finding Patterns in Ambiguity: Interpretable Stress Testing in the Decision Boundary. CoRR abs/2408.06302 (2024) - [i24]Thijs Snelleman, Bram M. Renting, Holger H. Hoos, Jan N. van Rijn:
Edge-Based Graph Component Pooling. CoRR abs/2409.11856 (2024) - 2023
- [j13]Mike Huisman
, Thomas M. Moerland, Aske Plaat, Jan N. van Rijn:
Are LSTMs good few-shot learners? Mach. Learn. 112(11): 4635-4662 (2023) - [j12]Felix Mohr
, Jan N. van Rijn
:
Fast and Informative Model Selection Using Learning Curve Cross-Validation. IEEE Trans. Pattern Anal. Mach. Intell. 45(8): 9669-9680 (2023) - [c27]Matthias König, Annelot W. Bosman, Holger H. Hoos, Jan N. van Rijn:
Critically Assessing the State of the Art in CPU-based Local Robustness Verification. SafeAI@AAAI 2023 - [i23]Ihsan Ullah, Dustin Carrión-Ojeda, Sergio Escalera, Isabelle Guyon, Mike Huisman, Felix Mohr, Jan N. van Rijn, Haozhe Sun, Joaquin Vanschoren, Phan Anh Vu:
Meta-Album: Multi-domain Meta-Dataset for Few-Shot Image Classification. CoRR abs/2302.08909 (2023) - [i22]Devis Tuia, Konrad Schindler, Begüm Demir, Gustau Camps-Valls, Xiao Xiang Zhu, Mrinalini Kochupillai, Saso Dzeroski, Jan N. van Rijn, Holger H. Hoos, Fabio Del Frate, Mihai Datcu, Jorge-Arnulfo Quiané-Ruiz, Volker Markl, Bertrand Le Saux, Rochelle Schneider:
Artificial intelligence to advance Earth observation: a perspective. CoRR abs/2305.08413 (2023) - [i21]Mike Huisman, Aske Plaat, Jan N. van Rijn:
Understanding Transfer Learning and Gradient-Based Meta-Learning Techniques. CoRR abs/2310.06148 (2023) - [i20]Mike Huisman, Aske Plaat, Jan N. van Rijn:
Subspace Adaptation Prior for Few-Shot Learning. CoRR abs/2310.09028 (2023) - [i19]Mike Huisman, Thomas M. Moerland, Aske Plaat, Jan N. van Rijn:
Are LSTMs Good Few-Shot Learners? CoRR abs/2310.14139 (2023) - 2022
- [j11]Mike Huisman
, Aske Plaat, Jan N. van Rijn:
Stateless neural meta-learning using second-order gradients. Mach. Learn. 111(9): 3227-3244 (2022) - [j10]Matthias König, Holger H. Hoos, Jan N. van Rijn:
Speeding up neural network robustness verification via algorithm configuration and an optimised mixed integer linear programming solver portfolio. Mach. Learn. 111(12): 4565-4584 (2022) - [c26]Charles Moussa
, Jan N. van Rijn
, Thomas Bäck
, Vedran Dunjko
:
Hyperparameter Importance of Quantum Neural Networks Across Small Datasets. DS 2022: 32-46 - [c25]Pavel Brazdil, Jan N. van Rijn, Henry Gouk, Felix Mohr:
Advances in Metalearning: ECML/PKDD Workshop on Meta-Knowledge Transfer. Meta-Knowledge Transfer @ ECML/PKDD 2022: 1-7 - [c24]Ihsan Ullah, Dustin Carrión-Ojeda, Sergio Escalera, Isabelle Guyon, Mike Huisman, Felix Mohr, Jan N. van Rijn, Haozhe Sun, Joaquin Vanschoren, Phan Anh Vu:
Meta-Album: Multi-domain Meta-Dataset for Few-Shot Image Classification. NeurIPS 2022 - [c23]Felix Mohr, Tom J. Viering, Marco Loog, Jan N. van Rijn:
LCDB 1.0: An Extensive Learning Curves Database for Classification Tasks. ECML/PKDD (5) 2022: 3-19 - [e3]Pavel Brazdil, Jan N. van Rijn, Henry Gouk, Felix Mohr:
ECML/PKDD Workshop on Meta-Knowledge Transfer, 23 September 2022, Grenoble, France. Proceedings of Machine Learning Research 191, PMLR 2022 [contents] - [i18]Felix Mohr, Jan N. van Rijn:
Learning Curves for Decision Making in Supervised Machine Learning - A Survey. CoRR abs/2201.12150 (2022) - [i17]Adrian El Baz, Isabelle Guyon, Zhengying Liu, Jan N. van Rijn, Sébastien Treguer, Joaquin Vanschoren:
Advances in MetaDL: AAAI 2021 challenge and workshop. CoRR abs/2202.01890 (2022) - [i16]Adrian El Baz, André C. P. L. F. de Carvalho, Hong Chen, Fabio Ferreira, Henry Gouk, Shell Hu, Frank Hutter, Zhengying Liu, Felix Mohr, Jan N. van Rijn, Xin Wang, Isabelle Guyon:
Lessons learned from the NeurIPS 2021 MetaDL challenge: Backbone fine-tuning without episodic meta-learning dominates for few-shot learning image classification. CoRR abs/2206.08138 (2022) - [i15]Charles Moussa, Jan N. van Rijn, Thomas Bäck, Vedran Dunjko:
Hyperparameter Importance of Quantum Neural Networks Across Small Datasets. CoRR abs/2206.09992 (2022) - 2021
- [j9]Mike Huisman
, Jan N. van Rijn
, Aske Plaat
:
A survey of deep meta-learning. Artif. Intell. Rev. 54(6): 4483-4541 (2021) - [j8]Matthias Feurer, Jan N. van Rijn, Arlind Kadra, Pieter Gijsbers, Neeratyoy Mallik, Sahithya Ravi, Andreas Müller, Joaquin Vanschoren, Frank Hutter:
OpenML-Python: an extensible Python API for OpenML. J. Mach. Learn. Res. 22: 100:1-100:5 (2021) - [c22]Adrian El Baz, Isabelle Guyon, Zhengying Liu, Jan N. van Rijn, Sébastien Treguer
, Joaquin Vanschoren:
Advances in MetaDL: AAAI 2021 Challenge and Workshop. MetaDL@AAAI 2021: 1-16 - [c21]Chitsutha Soomlek
, Jan N. van Rijn
, Marcello M. Bonsangue
:
Automatic Human-Like Detection of Code Smells. DS 2021: 19-28 - [c20]Pieter Gijsbers, Florian Pfisterer, Jan N. van Rijn, Bernd Bischl, Joaquin Vanschoren:
Meta-learning for symbolic hyperparameter defaults. GECCO Companion 2021: 151-152 - [c19]Florian Pfisterer, Jan N. van Rijn, Philipp Probst, Andreas C. Müller, Bernd Bischl:
Learning multiple defaults for machine learning algorithms. GECCO Companion 2021: 241-242 - [c18]Adrian El Baz, Ihsan Ullah, Edesio Alcobaça, André C. P. L. F. de Carvalho
, Hong Chen, Fabio Ferreira, Henry Gouk, Chaoyu Guan, Isabelle Guyon, Timothy M. Hospedales, Shell Hu, Mike Huisman, Frank Hutter, Zhengying Liu, Felix Mohr, Ekrem Öztürk, Jan N. van Rijn, Haozhe Sun
, Xin Wang, Wenwu Zhu:
Lessons learned from the NeurIPS 2021 MetaDL challenge: Backbone fine-tuning without episodic meta-learning dominates for few-shot learning image classification. NeurIPS (Competition and Demos) 2021: 80-96 - [c17]Bernd Bischl, Giuseppe Casalicchio, Matthias Feurer, Pieter Gijsbers, Frank Hutter, Michel Lang
, Rafael Gomes Mantovani, Jan N. van Rijn, Joaquin Vanschoren:
OpenML Benchmarking Suites. NeurIPS Datasets and Benchmarks 2021 - [c16]Nelly Rosaura Palacios Salinas, Mitra Baratchi
, Jan N. van Rijn
, Andreas Vollrath:
Automated Machine Learning for Satellite Data: Integrating Remote Sensing Pre-trained Models into AutoML Systems. ECML/PKDD (5) 2021: 447-462 - [e2]Isabelle Guyon, Jan N. van Rijn, Sébastien Treguer, Joaquin Vanschoren:
AAAI Workshop on Meta-Learning and MetaDL Challenge, MetaDL@AAAI 2021, virtual, February 9, 2021. Proceedings of Machine Learning Research 140, PMLR 2021 [contents] - [e1]Mitra Baratchi
, Lu Cao
, Walter A. Kosters
, Jefrey Lijffijt
, Jan N. van Rijn
, Frank W. Takes
:
Artificial Intelligence and Machine Learning - 32nd Benelux Conference, BNAIC/Benelearn 2020, Leiden, The Netherlands, November 19-20, 2020, Revised Selected Papers. Communications in Computer and Information Science 1398, Springer 2021, ISBN 978-3-030-76639-9 [contents] - [i14]Mike Huisman, Aske Plaat, Jan N. van Rijn:
Stateless Neural Meta-Learning using Second-Order Gradients. CoRR abs/2104.10527 (2021) - [i13]Pieter Gijsbers, Florian Pfisterer, Jan N. van Rijn, Bernd Bischl, Joaquin Vanschoren:
Meta-Learning for Symbolic Hyperparameter Defaults. CoRR abs/2106.05767 (2021) - [i12]Felix Mohr, Jan N. van Rijn:
Fast and Informative Model Selection using Learning Curve Cross-Validation. CoRR abs/2111.13914 (2021) - 2020
- [c15]Jeannette Shijie Ma, Marcello A. Gómez Maureira
, Jan N. van Rijn:
Eating Sound Dataset for 20 Food Types and Sound Classification Using Convolutional Neural Networks. ICMI Companion 2020: 348-351 - [i11]Mike Huisman, Jan N. van Rijn, Aske Plaat:
A Survey of Deep Meta-Learning. CoRR abs/2010.03522 (2020)
2010 – 2019
- 2019
- [j7]Marius Lindauer
, Jan N. van Rijn, Lars Kotthoff
:
The algorithm selection competitions 2015 and 2017. Artif. Intell. 272: 86-100 (2019) - [j6]Noureddin Sadawi
, Iván Olier
, Joaquin Vanschoren
, Jan N. van Rijn, Jeremy Besnard, G. Richard J. Bickerton, Crina Grosan
, Larisa N. Soldatova
, Ross D. King:
Multi-task learning with a natural metric for quantitative structure activity relationship learning. J. Cheminformatics 11(1): 68:1-68:13 (2019) - [c14]Abhinav Sharma, Jan N. van Rijn, Frank Hutter, Andreas Müller:
Hyperparameter Importance for Image Classification by Residual Neural Networks. DS 2019: 112-126 - [i10]Matthias Feurer, Jan N. van Rijn, Arlind Kadra, Pieter Gijsbers, Neeratyoy Mallik, Sahithya Ravi, Andreas Müller, Joaquin Vanschoren, Frank Hutter:
OpenML-Python: an extensible Python API for OpenML. CoRR abs/1911.02490 (2019) - 2018
- [j5]Salisu Mamman Abdulrahman
, Pavel Brazdil
, Jan N. van Rijn, Joaquin Vanschoren
:
Speeding up algorithm selection using average ranking and active testing by introducing runtime. Mach. Learn. 107(1): 79-108 (2018) - [j4]Jan N. van Rijn, Geoffrey Holmes, Bernhard Pfahringer, Joaquin Vanschoren
:
The online performance estimation framework: heterogeneous ensemble learning for data streams. Mach. Learn. 107(1): 149-176 (2018) - [c13]Jan N. van Rijn, Frank W. Takes
, Jonathan K. Vis
:
Computing and Predicting Winning Hands in the Trick-Taking Game of Klaverjas. BNCAI 2018: 106-120 - [c12]Benjamin Strang, Peter van der Putten, Jan N. van Rijn, Frank Hutter:
Don't Rule Out Simple Models Prematurely: A Large Scale Benchmark Comparing Linear and Non-linear Classifiers in OpenML. IDA 2018: 303-315 - [c11]Jan N. van Rijn, Frank Hutter:
Hyperparameter Importance Across Datasets. KDD 2018: 2367-2376 - [i9]Marius Lindauer, Jan N. van Rijn, Lars Kotthoff:
The Algorithm Selection Competition Series 2015-17. CoRR abs/1805.01214 (2018) - [i8]Florian Pfisterer, Jan N. van Rijn, Philipp Probst, Andreas C. Müller, Bernd Bischl:
Learning Multiple Defaults for Machine Learning Algorithms. CoRR abs/1811.09409 (2018) - 2017
- [c10]Jan N. van Rijn, Frank Hutter:
An Empirical Study of Hyperparameter Importance Across Datasets. AutoML@PKDD/ECML 2017: 91-98 - [i7]Marius Lindauer
, Jan N. van Rijn, Lars Kotthoff:
Open Algorithm Selection Challenge 2017: Setup and Scenarios. OASC 2017: 1-7 - [i6]Bernd Bischl, Giuseppe Casalicchio, Matthias Feurer, Frank Hutter, Michel Lang
, Rafael Gomes Mantovani
, Jan N. van Rijn, Joaquin Vanschoren:
OpenML Benchmarking Suites and the OpenML100. CoRR abs/1708.03731 (2017) - [i5]Jan N. van Rijn, Frank Hutter:
Hyperparameter Importance Across Datasets. CoRR abs/1710.04725 (2017) - 2016
- [c9]Martijn J. Post, Peter van der Putten, Jan N. van Rijn:
Does Feature Selection Improve Classification? A Large Scale Experiment in OpenML. IDA 2016: 158-170 - [i4]Hendrik Jan Hoogeboom, Walter A. Kosters, Jan N. van Rijn, Jonathan K. Vis:
Acyclic Constraint Logic and Games. CoRR abs/1604.05487 (2016) - [i3]Jan N. van Rijn, Jonathan K. Vis:
Endgame Analysis of Dou Shou Qi. CoRR abs/1604.07312 (2016) - [i2]Jan N. van Rijn, Frank W. Takes, Jonathan K. Vis:
The Complexity of Rummikub Problems. CoRR abs/1604.07553 (2016) - 2015
- [c8]Jan N. van Rijn, Geoffrey Holmes, Bernhard Pfahringer, Joaquin Vanschoren
:
Having a Blast: Meta-Learning and Heterogeneous Ensembles for Data Streams. ICDM 2015: 1003-1008 - [c7]Jan N. van Rijn, Salisu Mamman Abdulrahman
, Pavel Brazdil
, Joaquin Vanschoren
:
Fast Algorithm Selection Using Learning Curves. IDA 2015: 298-309 - [c6]Joaquin Vanschoren, Jan N. van Rijn, Bernd Bischl:
Taking machine learning research online with OpenML. BigMine 2015: 1-4 - [c5]Salisu Mamman Abdulrahman, Pavel Brazdil, Jan N. van Rijn, Joaquin Vanschoren:
Algorithm Selection via Meta-learning and Sample-based Active Testing. MetaSel@PKDD/ECML 2015: 55-66 - [c4]Jan N. van Rijn, Joaquin Vanschoren:
Sharing RapidMiner Workflows and Experiments with OpenML. MetaSel@PKDD/ECML 2015: 93-103 - 2014
- [j3]Hendrik Jan Hoogeboom
, Walter A. Kosters, Jan N. van Rijn, Jonathan K. Vis
:
Acyclic Constraint Logic and Games. J. Int. Comput. Games Assoc. 37(1): 3-16 (2014) - [j2]Jan N. van Rijn, Jonathan K. Vis
:
Endgame Analysis of DOU SHOU QI. J. Int. Comput. Games Assoc. 37(2): 120-124 (2014) - [c3]Jan N. van Rijn, Geoffrey Holmes, Bernhard Pfahringer, Joaquin Vanschoren
:
Algorithm Selection on Data Streams. Discovery Science 2014: 325-336 - [c2]Jan N. van Rijn, Geoffrey Holmes, Bernhard Pfahringer, Joaquin Vanschoren:
Towards Meta-learning over Data Streams. MetaSel@ECAI 2014: 37-38 - [i1]Joaquin Vanschoren, Jan N. van Rijn, Bernd Bischl, Luís Torgo
:
OpenML: networked science in machine learning. CoRR abs/1407.7722 (2014) - 2013
- [j1]Joaquin Vanschoren, Jan N. van Rijn, Bernd Bischl, Luís Torgo
:
OpenML: networked science in machine learning. SIGKDD Explor. 15(2): 49-60 (2013) - [c1]Jan N. van Rijn, Bernd Bischl, Luís Torgo
, Bo Gao, Venkatesh Umaashankar, Simon Fischer, Patrick Winter, Bernd Wiswedel, Michael R. Berthold, Joaquin Vanschoren
:
OpenML: A Collaborative Science Platform. ECML/PKDD (3) 2013: 645-649
Coauthor Index

manage site settings
To protect your privacy, all features that rely on external API calls from your browser are turned off by default. You need to opt-in for them to become active. All settings here will be stored as cookies with your web browser. For more information see our F.A.Q.
Unpaywalled article links
Add open access links from to the list of external document links (if available).
Privacy notice: By enabling the option above, your browser will contact the API of unpaywall.org to load hyperlinks to open access articles. Although we do not have any reason to believe that your call will be tracked, we do not have any control over how the remote server uses your data. So please proceed with care and consider checking the Unpaywall privacy policy.
Archived links via Wayback Machine
For web page which are no longer available, try to retrieve content from the of the Internet Archive (if available).
Privacy notice: By enabling the option above, your browser will contact the API of archive.org to check for archived content of web pages that are no longer available. Although we do not have any reason to believe that your call will be tracked, we do not have any control over how the remote server uses your data. So please proceed with care and consider checking the Internet Archive privacy policy.
Reference lists
Add a list of references from ,
, and
to record detail pages.
load references from crossref.org and opencitations.net
Privacy notice: By enabling the option above, your browser will contact the APIs of crossref.org, opencitations.net, and semanticscholar.org to load article reference information. Although we do not have any reason to believe that your call will be tracked, we do not have any control over how the remote server uses your data. So please proceed with care and consider checking the Crossref privacy policy and the OpenCitations privacy policy, as well as the AI2 Privacy Policy covering Semantic Scholar.
Citation data
Add a list of citing articles from and
to record detail pages.
load citations from opencitations.net
Privacy notice: By enabling the option above, your browser will contact the API of opencitations.net and semanticscholar.org to load citation information. Although we do not have any reason to believe that your call will be tracked, we do not have any control over how the remote server uses your data. So please proceed with care and consider checking the OpenCitations privacy policy as well as the AI2 Privacy Policy covering Semantic Scholar.
OpenAlex data
Load additional information about publications from .
Privacy notice: By enabling the option above, your browser will contact the API of openalex.org to load additional information. Although we do not have any reason to believe that your call will be tracked, we do not have any control over how the remote server uses your data. So please proceed with care and consider checking the information given by OpenAlex.
last updated on 2024-11-06 20:26 CET by the dblp team
all metadata released as open data under CC0 1.0 license
see also: Terms of Use | Privacy Policy | Imprint