"If we knew what it was we were doing, it would not be called research, would it?" - Albert Einstein

Postdoctoral Research Fellow
Johns Hopkins University
Previous Affiliations
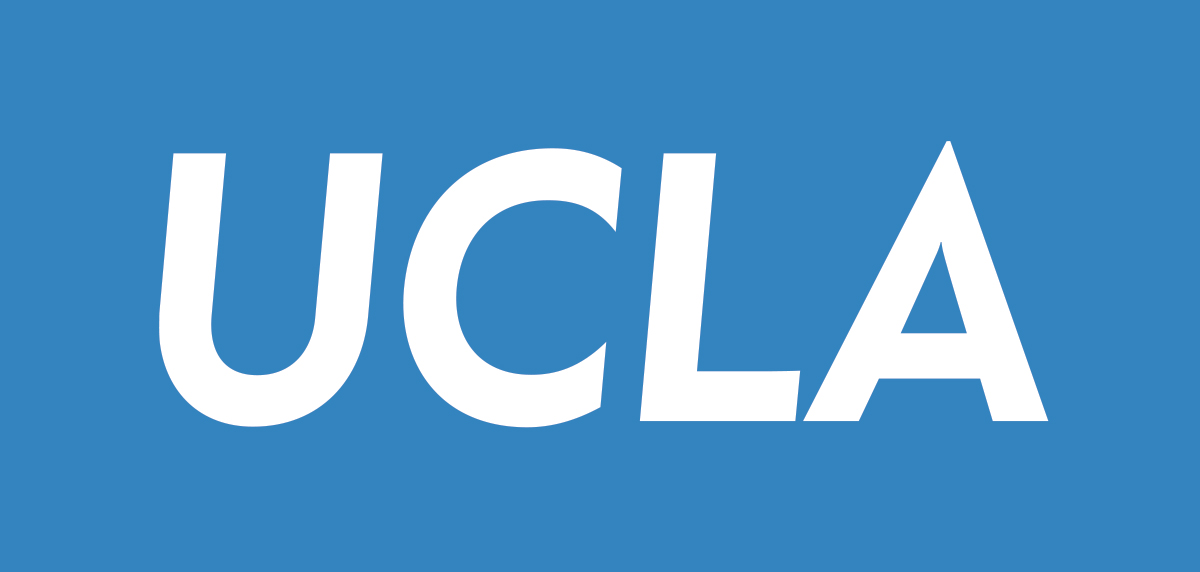


Postdoctoral Research Fellow Visiting Researcher PhD Student
(October 2014 - January 2016) (October 2013 - August 2014) (August 2009 - August 2013)
Brief Bio
Vittal Premachandran is a Postdoctoral Researcher at the Johns Hopkins University. He obtained the B.E. degree in Computer Science from Visveswaraya Technological University, India, in 2009, graduating with a First Class with Distinction. He received his Ph.D degree, also in Computer Science, from Nanyang Technological University, Singapore, in 2014. He has previously worked as a Postdoctoral Research Fellow at University of California, Los Angeles, and a visiting researcher at National University of Singapore.
His research interests span computer vision and machine learning, with a focus on semantic analysis of scenes. His work has received the Best Student Paper award at the IEEE International Conference on Image Processing (ICIP) in 2013.
Talks and Lectures
- Tutorial on Efficient Inference in Gaussian CRFs @ CCVL, Johns Hopkins Unviersity, August, 2016.
- Fully Convolutional Neural Networks - Guest Lecture for 'Deep Learning for Image Understanding' @ Johns Hopkins Unviersity, March, 2016.
- Introduction to Deep Learning and using Caffe - Guest Lecture for 'Deep Learning for Image Understanding' @ Johns Hopkins Unviersity, March, 2016.
- Reinforcement Learning - Guest Lecture for Stat 271: Probabilistic Models of the Visual Cortex, UCLA, November, 2015.
- Introduction to Deep Networks - Guest Lecture for Stat 271: Probabilistic Models of the Visual Cortex, UCLA, November, 2015.
- Introduction to Vision - Guest Lecture for Stat 271: Probabilistic Models of the Visual Cortex, UCLA, September, 2015.
- Introduction to Deep Networks - Stat 161/261: Introduction to Machine Learning, UCLA, June 2015.
- K-Means, GMM and EM - Stat 161/261: Introduction to Machine Learning, UCLA, May 2015.
- Decision Trees and Random Forest - Stat 161/261: Introduction to Machine Learning, UCLA, May 2015.
- Decision Trees - Stat 161/261: Introduction to Machine Learning, UCLA, May 2015.
- Soft Margin Support Vector Machines - Stat 161/261: Introduction to Machine Learning, UCLA, April 2015.
- Support Vector Machines - Stat 161/261: Introduction to Machine Learning, UCLA, April 2015.
- Perceptrons and Kernels - Stat 161/261: Introduction to Machine Learning, UCLA, April 2015.
- Introduction to Machine Learning - Stat 161/261: Introduction to Machine Learning, UCLA, March 2015.
- Hedging on diversity and making better predictions using EMBR - Guest Lecture for Stat 238: Vision as Bayesian Inference course @ UCLA, November, 2014.
- Empirical Minimum Bayes Risk Prediction @ CCVL, UCLA, October, 2014
- Exploiting Shape Properties for Improved Retrieval, Discrimination and Recognition @ Amazon, Seattle, May 2014.
- Exploiting Shape Properties for Improved Retrieval, Disctimination and Recognition @ NTU, Singapore, February, 2014
Publications
Pre-prints

Daniil Pakhomov, Vittal Premachandran, Max Allan, Mahdi Azizian, Nassir Navab [arXiv]
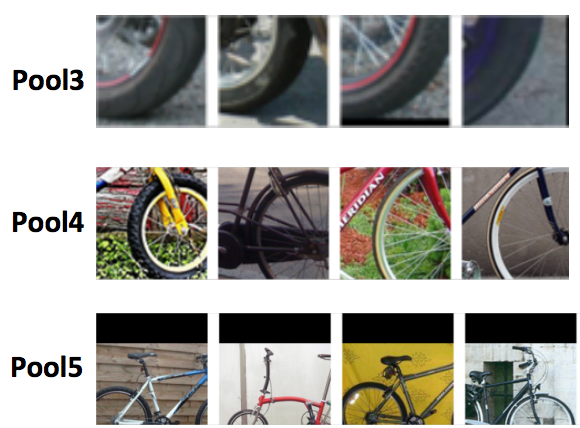
Jianyu Wang, Zhishuai Zhang, Cihang Xie, Vittal Premachandran, Alan Yuille [arXiv]
Journal Publications

Vittal Premachandran, Daniel Tarlow, Alan L. Yuille, Dhruv Batra
Transactions on Pattern Analysis and Machine Intelligence (TPAMI), 2016,

Vittal Premachandran and Ramakrishna Kakarala
Pattern Recognition, 2013,
[ArXiv]
Conference Publications
-
PASCAL Boundaries: A Semantic Boundary Dataset with A Deep Semantic Boundary Detector
Vittal Premachandran, Boyan Bonev, Xiaochen Lian and Alan L. Yuille
Winter Conference on Applications of Computer Vision (WACV), 2017, Santa Rosa, California. [PDF]
-
Empirical Minimum Bayes Risk Prediction:
How to extract an extra few % performance from vision models with just three more parameters,
Vittal Premachandran, Daniel Tarlow and Dhruv Batra
IEEE Conference on Computer Vision and Pattern Recognition (CVPR), 2014, Columbus, Ohio. [Project Page]
-
What parts of a shape are discriminative?,[Best Student Paper Award!]
Vittal Premachandran and Ramakrishna Kakarala
IEEE International Conference on Image Processing (ICIP), 2013. [PDF] [Presentation Slides]
-
Consensus of k-NNs for Robust Neighborhood Selection on Graph-Based Manifolds,
Vittal Premachandran and Ramakrishna Kakarala
IEEE Conference on Computer Vision and Pattern Recognition (CVPR), 2013, Portland, Oregon. [PDF]
-
Three-dimensional bilateral symmetry plane estimation in the phase domain,
Ramakrishna Kakarala, Prabhu Kaliamoorthi*, and Vittal Premachandran*
IEEE Conference on Computer Vision and Pattern Recognition (CVPR), 2013, Portland, Oregon. [PDF]
* indicates equal contribution
-
Can relative skill be determined from a photographic portfolio?,
Abhishek Agrawal, Vittal Premachandran, Rajesh Somavarapu, Ramakrishna Kakarala
SPIE Conference on Electronic Imaging, 2013. [PDF] [SPIE]
-
Dense sampling of shape interiors for improved representation,
Vittal Premachandran and Ramakrishna Kakarala,
SPIE Conference on Electronic Imaging, 2013. [PDF] [SPIE]
-
Improving Shape Context Using Geodesic Information And Refection Invariance,
Vittal Premachandran and Ramakrishna Kakarala,
SPIE Conference on Electronic Imaging, 2013. [PDF] [SPIE]
-
Comparing automated and human ratings of photographic aesthetics,
Ramakrishna Kakarala, Todd Sachs, and Vittal Premachandran,
2011 Color Imaging Conference, 7-11 Nov, San Jose CA. [PDF] [Web Link]
"Measuring the Effectiveness of Bad Pixel Detection Algorithms Using the ROC Curve",
Vittal Premachandran and Ramakrishna Kakarala,
IEEE Transactions on Consumer Electronics, November 2010. [PDF] [IEEE Link]
Ph.D Thesis
-
"Exploiting Shape Properties for Improved Retrieval, Discrimination and Recognition"
Vittal Premachandran
February 2014. [PDF ~12.5MB]
Defense Slides: [PPTx ~14MB] [PDF ~5MB]
Awards
- Best Student Paper Award at the IEEE International Conference on Image Processing (ICIP), 2013, Melbourne, Australia. [NTU-SCE Website]
- Best Presentation Award at AOTULE 2011, Beijing. [NTU-SCE Website]